AI’s Next Chapter Requires Human Expertise
Annie K. Lamar / Apr 25, 2025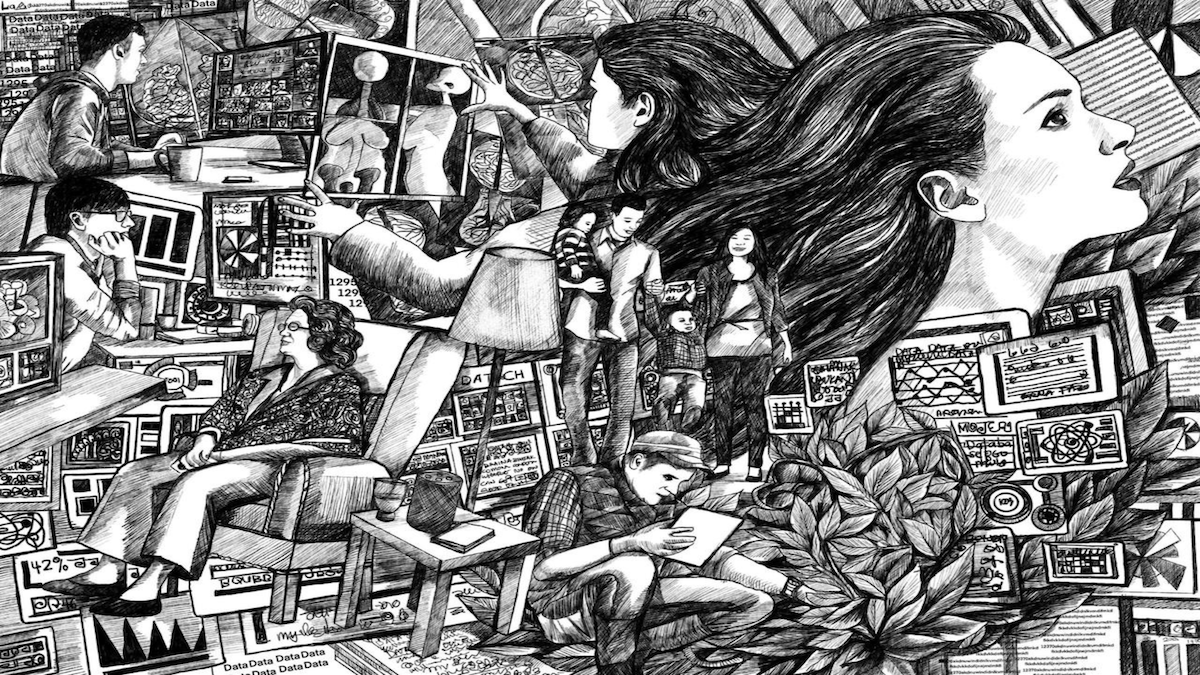
Ariyana Ahmad & The Bigger Picture / Better Images of AI / Licensed by CC-BY 4.0
Tech layoffs continued to surge last month. Many companies, including Microsoft, Oracle, and Amazon, have cited the expansion of AI into software development as a driving force behind these layoffs. In an employment ecosystem where technical skills no longer guarantee jobs, there is a push towards training and education in creative fields—jobs that many hope will be ‘AI-proof’ in the future.
But this narrative gets it backward. The future of AI doesn’t just require technical skills; it demands deep human expertise. Linguists, historians, sociologists, and artists will be critical to building AI systems that are nuanced, ethical, and socially informed.
Yet just as this expertise becomes more essential, policymakers are defunding it. States are gutting humanities programs. Federal cuts to research funding are hitting the sciences and threaten to devastate the humanities and social sciences. These aren’t just cultural losses. They’re strategic errors.
The next generation of artificial intelligence will not only rely on massive datasets to generate text or simulate knowledge. It will also rely on small, tailored, domain-specific data: training sets designed not to generalize, but to capture nuance.
Nearly two years ago, Sam Altman, the CEO of OpenAI, told an audience at MIT that we were at the “end of an era” when it came to giant data. Altman’s statement has done little to curb enthusiasm for large language models (LLMs), both in the start-up sphere and broader society, but he wasn’t wrong. Big data has solved big problems, but it’s also created massive ones too: surging carbon emissions, escalating misinformation, and new waves of cybersecurity threats and scams.
An alternative is small data, and the small language models we can train with offer us unique opportunities to solve complex problems in more sustainable ways. However, this depends on the ability to curate data with precision and to evaluate output with depth, which requires disciplinary expertise.
Tiny models, or “low-resource” models, are trained on datasets far smaller than those used by systems like ChatGPT. The definition of "tiny data" varies depending on the field and the type of measurement, but it generally refers to datasets around 10 GB or smaller—essentially, anything that can be processed on a single machine, such as a personal laptop. In natural language processing, low-resource models are often trained on less than 1 gigabyte of data (or less than approximately one million sentences). In contrast, large language models like ChatGPT-3 were trained on data sets such as CommonCrawl, constituting 45 terabytes of text.
Compared to larger models, tiny models can often generate responses more quickly, use less energy, and—crucially—perform well in specific and narrowly defined contexts like ecological modeling, medical diagnostics, and geographically-limited studies. Tiny data models help us to perform tasks ranging from accurately measuring the presence of rare species, detecting different types of cancers and red blood cell abnormalities, and predicting where parking will be available.
What unites these examples is the need for precision. Medical diagnostics, for instance, must go beyond general labels—a vague alert like "you have cancer" is far less useful than identifying a specific type that informs treatment. Similarly, predicting parking availability requires detailed knowledge of a city’s infrastructure and the behaviors of its residents. Further, since location-specific data is often not joinable with other datasets for structural, logistical, or political reasons, tiny data models are often the only viable choice for urban planners and local officials seeking to apply machine learning to local challenges.
A particularly compelling case is air quality forecasting. Machine learning models for this task typically rely on large datasets collected from major cities. As a result, smaller cities and less affluent countries—often those most affected by air pollution—are excluded. In such cases, tiny data is not just an alternative; it is the only viable option. Building effective models under these constraints requires more than just clever algorithms. It demands a deep understanding of the local context, including economics, environmental patterns, and everyday human activity. When combined with even limited data, this collaborative model can produce models that are as reliable as more generic, large-scale systems.
The great advantage of a large language model is its ability to generalize. Generalization is the key to this AI model’s ability to produce ‘original’ generated text. However, in today’s world, it is also one of its greatest weaknesses., Despite advanced generalization capabilities and vast training datasets, LLMs may be unable to help us address many of the complex and nuanced challenges society will face in the decades ahead.
The solutions to these challenges will require tiny data models built not to generalize everything, but to understand something deeply. Small data offers us a path forward that prioritizes accuracy, efficiency, and human expertise. But this shift doesn’t just demand better code; it demands better collaboration. Medical diagnostics need oncologists. Climate models and planning benefit from the expertise and input from archaeologists and environmental historians. Preserving endangered languages requires linguists.
Thus, the future of AI depends not just on engineering breakthroughs but on a continued investment in the humanities and social sciences. Yet at this crucial moment, public policy is moving in the opposite direction. Across the United States, state governments are defunding humanities and social science programs and stripping support for disciplines deemed ‘non-essential.’ Federally, proposed cuts to research budgets threaten not only scientific innovation but also the already fragile infrastructure of humanities scholarship.
Proposed reductions to NSF, NIH, and NEH budgets will erode support for precisely the kinds of disciplinary expertise small models depend on. If we want models that reflect the richness of human experience, we must invest in the fields that study it. That means restoring federal and state support for humanities and social science research and protecting educational programs that prepare students to work at the intersection of technology and culture. The future of AI is not just about engineering: it’s about understanding the world we’re engineering for.
Authors
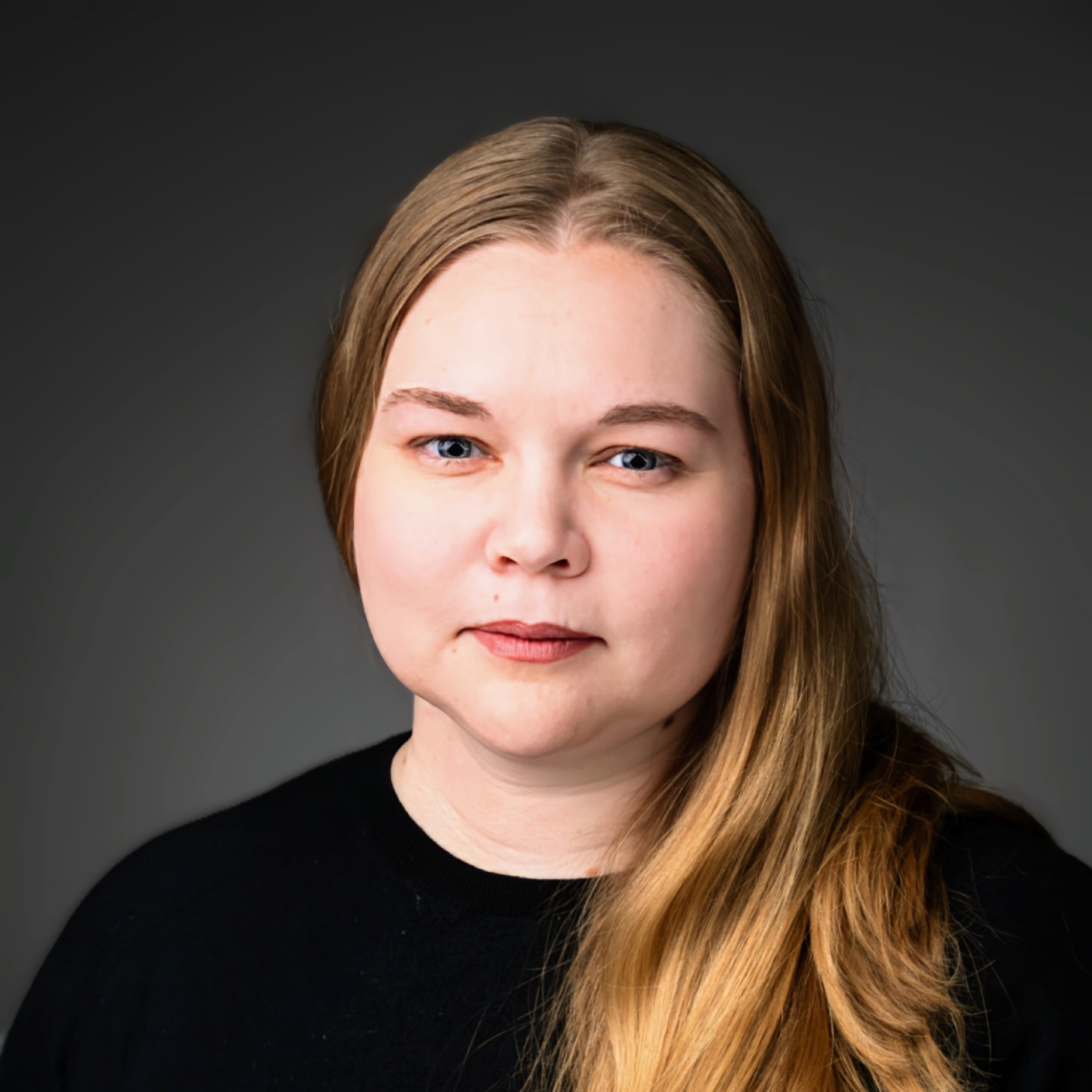