Before AI Agents Act, We Need Answers
Ruchika Joshi / Apr 17, 2025AI agents are being deployed faster than developers can answer critical questions about them. That needs to change, writes Ruchika Joshi, AI Governance Fellow at the Center for Democracy & Technology.
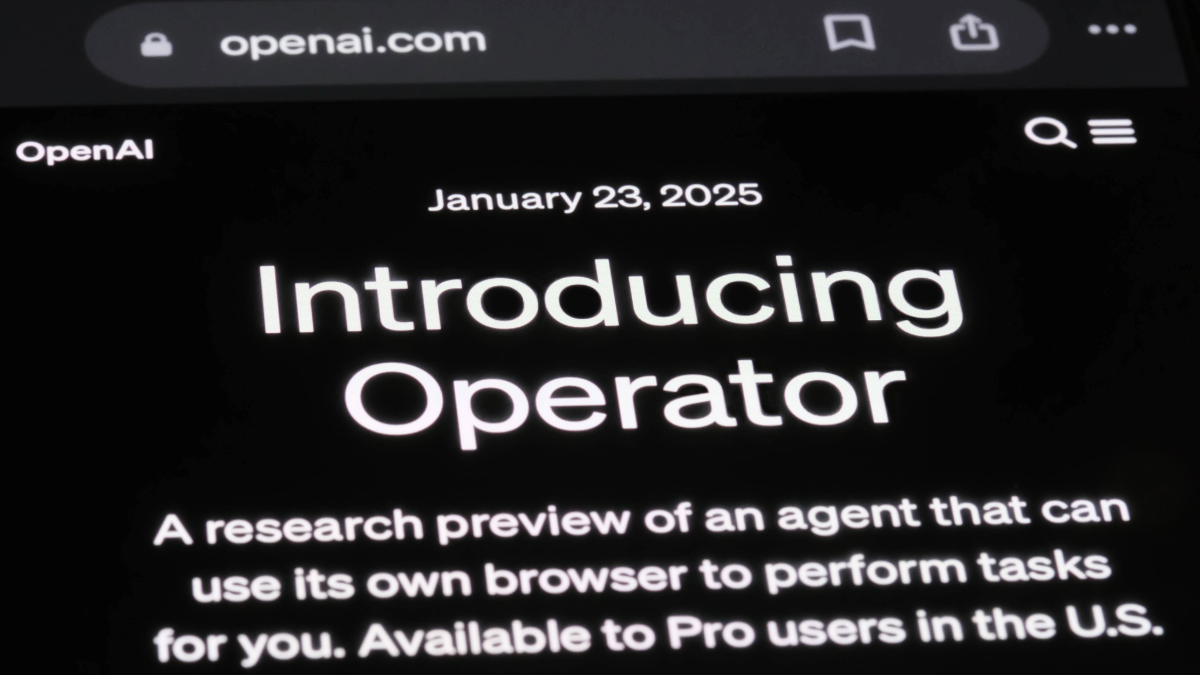
The landing page for OpenAI's Operator service. Shutterstock
Tech companies are betting big on AI agents. From sweeping organizational overhauls to CEOs claiming agents will ‘join the workforce’ and power a multi-trillion-dollar industry, the race to match hype is on.
While the boundaries of what qualifies as an ‘AI agent’ remain fuzzy, the term is commonly used to describe AI systems designed to plan and execute tasks on behalf of users with increasing autonomy. Unlike AI-powered systems like chatbots or recommendation engines, which can generate responses or make suggestions to assist users in making decisions, AI agents are envisioned to execute those decisions by directly interacting with external websites or tools via APIs.
Where an AI chatbot might have previously suggested flight routes to a given destination, AI agents are now being designed to find which flight is cheapest, book the ticket, fill out the user’s passport information, and email the boarding pass. Building on that idea, early demonstrations of agent use include operating a computer for grocery shopping, automating HR approvals, or managing legal compliance tasks.
Yet current AI agents have been quick to break, indicating that reliable task execution remains an elusive goal. This is unsurprising, since AI agents rely on the same foundation models as non-agentic AI and so are prone to familiar challenges of bias, hallucination, brittle reasoning, and limited real-world grounding. Non-agentic AI systems have already been shown to make expensive mistakes, exhibit biased decision making, and mislead users about their ‘thinking’. Enabling such systems to now act on behalf of users will only raise the stakes of these failures.
As companies race to build and deploy AI agents to act with less supervision than earlier systems, what is keeping these agents from harming people?
The unsettling answer is that no one really knows, and the documentation that the agent developers provide doesn’t add much clarity. For example, while system or model cards released by OpenAI and Anthropic offer some details on agent capabilities and safety testing, they also include vague assurances on risk mitigation efforts without providing supporting evidence. Others have released no documentation at all or only done so after considerable delay.
However, the public needs far more information to meaningfully evaluate whether, when, and how to use AI agents, and what safeguards will be needed to manage agent risks. To that end, six questions stand out as critical for developers to answer:
1. How are developers preventing agents from being hacked or used for hacking?
Since AI agents are being designed to interact with third-party systems, access user data, and even control devices, attack surfaces for hackers are exponentially increasing. As a result, the consequences of prompt injection—attacks that manipulate system inputs to override intended agent behavior, bypass safeguards, or trigger unauthorized actions—become more serious.
AI agent developers appear to recognize this threat, with some reporting statistics on the efficacy of efforts to detect and block such attacks, while others release “beta” models with little detail on prompt injection mitigations and just a warning to avoid sensitive tasks. But such metrics often lack critical context: What kinds of attacks are reliably blocked, and how do developers anticipate defenses evolving as adversaries adapt? How comparable are security mitigations and evaluations across companies? And in high-risk domains like finance, healthcare, or cybersecurity, are the current failure rates even acceptable? For example, Anthropic reports that while testing its experimental Computer Use agent, it was able to block 88% of prompt injection attempts, but that still means more than one in 10 attacks succeeded.
Beyond prompt injection threats, there’s also the broader risk of deliberate misuse of agents by users themselves, especially in cybersecurity contexts, which raises further questions about how developers are safeguarding against their agents wreaking cyber-havoc on the internet.
2. How much do agents know about users—and when and with whom can they share that information?
The more AI agents know and remember about users, the more personalized their assistance can presumably be. But the information agents can access or hold also makes people more vulnerable to data leaks, adversarial attacks, or product decisions that may trade away privacy for convenience. Unlike social media platforms or traditional apps that may store data for a defined set of functionalities or contexts, agents are being explicitly designed to operate across platforms, tasks, and time, which could incentivize even more personal data collection.
For example, a scheduling agent that integrates with a user’s email, calendar, and messaging apps might not only access sensitive data like calendar event details or login credentials, but also infer more intimate, multi-dimensional information spanning the user’s medical conditions or financial activity.
Currently, users have some control over what agents remember, store, or share—often similar to controls available for non-agentic product offerings. For instance, OpenAI allows one-click deletion of browsing data, chats, and login sessions.
But when users don’t choose to delete their data, what information do agents retain across user sessions, and how is that leveraged? Can that data, for example, be used to infer user traits like political views or mental health? And when agents interact with other services, what data sharing occurs? Before users entrust their data to AI agents, these questions need answers.
3. What control do users have over what agents are doing?
As AI agents become capable of executing more complex tasks with decreasing supervision, they raise urgent questions of human oversight and control. Too little human involvement, and agents risk taking unintended or harmful actions. Too much friction—like needing multiple human approvals or constant monitoring at every single step—erodes the primary value proposition of agents
So, how do developers ensure that agents accurately report to users what they plan to do, what they’ve done, and why? How are thresholds around which agent actions require user approval defined? And how reliable are the systems enforcing those thresholds?
Early reports show that AI agents still have lots to learn about when they need to stop and get user approval. For example, OpenAI’s computer use agent, Operator, reportedly purchased a dozen eggs online for a total cost of $31, when all the user had asked it to do was to locate a nearby grocery store with the cheapest eggs. Instead, the agent leapfrogged to making the purchase without approval and even misreported the final cost, despite OpenAI’s assurances that Operator requires user confirmation and automatically blocks high-risk tasks.
Without adequate opportunity for users to assess, pause, or override agent actions, agent failures are poised to make even costlier errors like filling out the wrong medical form, prematurely sending a sensitive email, or selling a stock without authorization.
4. What shared technical or legal infrastructure must be built to govern agents?
Since AI agents can operate at scale to browse the web, submit forms, make purchases, or query APIs across systems within a matter of seconds, their collective impact on the digital ecosystem demands serious attention. For instance, currently, there is no standard way to flag AI-generated internet traffic as distinct from humans. Without clear agent identification, agent activity can't be reliably tracked or audited, even when it overwhelms websites or facilitates manipulation and fraud at scale.
Addressing these challenges goes beyond what any single AI developer can do, and what interoperability-related coordination efforts—like OpenAI’s adoption of Anthropic’s Model Context Protocol—can achieve. In case of agent visibility, for instance, it involves answering broader questions like: Should agent interactions be labeled? To what extent should users be notified when they're engaging with an AI agent, not a person? Could such identifiers be enforced technically or legally without undermining privacy, anonymity, or free expression?
Questions about agent visibility point to a larger set of governance challenges—such as monitoring real-world harms, setting safety standards for model access and deployment, and enabling effective public oversight mechanisms—that will require revisiting the legal and technical infrastructure needed to govern AI agents across platforms, jurisdictions, and stakeholder groups.
5. What strategies are needed to mitigate psychological, social, and political risks from designing increasingly human-like agents?
Tens of millions of users engage daily with personalized AI companions, often for over an hour a day. At the same time, recent reports of people forming strong emotional bonds with AI chatbots raise concerns about the implications of these systems for their users, particularly those who are young, isolated, or emotionally vulnerable. Indeed, an OpenAI and MIT study reports that extended use of chatbots by users who experience greater loneliness correlates with negative impacts on their well-being.
As AI systems increasingly mimic human mannerisms and implement tasks on their behalf, users may trust them more, disclose more sensitive information, and form emotional attachments. Such interactions can leave users vulnerable to emotional manipulation by AI systems, potentially fueling misinformation, impersonation scams, or unhealthy relational patterns.
These dynamics raise important questions: What design choices are being made to encourage—or prevent—users from building emotional relationships with agents? Are users clearly informed when they’re speaking to an AI system, and are those signals sufficient against human tendency to anthropomorphize agents anyway? What controls do users have to set emotional boundaries or adjust the level of human-likeness an agent demonstrates? Currently, developers eager to capitalize on user attention and emotional connection with human-like agents share little on how these concerns are informing their design choices.
6. What responsibilities do developers have when agents cause harm?
Most AI agent developers disclaim responsibility upfront by deploying AI products “as-is” in their terms of use or software licenses. An emerging trend of concern is companies releasing AI agents as ‘research previews’ or ‘prototypes’, even as they incorporate advanced capabilities into premium-tier product offerings, seemingly allowing companies to benefit from early deployment while avoiding accountability if things go wrong.
Meanwhile, the broader regulatory landscape is moving away from closing gaps in liability regimes as related to AI. For instance, the EU recently dropped efforts to advance the AI Liability Directive, which would have allowed consumers to sue for damages caused by the fault or omission of AI developers, providers, or users.
In a situation where liability remains undefined, who will be responsible when an agent misbehaves and causes financial loss, clinical misdiagnosis, or emotional harm? In which contexts should developers, deployers, or other actors along the AI supply chain be expected to accept responsibility? And if they won’t do so voluntarily, what legal, regulatory, or societal mechanisms are needed to change that?
Past experience of consumer technology suggests that user attention, trust, and engagement are primarily monetized through behavioral advertising. As developers explore business models for AI agents, what duty of care should they have to protect users from manipulation, misuse, and harm? A world in which developers seek to capture the economic upside of agent deployment while offloading all risks to the public seems neither just nor sustainable.
Across these six areas of concern, one thing is clear: AI agents are being deployed faster than developers can answer critical questions about them. While some experts have urged halting highly autonomous agents until society catches up, current market dynamics appear to make that unlikely. With billions of dollars riding on agents, most companies are accelerating agent deployment by emphasizing convenience and sidelining critical risks. Initial efforts by some developers to publish a few safety metrics, offer basic user controls, and acknowledge real-world limitations are a welcome start. But they remain insufficient for addressing emerging risks to human rights, safety, and public trust.
And yet, a narrow window still exists to get ahead of risks before AI agents are adopted widely. Unlike the rollout of social media or the early internet—where individual and societal harms were acknowledged after they became entrenched—developers now have a chance to build safer, more accountable systems from the start. Admittedly, the questions they face are thorny and involve complex tradeoffs, answering which will require collaboration with civil society, academics, and policymakers, even as developers remain ultimately responsible for the products they build. Developers must therefore shift away from releasing powerful agents as ‘research prototypes’ with opaque safety assurances, towards addressing these questions head-on—inviting meaningful input from public interest experts and others who stand ready to help.
Authors
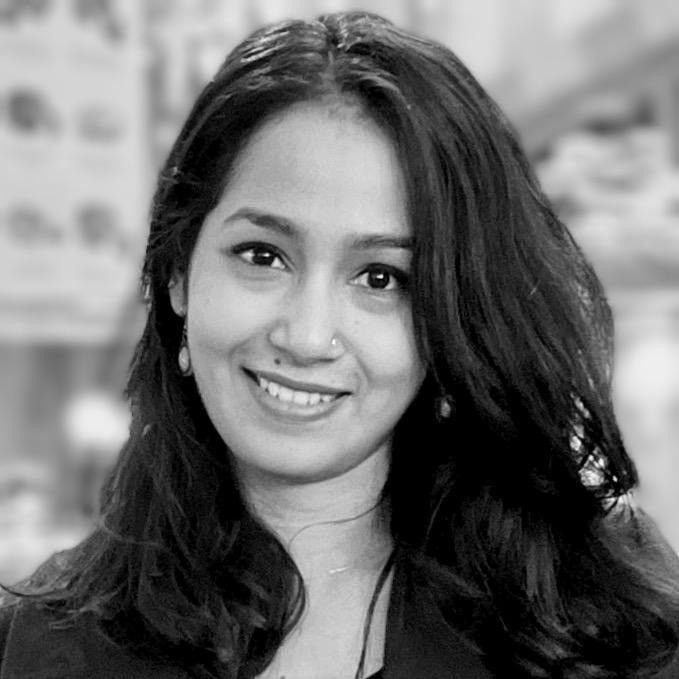