Beyond Safe Models: Why AI Governance Must Tackle Unsafe Ecosystems
Ludovic Terren / May 1, 2025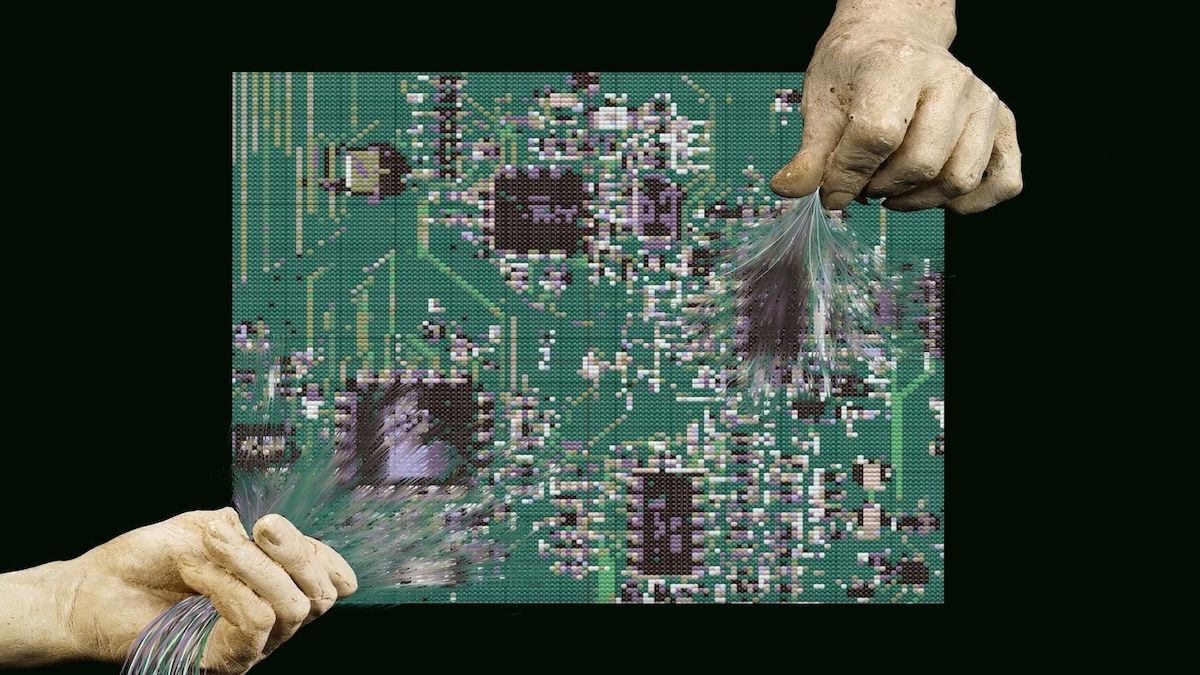
Hanna Barakat + AIxDESIGN & Archival Images of AI / Better Images of AI / Woven Circuit / CC-BY 4.0
Just as a safe tool can become dangerous in the wrong hands, a well-aligned AI model can still cause harm when deployed in misaligned, opaque, or unprepared systems. While global attention to AI governance is growing, much of the focus remains on model-level safety— ensuring that the tool operates as intended. However, some of the most immediate risks arise not from AI itself, but from how it functions within its context—when embedded in institutions with conflicting incentives, weak oversight, or inadequate safeguards. The EU AI Act lays important groundwork by introducing procedural and technical obligations and restricting specific high-risk applications. However, like many current efforts, it primarily focuses on the properties of AI models, rather than the environments in which they are deployed. To govern AI effectively, we need to broaden our focus from safe models to safe ecosystems.
These deployment risks aren’t theoretical. Recommender systems on social media platforms, for example, are technically sound—built to optimize user engagement. But in doing so, they have been found to amplify polarization and misinformation. The harm isn’t in the algorithm’s logic, but in the platform’s incentive to prioritize attention at all costs.
Similarly, AI tools used in hiring have shown racial and gender discrimination despite meeting technical standards. One system ranked candidates lower for having attended women’s colleges—not due to a technical failure, but because it inherited bias from past hiring decisions and was deployed without meaningful oversight or contestability.
In both cases, the underlying models may meet technical benchmarks. But deployed in high-stakes, opaque environments with misaligned goals, they can produce outcomes that are neither fair nor safe.
From Safe Models to Safe Ecosystems
Despite the evident risks of unsafe deployment ecosystems, the prevailing approach to AI governance still heavily emphasizes pre-deployment interventions—such as alignment research, interpretability tools, and red teaming—aimed at ensuring that the model itself is technically sound. Governance initiatives like the EU AI Act, while vital, primarily place obligations on providers and developers to ensure compliance through documentation, transparency, and risk management plans. However, the governance of what happens after deployment when these models enter institutions with their own incentives, infrastructures, and oversight receives comparatively less attention. For example, while the EU AI Act introduces post-market monitoring and deployer obligations for high-risk AI systems, these provisions remain limited in scope. Monitoring primarily focuses on technical compliance and performance, with little attention to broader institutional, social, or systemic impacts. Deployer responsibilities are only weakly integrated into ongoing risk governance and focus primarily on procedural requirements—such as record-keeping and ensuring human oversight—rather than assessing whether the deploying institution has the capacity, incentives, or safeguards to use the system responsibly. As a result, there is limited assurance that AI systems will be embedded in environments capable of managing their evolving real-world risks.
Yet, as seen with biased hiring AI and polarizing recommender systems, it is in the deployment ecosystem that much of the risk materializes. This ecosystem is a complex, interconnected environment involving the institutions that deploy the AI, the objectives they optimize for (such as efficiency, engagement, or profit), the technical and organizational infrastructure supporting its use, and the legal, regulatory, and social contexts in which it operates. Like any ecosystem, its elements are interdependent: choices made in one area shape and are shaped by others. If, for example, deployers lack adequate training because incentive structures prioritise rapid deployment over thorough preparation, or if the public lacks recourse to challenge automated decisions because opaque design choices and institutional fragmentation obscure lines of accountability, then technical safety alone cannot prevent downstream harm.
AI governance must therefore ask: Where is this system being used, by whom, for what purpose—and with what kind of oversight? We must move beyond pre-deployment model checks and adopt a robust framework that places the safety of the deployment ecosystem at the centre of risk evaluation.
A Context-Aware Risk Assessment Framework
To help shift the focus beyond model-centric governance, I highlight four critical features of deployment ecosystems that can amplify or mitigate the risks of AI in practice:
- Incentive alignment: Governance must consider whether institutions deploying AI systems prioritize the public good over short-term objectives, such as profit, engagement, or cost-cutting. Even a technically sound AI can cause harm when used in a setting where incentives reward manipulative or extractive outcomes. While the EU AI Act regulates certain use cases and assigns risk levels, it does not systematically evaluate the motivations or optimization goals of deploying organizations—leaving a critical layer of real-world risk unexamined.
- Contextual readiness: Not all deployment ecosystems are equally equipped to manage the risks of AI. Underlying factors such as legal safeguards (e.g., enforceable rights to contest decisions), technical infrastructure (e.g., secure data systems), institutional resilience (e.g., the capacity to detect, absorb, and adapt to emerging harms), and AI literacy of relevant professionals shape how responsibly a model can be used. A technically safe AI deployed in a region or sector lacking regulatory capacity or social protections can still produce systemic harm. While the EU AI Act rightly identifies high-risk domains, it does not systematically assess whether the institutions deploying AI are equipped to manage and mitigate those risks. For instance, a multinational with in-house legal teams and audit protocols is very different from a small HR platform using an off-the-shelf AI tool with little capacity for oversight—yet both are considered under the same risk classification.
- Institutional accountability and power transparency: Institutions deploying AI systems should be structured in a way that is responsible, contestable, and equitable. That includes clear lines of responsibility, mechanisms to challenge decisions, and visibility into who benefits—and who bears the risks. Without such transparency and redress, even technically compliant systems can entrench asymmetries of power and erode public trust. For example, while the EU AI Act introduces some procedural safeguards, it falls short of guaranteeing meaningful recourse—offering explanations for high-risk AI decisions but no clear right to challenge or overturn them, leaving accountability diffuse and practical redress limited.
- Adaptive oversight and emergent risk: AI systems interact with dynamic social environments, often producing effects that were not foreseen at the time of deployment and risk assessment. Governance must therefore be iterative—capable of monitoring real-world outcomes and responding to new risks as they emerge. Although the EU AI Act mandates post-market monitoring for high-risk systems, its scope remains narrow, as it is mainly provider-driven and focuses on technical compliance and serious incidents, rather than systemic harms or long-term social impacts. As AI systems continue to evolve and are deployed in ever more diverse settings, governance must include clear mechanisms to detect and address the risks that emerge from the specific ways and contexts in which these systems are deployed.
Conclusion
In conclusion, we don’t just need safe models—we need safe deployment ecosystems. As AI becomes increasingly embedded across societies, the risks lie not only in rogue code but in the blind spots of governance: incentives we don’t examine, contexts we don’t assess, and harms we only notice too late. Expanding the governance lens to include the safety of the deployment ecosystem systematically is essential. In the end, what makes AI risky isn’t just its capabilities, but also what we fail to question about the world into which we release it.
Authors
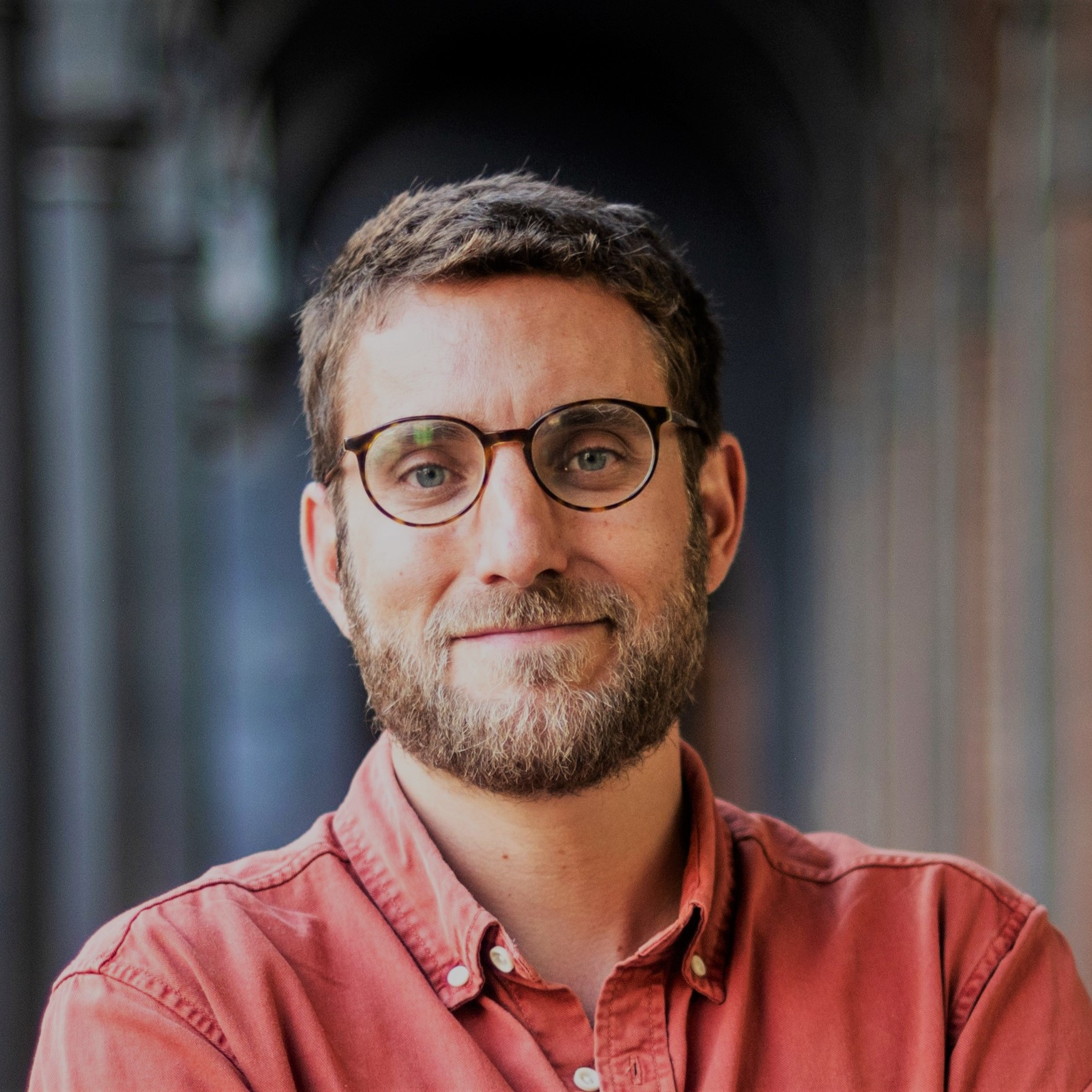