Can We Manage the Risks of General-Purpose AI Systems?
Anthony M. Barrett, Jessica Newman, Brandie Nonnecke, Dan Hendrycks, Evan R. Murphy, Krystal A. Jackson / Dec 5, 2023Anthony M. Barrett is a researcher affiliated with UC Berkeley’s AI Security Initiative (AISI) at the UC Berkeley Center for Long-Term Cybersecurity. Jessica Newman is Director of the AISI. Brandie Nonnecke is Director of the CITRIS Policy Lab at UC Berkeley. Dan Hendrycks is a recent UC Berkeley PhD graduate. Evan R. Murphy and Krystal Jackson are non-resident research fellows with the AISI.
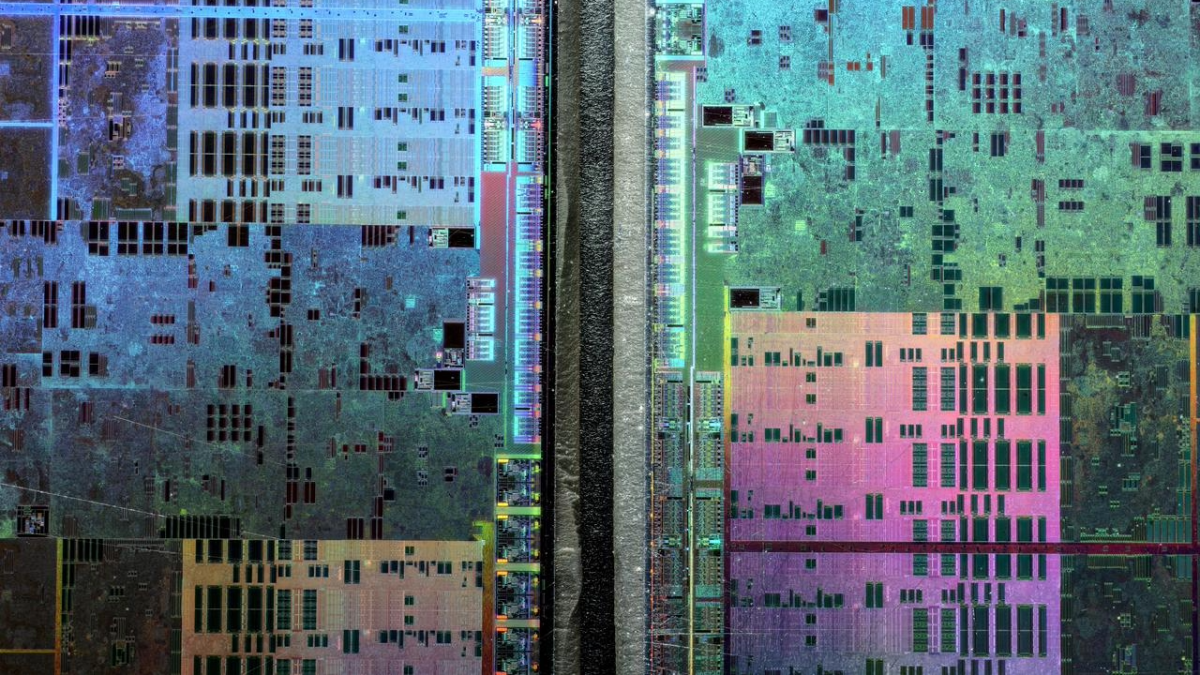
Fritzchens Fritz / Better Images of AI / GPU shot etched 5 / CC-BY 4.0
In the flurry of AI-related summits, executive orders, speeches, and hearings across the world over the past few months, one common sentiment stood out: how do we harness the benefits of powerful, but flawed, AI systems while minimizing the risks? Policymakers are hoping to pass legislation to mitigate the risks of commercial AI products and services to better protect the public. In the United States, this idea was echoed explicitly in Congressional Office of Management and Budget (OMB) guidance, which urges “Federal agencies to strengthen their AI governance and innovation programs while managing risks from the use of AI, particularly when that use affects the safety and rights of the public.”
Of particular concern to policymakers are the massive, cutting-edge generative AI systems developed by a handful of research labs and large companies. These AI systems are also known as general-purpose AI systems (GPAIS), foundation models (a large-scale, high-capability subset or component of GPAIS), or frontier models (the largest-scale, highest-capability subset of GPAIS or foundation models) due to their broad capabilities. (We typically use GPAIS as an umbrella term including the other terms, except where more specificity is needed.) For example, large language models or multi-modal models, such as GPT-4, power both popular chatbots and a range of commercial applications, including the GitHub Copilot software code generation tool. These systems are poised to significantly impact society by providing the foundation on which a variety of new applications have and will be built.
Top AI companies developing and deploying these systems have taken some early steps in establishing responsible capability scaling, red teaming, privacy-preserving techniques, and various other AI governance strategies, including voluntary commitments. However, varied approaches to guardrails for AI create an environment where different understandings of risks and their potential impacts compete with one another. Furthermore, these practices are not always clearly grounded in risk management best practices or policies. The recent leadership upheaval at OpenAI underscores the fragility of any voluntary commitments that depend upon executive buy-in.
The global AI governance landscape is also far from certain. In a particularly concerning shift, multiple EU countries are now seeking to weaken or remove guidance for foundation models from the EU AI Act, despite calls from scholars and experts not to exclude these powerful AI systems due to their serious risks.
Additional policies are needed to better manage the risks of GPAIS and foundation models. Based on a comprehensive policy brief we published last month, we believe these policies should include:
1) further establishing rights-respecting and safety-related standards for GPAIS and foundation models, including evaluation procedures and unacceptable-risk criteria;
2) making such standards mandatory for GPAIS and foundation model developers; and
3) ensuring that regulators have sufficient authority and resources to enforce compliance with such standards.
To help address the first of these and to work toward a more standardized approach to risk management for the most impactful AI systems, our team at UC Berkeley developed a set of practices and controls for identifying, analyzing, and mitigating risks of GPAIS and foundation models, the AI Risk-Management Standards Profile for General-Purpose AI Systems (GPAIS) and Foundation Models. Our approach builds on and is designed to complement the broadly applicable guidance in the US National Institute of Standards and Technology (NIST) AI Risk Management Framework (AI RMF) or a related AI risk management standard such as ISO/IEC 23894, aligning specific portions of our guidance to sub-categories of the NIST AI RMF’s four core functions: Govern, Map, Measure, and Manage.
Risk management is not a one-size-fits-all endeavor. AI developers and users need to adopt the pieces of any risk management framework that will allow them to identify, assess, monitor, and mitigate risks specific to their use cases. However, we have identified what we believe to be the nine minimum best practices for GPAIS and foundation model developers to implement. These include:
- Check or update, and incorporate, each of the following best-practices steps when making go/no-go decisions, especially on whether to proceed on major stages or investments for development or deployment of cutting-edge large-scale GPAIS (Manage 1.1)
- Take responsibility for risk assessment and risk management tasks for which your organization has access to information, capability, or opportunity to develop capability sufficient for constructive action, or that is substantially greater than others in the value chain (Govern 2.1)
- Set risk-tolerance thresholds to prevent unacceptable risks (Map 1.5)
- Identify reasonably foreseeable uses, misuses, and abuses for a GPAIS (e.g., automated generation of toxic or illegal content or disinformation, or aiding with proliferation of cyber, chemical, biological, or radiological weapons), and identify reasonably foreseeable potential impacts (e.g., to fundamental rights) (Map 1.1)
- Identify whether a GPAIS could lead to significant, severe, or catastrophic impacts (e.g., because of correlated failures or errors across high-stakes deployment domains, dangerous emergent behaviors or vulnerabilities, or harmful misuses and abuses) (Map 5.1)
- Use red teams and adversarial testing as part of extensive interaction with GPAIS to identify dangerous capabilities, vulnerabilities, or other emergent properties of such systems (Measure 1.1)
- Track important identified risks (e.g., vulnerabilities from data poisoning and other attacks or objectives mis-specification) even if they cannot yet be measured (Measure 1.1 and Measure 3.2)
- Implement risk-reduction controls as appropriate throughout a GPAIS lifecycle (e.g., independent auditing, incremental scale-up, red-teaming, and other steps) (Manage 1.3, Manage 2.3, and Manage 2.4)
- Incorporate identified AI system risk factors, and circumstances that could result in impacts or harms, into reporting and engagement with internal and external stakeholders (e.g., to downstream developers, regulators, users, impacted communities, etc.) on the AI system as appropriate (e.g., using model cards, system cards, and other transparency mechanisms) (Govern 4.2)
To test how well this guidance could work in practice, we applied it retrospectively to four foundation models: GPT-4, Claude 2, Palm 2, and Llama 2. To rate the level of fulfillment of each guidance step that we found for each model, we used a straightforward rating system of High/Medium/Low fulfillment, with a fourth Unclear rating when insufficient information was found.
This testing was limited because we relied exclusively on publicly available information about these models in our evaluations, and also because as a third party we were applying the guidance retrospectively, rather than applying it during model development as developers would. However, it still can help illustrate what our high-priority guidance steps mean in the context of real-world systems. Below is a summary table, with detailed rationales for each of our ratings provided in Appendix 4 of the profile.
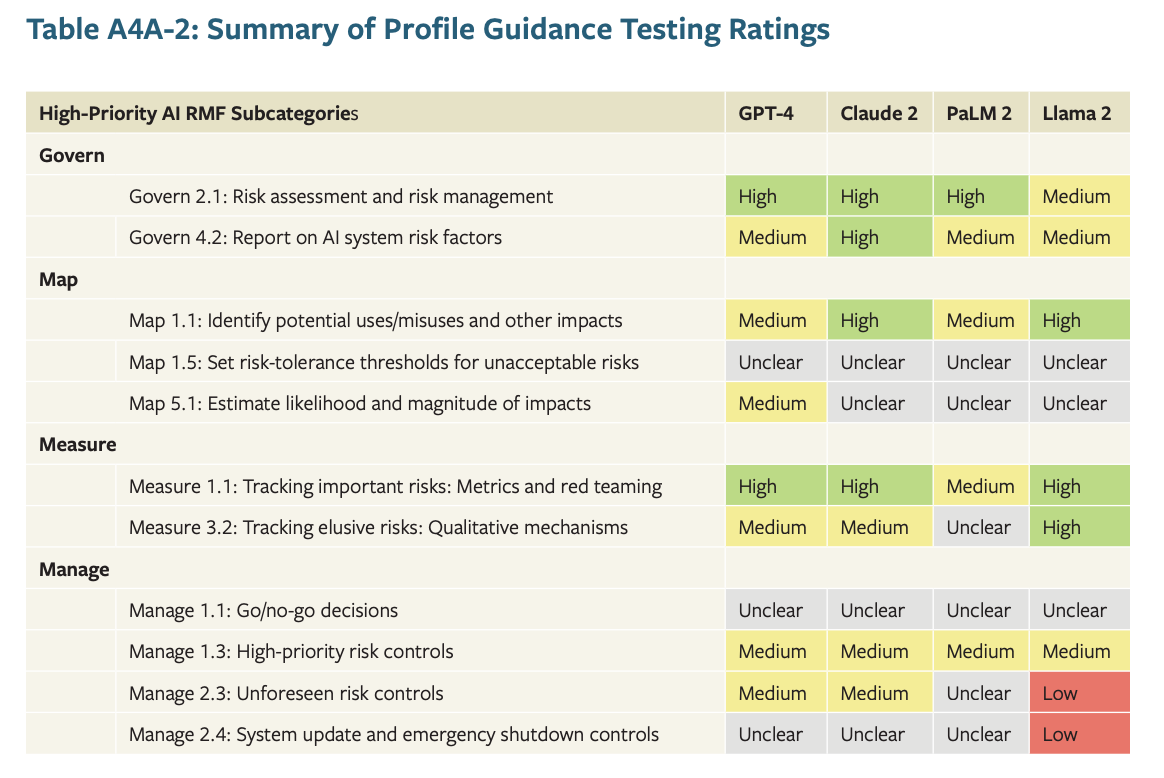
We believe the AI Risk-Management Standards Profile for General-Purpose AI Systems (GPAIS) and Foundation Models will be useful to GPAIS developers, regulators, auditors and evaluators, and other standards development stakeholders during this time of urgent interest in AI risk management. However, we do not consider the guidance to be perfect. We will continue improving and revising our standards profile to keep it up to date with a technology and an industry that makes most things previously called “fast-paced” seem charmingly sluggish. We also do not see the guidance as sufficient in and of itself, but rather as one small part of the broader landscape of policies, practices, and regulations needed to manage the risks of GPAIS. Standards development is just a first step, and should be followed by the implementation of mandatory standards for foundation model developers and by ensuring that regulators have the authority and resources to enforce compliance.
The recent Executive Order on AI has tasked many parts of the US government with roles in helping to promote the safe, secure, and trustworthy development and use of AI. For example, NIST’s role in developing standards and guidance for AI has only grown and includes the establishment of the US Artificial Intelligence Safety Institute as well as the creation of dual-use hazard assessment guidance.
Federal legislation is also needed to strengthen the objectives outlined in the executive order, including by making appropriate rights-respecting and safety-related standards part of hard-law regulatory requirements for frontier model developers, rather than just soft-law voluntary guidelines. For example, recent bills with standards-based approaches include the Federal Artificial Intelligence Risk Management Act of 2023, and the Artificial Intelligence Research, Innovation, and Accountability Act of 2023. We hope to see such bipartisan efforts continue.
The risks of GPAIS and foundation models are complex—they include privacy leaks, job automation, misinformation, novel safety and security vulnerabilities, and threats to human rights and wellbeing, among others. Managing these risks requires evaluation, testing, and auditing models, as well as studying human interaction and the contexts of use, and investigating broader societal and systemic impacts. Foundation model developers must be held accountable for managing the risks they are best positioned to understand and address. Understanding the full picture of risks and impacts and appropriate interventions requires input and collaboration among industry, government, academia, and civil society. Our profile, developed with feedback and engagement from more than 100 people across these sectors, is a step toward this necessary collaboration.
Authors
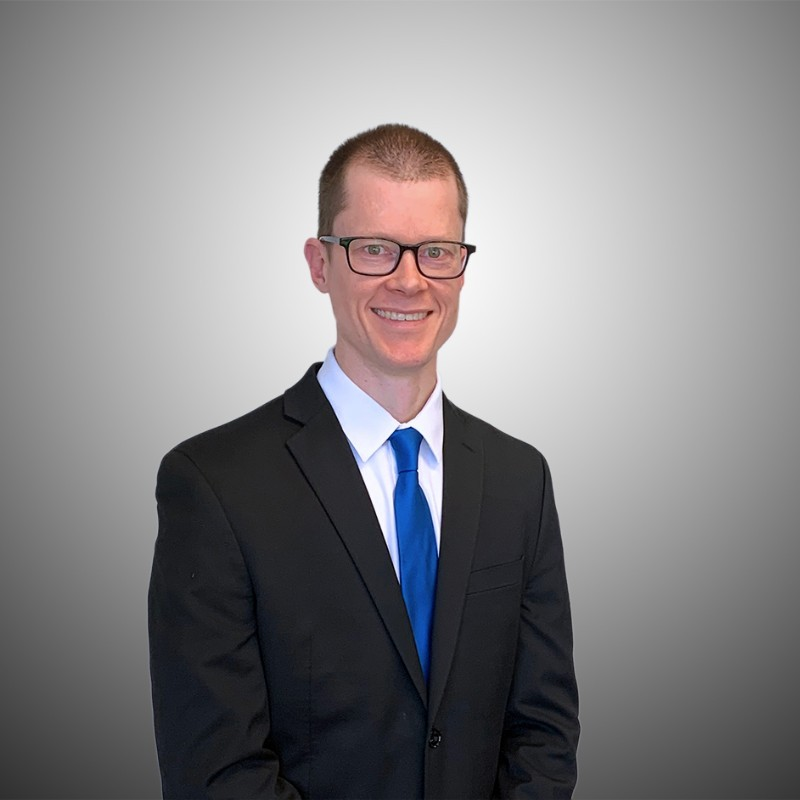
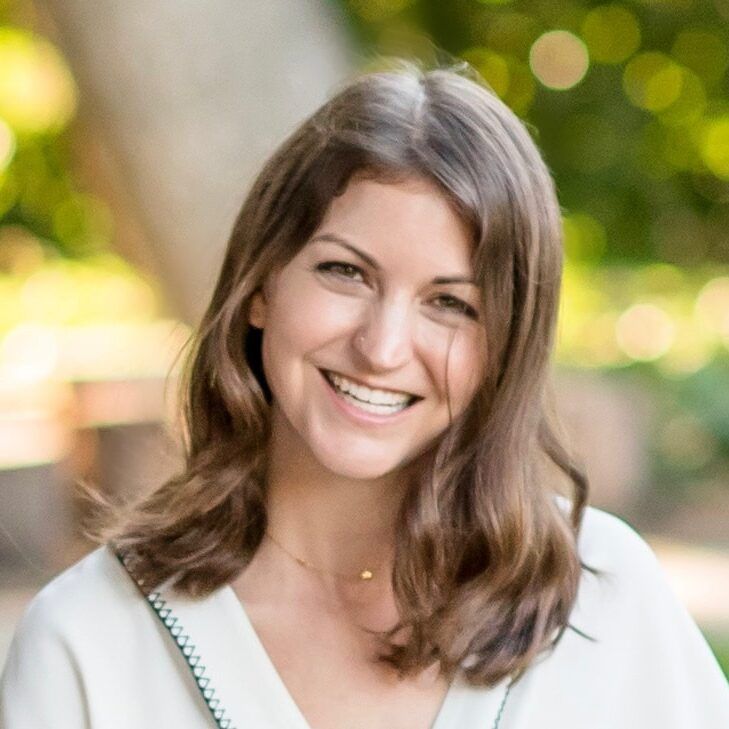
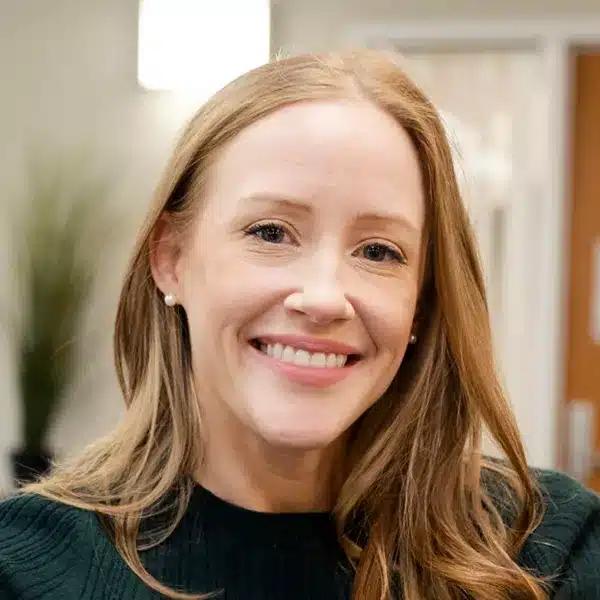
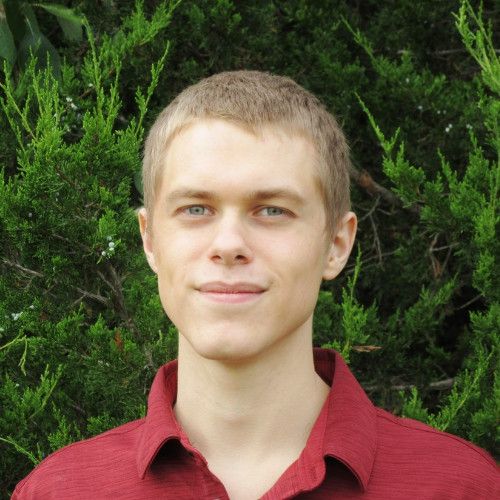
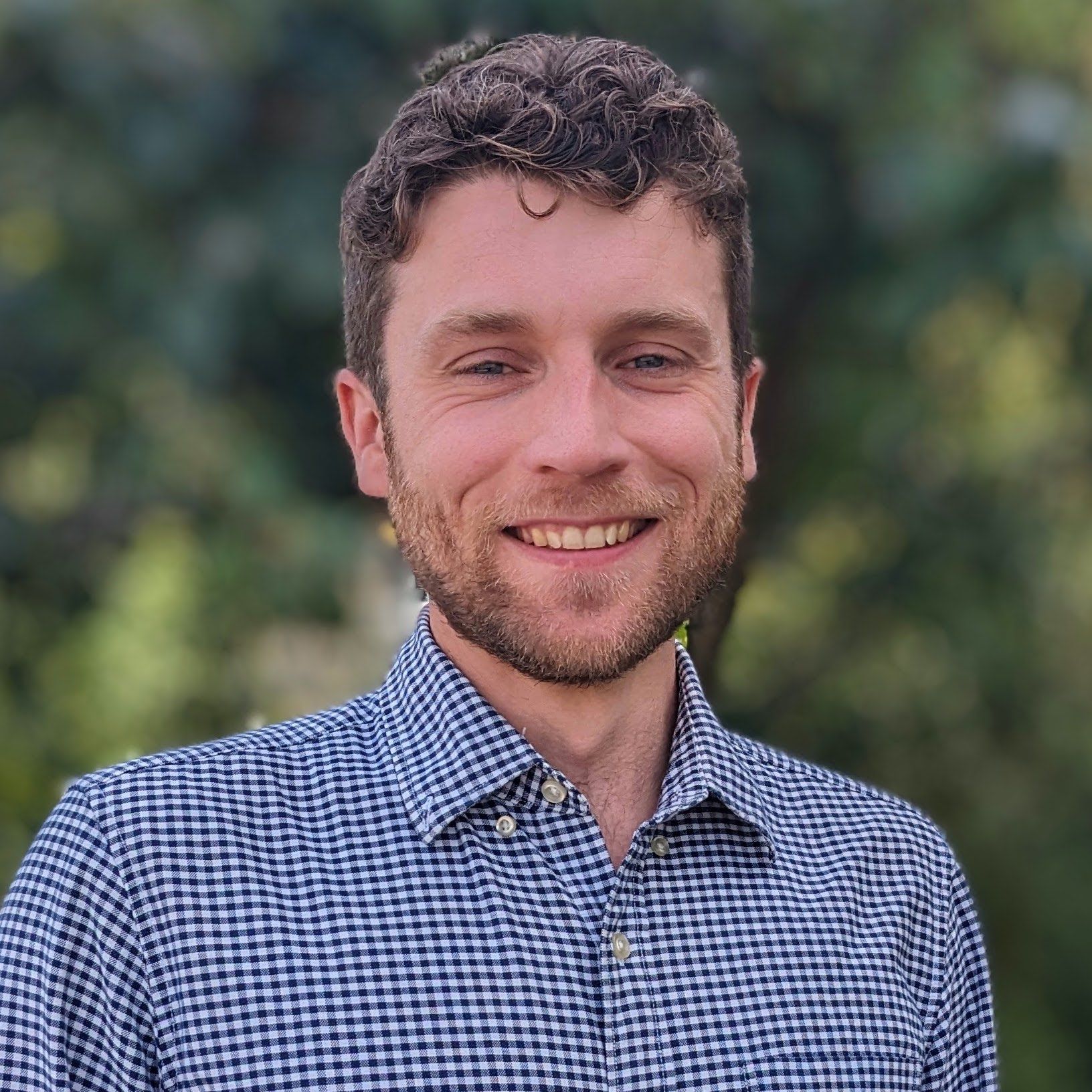
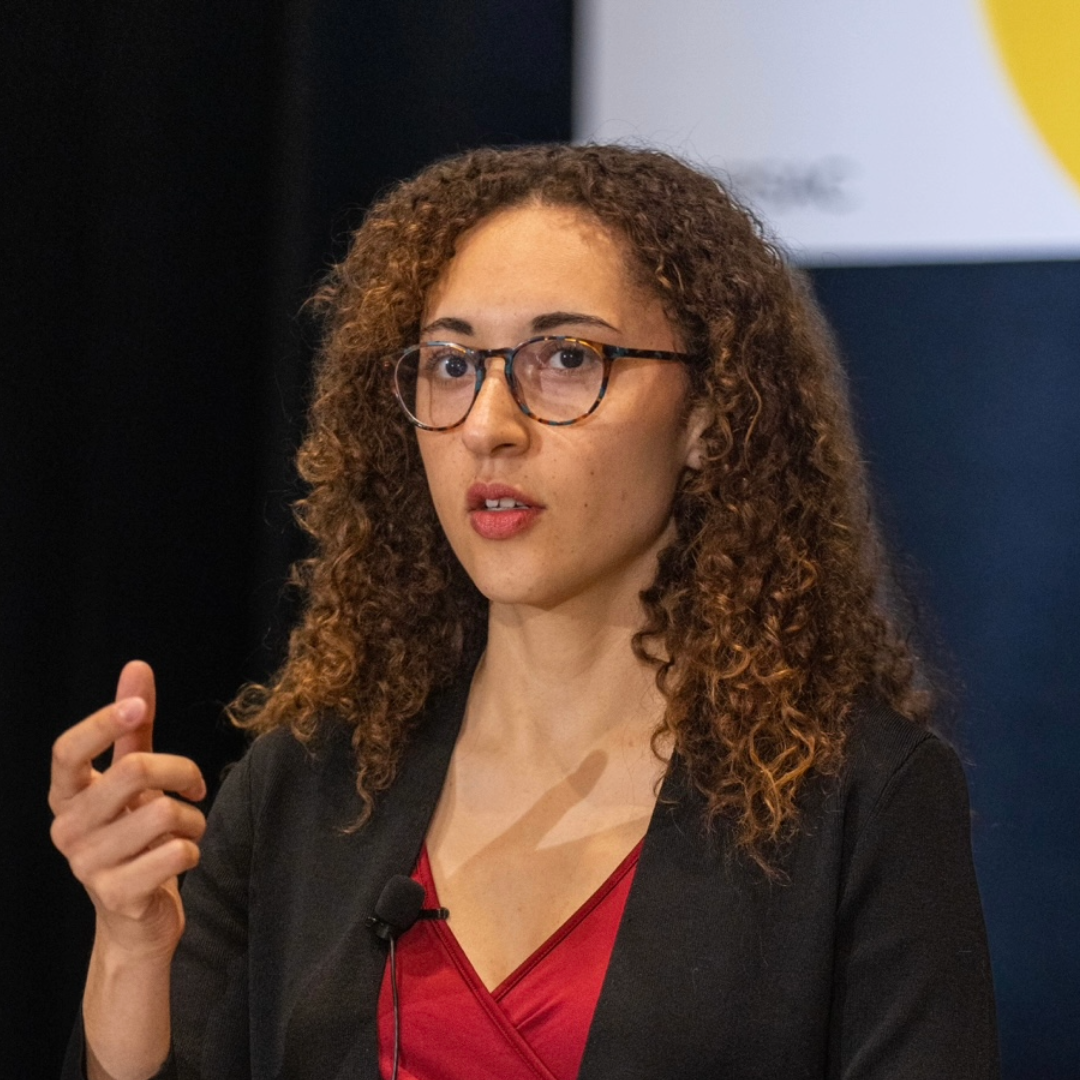