Compute Accounting Principles Can Help Reduce AI Risks
Krystal A. Jackson, Karson Elmgren, Jacob Feldgoise, Andrew Critch / Nov 30, 2022Krystal Jackson is a visiting AI Junior Fellow at Georgetown University’s Center for Security and Emerging Technology (CSET), where Karson Elmgren is a research analyst and Jacob Feldgoise is a data research analyst. Andrew Critch is an AI research scientist at UC Berkeley’s Center for Human-Compatible AI (CHAI), and also the CEO of Encultured AI, a small AI-focussed video game company.
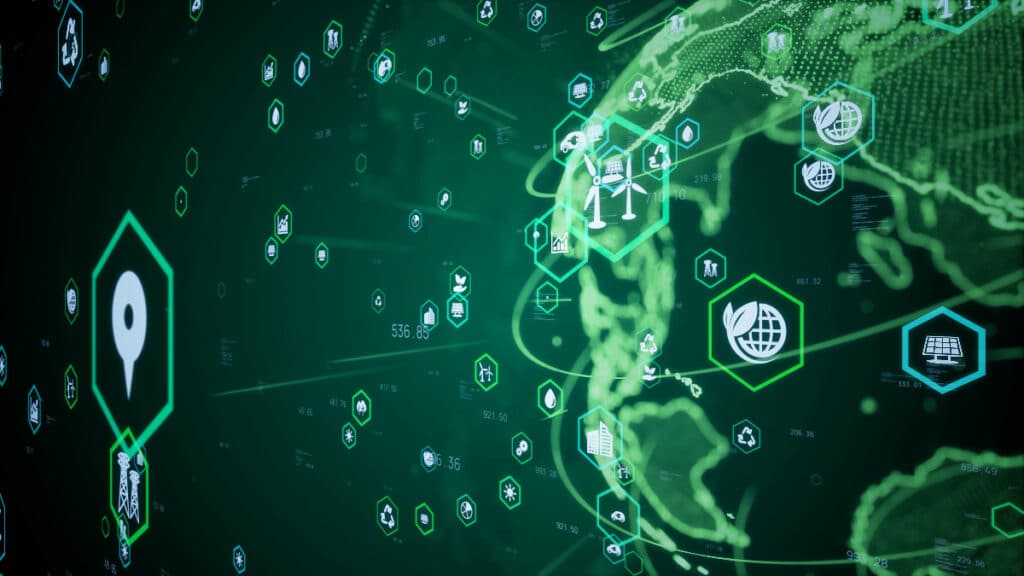
Computational power, colloquially known as “compute,” is the processing resource required to do computational tasks, such as training artificial intelligence (AI) systems. Compute is arguably a key factor driving AI progress. Over the last decade, it has enabled increasingly large and powerful neural networks and ushered in the age of deep learning. Given compute's role in AI advances, the time has come to develop standard practices to track the use of these resources.
Modern machine learning models, especially many of the most general ones, use orders of magnitude more compute than their predecessors. Stakeholders, including AI developers, policymakers, academia, and civil society organizations, all have reasons to track the amount of compute used in AI projects. Compute is at once a business resource, a large consumer of energy (and thus a potential factor in carbon emissions), and a rough proxy for a model’s capabilities. However, there is currently no generally accepted standard for compute accounting.
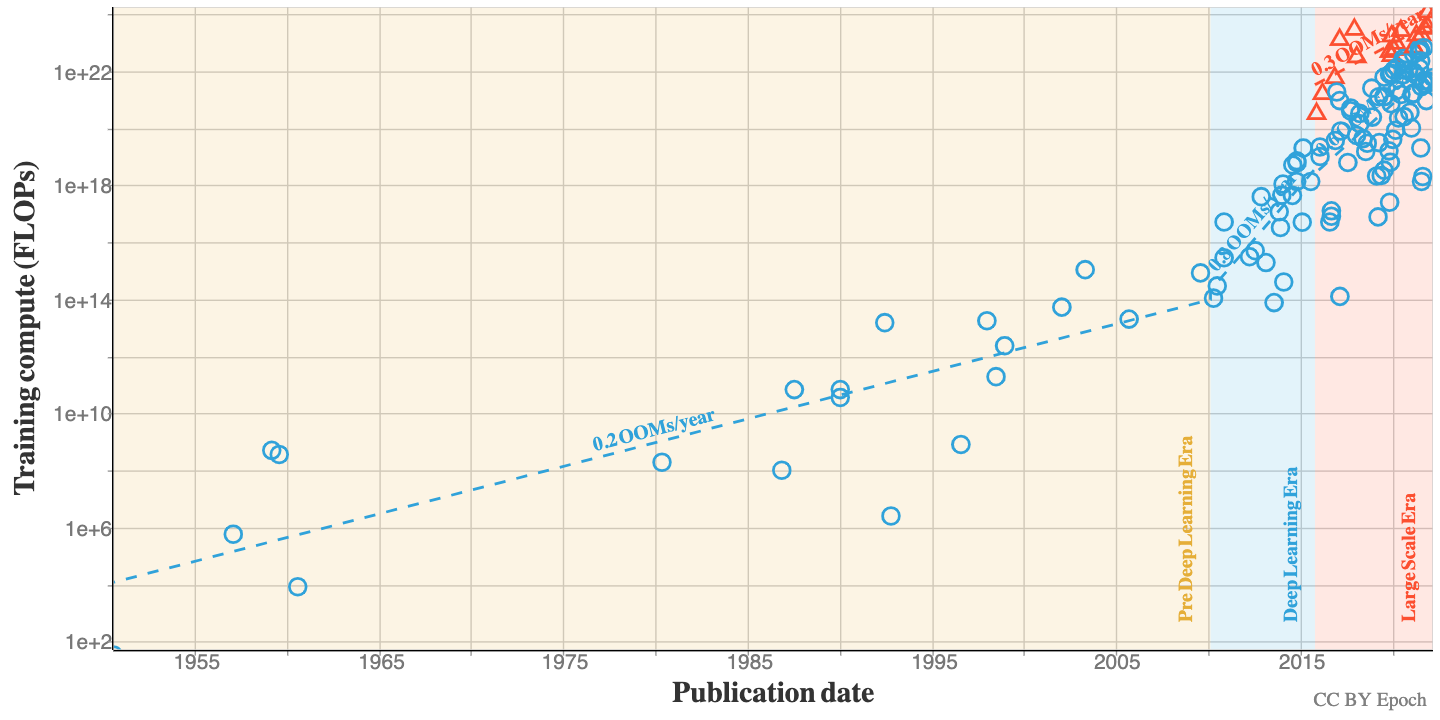
There are two critical reasons for compute accounting standards: (1) to help organizations manage their compute budgets according to a set of established best practices and (2) to enable responsible oversight of AI technologies in every area of the economy. AI developers, government, and academia should work together to develop such standards. Among other benefits, standardized compute accounting could make it easier for company executives to measure, distribute, and conduct due diligence on compute usage across organizational divisions. Moreover, we need such standards to be adopted cross-industry, such that top-level line items on accounts can be compared between different sectors.
Many large companies already build substantial internal tracking infrastructure for logging, annotating, and viewing the project-specific usage of compute. Cloud-computing providers, such as Amazon Web Services (AWS), Google Cloud Platform, and Microsoft Azure, provide users with tools to track how their resources are spent. However, there is not yet an industry standard to document compute usage.
This absence of standardized compute accounting contrasts sharply with the situation for other essential resources and impacts that span across industry sectors, like financial assets, energy, and other utilities, as well as externalities such as carbon emissions, which are tracked using accounting standards. For instance, companies do not invent their own financial accounting software to keep track of money; they use ready-made solutions that work across banks and payment platforms. A single company can easily use multiple banks at once and consolidate all of its revenue and expenditures into a single standardized bookkeeping system using Generally Accepted Accounting Principles (GAAP). Standard practices enable apples-to-apples comparisons between organizations, which in turn fosters trust between investors, lenders, and companies. This trust adds significant economic value by facilitating well-informed transactions of all kinds.
In contrast, the absence of a compute accounting standard makes it challenging to exercise due diligence and audit compute usage. Both activities rely on consistent measurement and record-keeping, which currently does not exist across the industry or even, in some cases, between a large company's divisions. This makes it more difficult for companies to conduct due diligence, for organizations to track and audit their use of these resources, and for governments and researchers to study how compute relates to AI progress, risks, and impacts. For example, without a compute accounting standard, measuring the environmental impact of AI training and inference has proven to be challenging.
There are many unanswered questions concerning the best approaches for compute accounting standards which further research should address:
1. Tools for Companies
With vendor-agnostic compute accounting tools, small companies would not need to invent their own compute accounting practices from scratch; they could simply employ publicly available best practices and tools. Furthermore, if compute providers offered usage reports in a standardized format, then consumers of compute — small and large businesses alike — could more easily track performance across their usage of multiple providers simultaneously. Instead of copying or reinvesting in these systems, companies could reduce costs by picking from a menu of accredited standards from the beginning. A mixture of copying and reinvention already happens to some degree, and there are efficiency gains to be made by standardizing the choices involved at start-up time.
How researchers can help: Continue to build and develop open-source tools for estimating and reporting compute usage. Several programming libraries and tools exist to calculate compute; however, many only estimate compute usage instead of measuring it, while others are vendor specific. Software developers could create general compute accounting tools to build a foundation for implementing practices and standards.
2. Tracking Environmental Impacts
Compute accounting standards could help organizations measure the environmental impacts of their business activities with greater precision. The cost of compute has decreased significantly, enabling many resource-intensive AI projects; however, the increase in compute accessibility has also increased the risk of high-carbon emission projects. Standards that facilitate tracking environmental impacts as part of a risk calculation could allow organizations to manage resources to meet their environmental goals and values. Tracking compute in a standardized way would help elucidate the relationships between energy use, compute, and performance in order to better manage tradeoffs in building AI systems.
How researchers can help: More research is needed to evaluate the environmental impacts of AI. We do not fully understand where and how energy is used in the AI development pipeline. When developers report final training information, they usually do not include previous training runs or consider how energy is sourced. Research into the environmental impact across the AI pipeline and how we can track that impact would help inform metrics and reporting practices.
3. Critical Resource Tracking
A standard compute accounting measure would enable industry-wide tracking of this critical resource. Such a standard would make it easier for industry associations and researchers alike to study how compute is distributed. A standard would also help policymakers decide whether additional measures are needed to provide equitable access to compute—building, for example, on the mission of the National AI Research Resource.
How researchers can help: Determine what barriers exist to equitable access to computational resources. Identify the best ways to measure and track these disparities so the resulting data can be used to help remedy inequity.
4. Assessment of Scaling Risk
In addition, careful tracking of compute could aid in risk assessment. As AI systems scale up in some domains, they can exhibit emergent capabilities – ones that were entirely absent in smaller models. Since models with emergent capabilities may pose new risks, organizations should consider imposing additional safeguards and testing requirements for larger AI systems. A consistent means of counting the compute used to train AI models would allow for scale-sensitive risk management within and between organizations.
How researchers can help: Additional research on the scaling properties of different model types would help determine the relationship between compute and capabilities across domains.
- - -
Standards development organizations should convene industry stakeholders to establish compute accounting standards. Specifically, standards bodies such as NIST, ISO, and IEEE should begin working with large companies that have already developed internal practices to track and report compute usage to establish readily-usable standards that are useful to businesses everywhere. Additionally, technology and policy researchers should conduct relevant research to help inform a compute accounting standard. These actions would help realize the benefits of compute accounting for all stakeholders and advance best practices for AI.
Authors
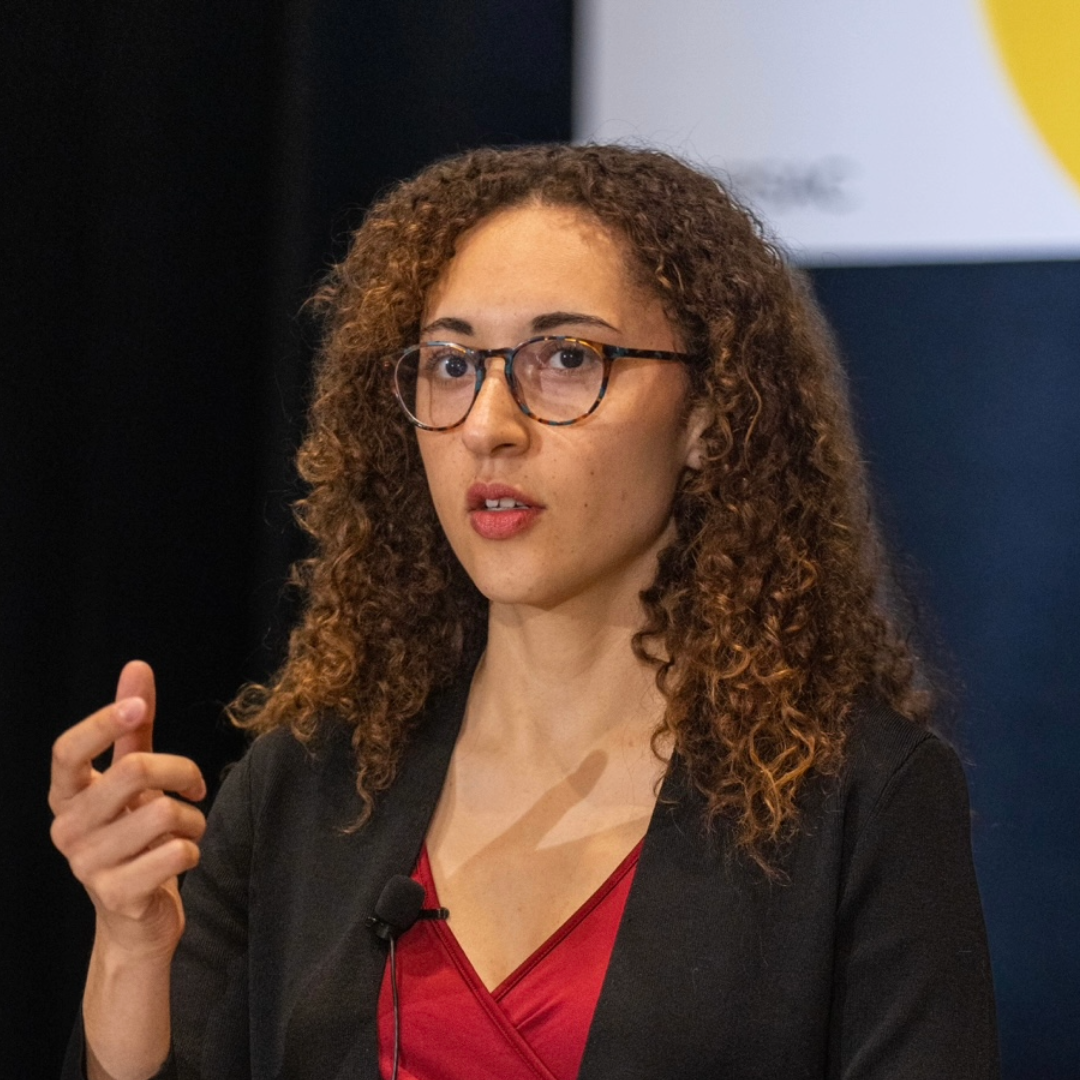
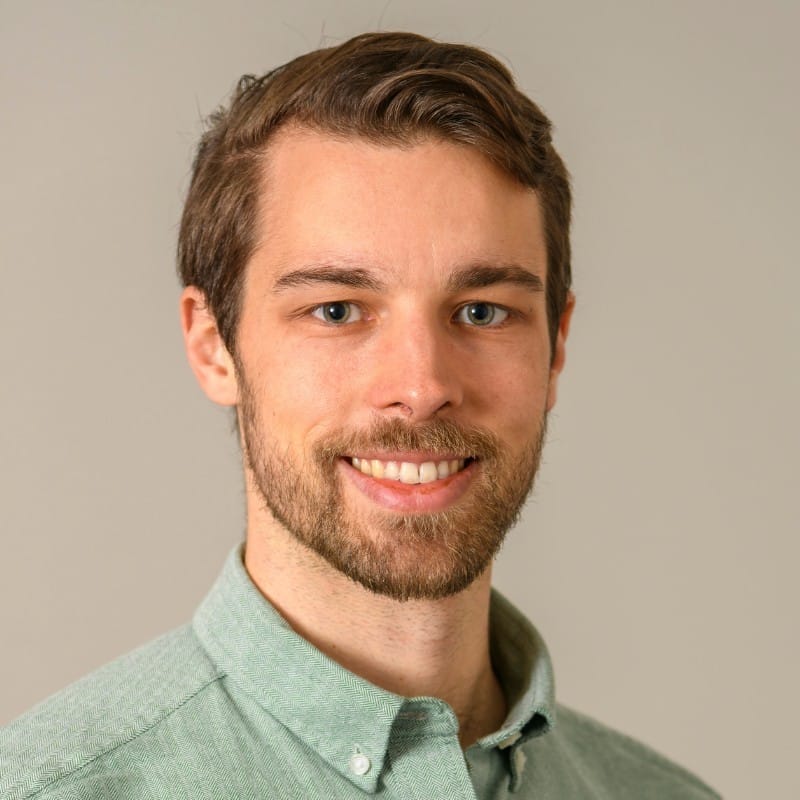
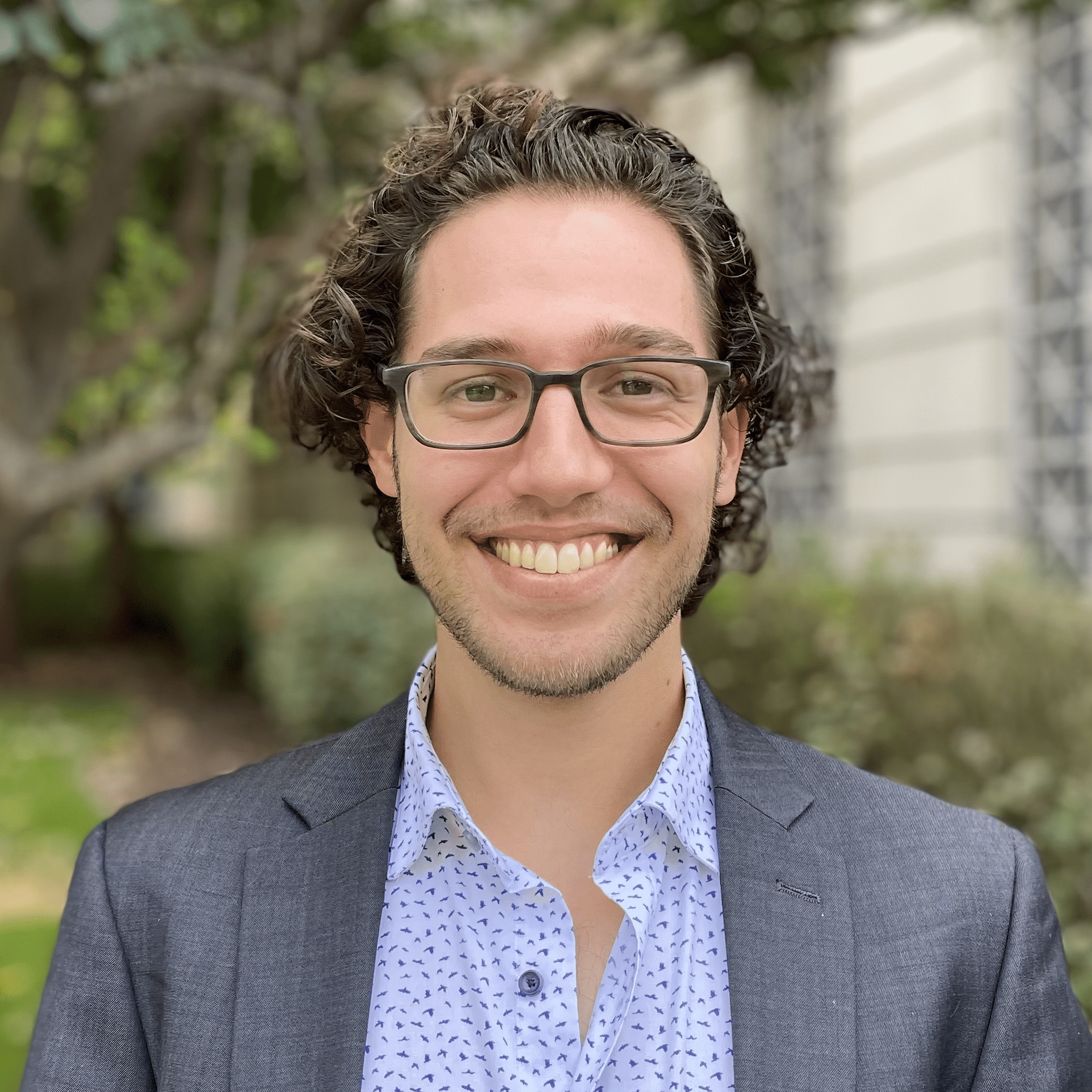
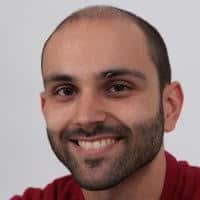