Context, Consent, and Control: The Three C’s of Data Participation in the Age of AI
Eryk Salvaggio / Jun 12, 2024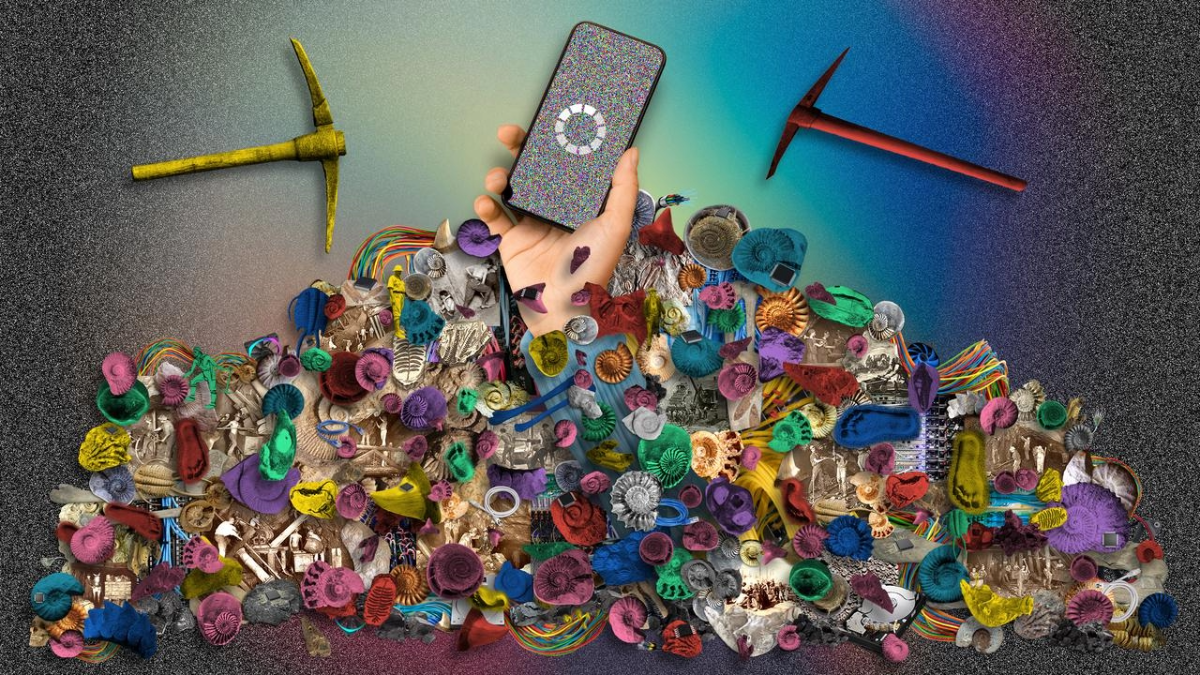
Luke Conroy and Anne Fehres & AI4Media / Better Images of AI / Models Built From Fossils / CC-BY 4.0
What would you want in exchange for your data? It’s an interesting question in the current climate, where most personal data is acquired in ways that feel increasingly extractive and unwanted. Backlash is mounting against a stream of vendors that force users to provide access to data as a requirement to use their products.
When Adobe was accused of locking users out of Photoshop until they accepted a data-sharing agreement, the company had to clarify that this didn’t mean training AI on user photographs. But the user backlash was fierce, and reasonable. Meanwhile, European and UK Facebook users are reporting deep frustration over exercising their right to opt out of AI training by Meta, despite the right to opt-out being protected by the General Data Protection Regulation (GDPR). Meanwhile, LAION 5B — a dataset which has already come under fire for containing images of child abuse — has also gathered images of children along with identifiable metadata.
People are growing ever more frustrated by the intrusiveness of tech. This frustration feeds a cycle of fear that can be quickly dismissed, but doing so strikes me as either foolish or cynical. I am not a lawyer, but lately I have been in a lot of rooms with lawyers discussing people’s rights in the spheres of art and AI. One of the things that has come up recently is the challenge of translating oftentimes unfiltered feelings about AI into a legal framework.
Unfortunately, anti-AI anxiety often presents itself through vague and inexpressible discomfort. It rises up from the body of the AI skeptic as an almost instinctual recoiling, an exasperated physical cringe. Even for those fighting AI through legal mechanisms such as copyright law, this response is deeply unhelpful. Without articulating details of that anxiety, there’s no way to translate the cringe into remedies. For those working on behalf of AI companies, the cringe can be weaponized, too — dismissed as a demand to legislate merely to preserve “warm and fuzzy feelings.”
This phrase has stuck with me, because it points to the incompatibility of case law on copyright with the current AI data grab. There is something that is overwhelming people, often in ways they cannot capture with precise language. If we don’t have the language to describe what’s happening, we certainly don’t have the language to articulate solutions. The legal system’s vocabulary for copyright is completely unaligned with the needs of artists in the current moment. This is true for those demanding strict adherence to copyright laws, as well as those arguing for greater flexibility. It’s hard to make an argument through gritted teeth.
Consider, for example, the case of AI music generation. A system like Udio or Suno is designed to stream generated music on demand to users who type keywords into the system, describing songs. If such a system were to scale to one tenth of the size of Spotify, how would copyright laws respond to every instance of a resemblance to certain song structures? Copyright law has by tradition been decided on a case by case basis. Is the legal system prepared for a world where hundreds of thousands of infringements are generated on a daily basis, distributed across millions of users, with no permanent access to what was generated?
The tech industry is not respecting copyright in the ways that it trains these models, and it will not respect copyright in the way that it distributes the outcomes of these models. Too much of the conversation about AI remains focused on outputs that resemble direct copies of images, music, or text. What is lost is that the data is itself copied into the training model. Regardless of the legal status of that movement from “image” into “data,” it leaves many feeling deeply uncomfortable. Rather than dismiss this discomfort, it merits understanding the source. If case law on copyright cannot support a popular consensus around data rights, perhaps policy changes are needed.
If we want to think carefully about the meaningful management of AI in policymaking — and in establishing norms that protect and encourage sharing various forms of creativity in the public sphere — we need to pay attention to what is signaled through these feelings of discomfort.
Because of my practice as both an artist and a policy researcher — both dealing explicitly with generative AI — I have spoken to countless experts and non-experts. I’ve discussed AI at academic conferences, universities, government roundtables, and on radio programs, with cabinet ministers and policymakers, representatives from human rights organizations, non-profits, and corporations, and the attendees of music and art festivals, as well as librarians, curators, activists, and archivists.
In short, I have been blessed by an opportunity to listen as people have expressed their concerns in vague terms. Rather than dismissing these emotions as uninformed (or over-informed), I’ve been trying to understand what exactly this unease arises from and how it might best be conveyed to people who hope to set them into action.
I would never claim to speak to the concerns of everyone I’ve spoken with about AI, but I have made note of a certain set of themes. I understand these as three C’s for data participation: Context, Consent, and Control.
Context
Too often, data is treated as if it arises from an ahistorical ether. The same word describes collections of user interactions with a website and an archive consisting of family photos. This leads to remarkable imprecision when discussing who should have the rights to what these datasets contain. It is also a frame of particular use to companies which seek to collect more and more information: data is not protected by copyright. Assemble enough data, though, and your dataset becomes copyrightable as a compilation of facts.
In most cases, datasets about images have pointed to the images, pairing these URLs with descriptions relevant to research. The compilation is then protected by whomever assembled it, but this should never trump the copyright status of the material that was being compiled. For example, using a dataset such as Common Crawl to analyze web pages for evidence of hate speech is distinct from using the dataset to find images for training an AI model. A hate speech researcher makes use of the Common Crawl dataset to examine the data it assembles. An AI company makes use of the dataset to find images to download, diffuse, and train on.
It is easy enough for a lawyer to say that Common Crawl is a compilation of facts, and that any text or image that results from an analysis of those facts is fair use. But when we refer to all collective culture as data, we raise questions that defy logic. If I share a photo online, I don’t lose copyright protection over it. But I do lose control over how it is integrated into datasets.
What are the facts of my family photos or LiveJournal entries that make them ‘data’? My family photographs become patterns of pixels to be traced and abstracted into patterns of imaginary families. Poetry becomes a cluster of words to measure the weight of other words.
AI companies seem to suggest they have a right to this data for these purposes. But if I create a song, or an image, and never share it, how would they enforce this right? It is confusing, to the lay user, what it is about a public performance or display of an artwork online that forces us to surrender it. Why does sharing a work on a website turn it into ‘facts,’ while sharing the work with my friends keeps it a work of art? Why does shared performance suddenly strip us of certain rights to the work, as opposed to the common understanding that performance and display is an assertion of those rights?
These are questions of context. We ought to think deeply about the structure of datasets that compile creative works and how they change the works they contain. Without context, the data analysis required for AI feels disquietingly reductive and disempowering. How, then, might we return power to the creator over their work? Likewise, many of us are fine with humans doing whatever they want with the things we share, but feel uncomfortable with the thought of a large corporation taking this material for inhuman processing at scale. Is there really no way to differentiate these contexts of use in policy and legal terms?
Consent
The current regime of data collection is not participatory but mandatory, in hopes of establishing a norm that training doesn’t require consent of any kind. The argument is that AI systems should have the ‘right to learn,’ though models do not exist until they are trained on that data. The navigation of consent in this matter is a challenge that case law struggles to define. We so often see the use of datasets for image training defended as a research exception. AI training is a research project, and copying these datasets for processing advances the cause of AI — a tautology we can’t unpack here. But even if we grant that the models that result from research practices produce scientific knowledge, the next step — monetizing access to those models — lies beyond the research exception. Even if we accept the argument of a ‘right to learn,’ there is no implied right to monetize this learning at the expense of others.
What people might want in order to share this data with others? For many, it is to be asked for it, but also being informed of how that data might be used. People may be more willing to share their data for models that are open access, allowing transparency and non-commercial uses. People may be opposed to AI in principle: many I have spoken to are fundamentally upset by the mechanization of creative labor, and want nothing to do with training the systems that advance it. Others may be fine with any use of their photographs or text, for any purpose.
At the moment, though, there is no mechanism for making these distinctions. I am heartened by Creative Commons’ work on preference signals (and was delighted to be a part of a recent conversation exploring their possibilities for cultivating participation in the data economy). Preference signals can indicate what a creator sees as an acceptable use of their data, and for which kind of systems. We may be comfortable with an AI tool that can quickly adjust lighting for individual skin tones, while being repulsed by a model that generates fully formed images of diverse people for advertisements. Preference signals would not replace or usurp traditional CC licensing schemes, but would enrich our ability to communicate comfort and consent about AI across broader scales of time and space.
Consent can be paired with context (and control) in that often, the data we make available for one use goes on to be used for something else. This transition from “a thing I shared” to a data point to a dataset, and then to data infrastructure, has been a sour pill for those of us who shared our work online without foreseeing the future of AI models for labor displacement, surveillance, or other unsavory forms of automation.
For preference signaling to provide these assurances, however, it would require some kind of enforcement mechanism. At the moment, this is a challenge to define. To work at all, it would also require a policy imagination that sees datasets as the sum of their parts, not merely things in and of themselves.
Control
Ignoring the emotions related to artificial intelligence may seem like a way to achieve a rational policy position. Indeed, many emotional responses to AI are deeply counterproductive, in that they steer conversations away from present-day problems to focus on aligning hypothetical systems. But it is reasonable for people to feel out of control when they see the hype coming from Silicon Valley. We have seen out-of-touch ads that proudly boast of literally crushing human expression into a tight black box. Many from my generation can’t understand why single moms were fined $1.5 million for downloading mp3s while tech companies can now hoover up our YouTube videos and have seats on government panels about regulating AI. While oriented in distinct legal precedents, it feels overwhelmingly unfair.
The emotional piece of this is often driven by the overwhelming sense of scale. The scale of AI systems seem designed to disempower people. It tells us that what is personal to us is meaningless, just another number in a system, and that we have no say in how our information may be used simply because of the vast sums of information that have been taken from so many people. It is another tautology among many, declaring that there’s so much data involved in AI that nobody’s data matters at all. The emotional destabilization of this assertion reaches beyond the law. It speaks to a loss of control over the speed and purpose of the artificial intelligence industry by those whose data it has relied on to exist.
“Warm and fuzzy feelings” emerge when people feel that they have control over a system, can see their inputs into it, and steer into or away from uses they want to see in the world. The opposite occurs when we feel compelled to participate in a system that produces negative consequences beyond our control. Many of these forms of control exist in other industries, with varying degrees of political power and effectiveness. Often these are market-based solutions to failures of policy, such as buying a carbon offset from an airline or socks that donate a matching pair to a homeless shelter. I can also choose to boycott companies I disagree with, and I am not obligated to buy stocks in weapon manufacturers.
Yet, AI seems to be inescapable, monitoring us not only online but in the real world. This palpable sense of paranoia is anything but irrational. It’s baked into the history of data-driven AI. An early example came in 2019, when a live-streamed laundromat in San Francisco was captured by researchers at Stanford and shared as a dataset. The dataset was eventually used by Chinese military researchers for analyzing gait. This analysis of how people walk — and how to identify them — became embedded into a system for identifying oppressed Uighur minorities in China, leading Stanford to pull the dataset. By then, the tech had been built.
If consent is about knowing what I am being asked for, control is about being empowered to refuse it. Yet, it is difficult to see how we make distance from, or disengage completely, from artificial intelligence companies if we wish to exist online. At the moment, there are opt-out registries, such as haveibeentrained.com, which allow people to see — and withdraw — from model training. But not every AI company is required to work with a single database of opt-out requests. As models proliferate, control will continue to diminish as more companies create their own opt-out registries. Opting in by default is bad enough — requiring us to track down every possible model to manually deny its use of our data is not empowerment.
The GDPR protects the right to opt out of training data collection. Recently, Meta has been accused of using dark patterns to thwart opt-outs from European and UK users. By shifting the demand to the consumer for opt-outs, Meta has nothing to lose by designing confusing or broken opt-out mechanisms. In fact, bad design is incentivized: if I am frustrated enough to give up by June 26th, Meta gets my data.
Conclusions
In summary, we might define the three “c’s” of participatory data as:
- Context: When and where can my data be used, and by whom?
- Consent: Inform me of how that data is going to be used.
- Control: Allow me to refuse the use of my data for certain uses.
We are in desperate need of policy considerations for data rights that work to accommodate these preferences, rather than dismissing the desire for “warm and fuzzy feelings” in the AI space as irrational or uninformed. Indeed, many of these concerns may seem naive, as so many of these conflicts have clear explanations when seen solely through the lens of case law. But it is equally naive to assume that these emerging tensions in policy development and norms of use could be resolved through a focus on copyright alone. We might look instead to cultivate willing participation in building online platforms and AI models on human terms.
Authors
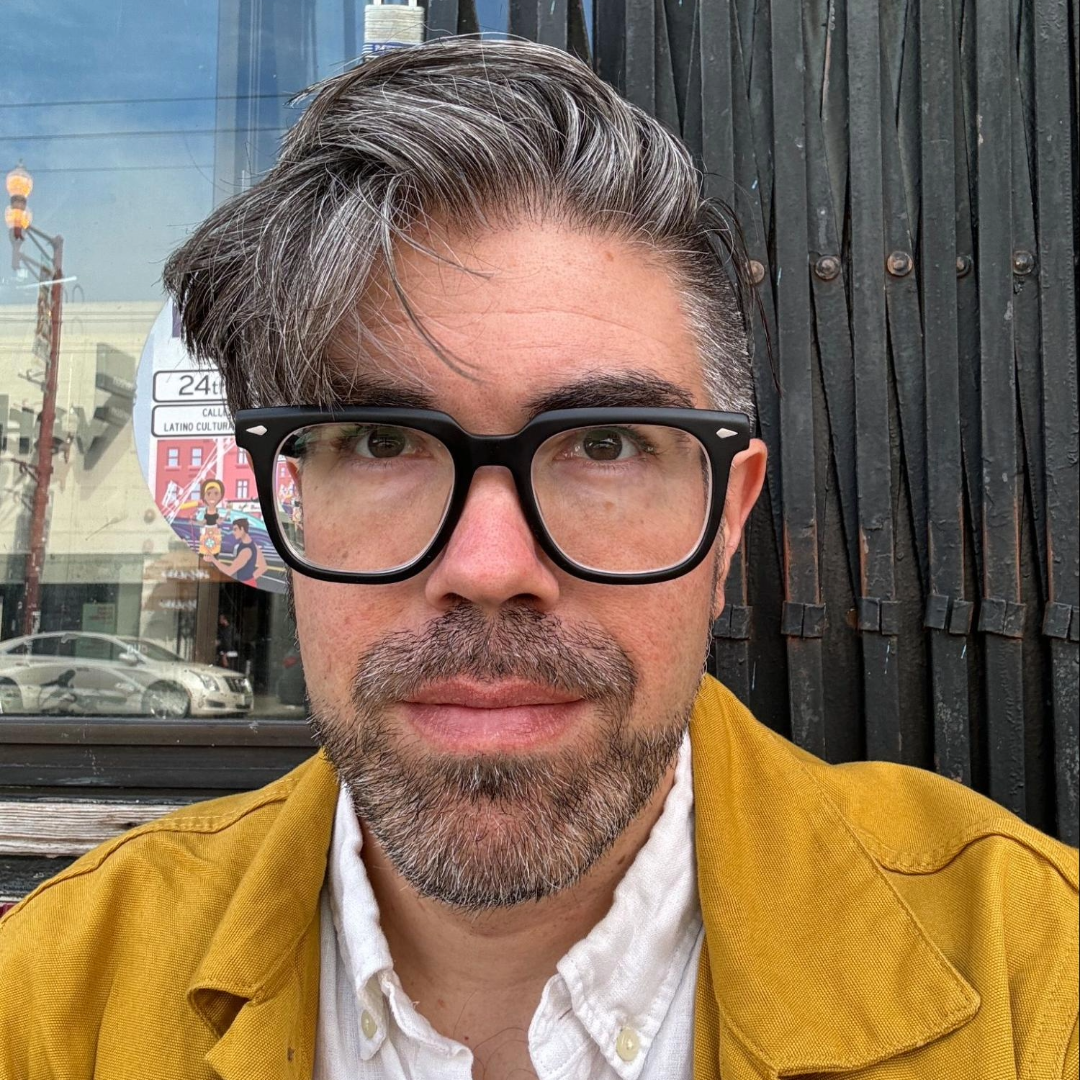