From Doomsday to Due Diligence: A Broader Mandate for AI Safety
Atoosa Kasirzadeh / May 22, 2025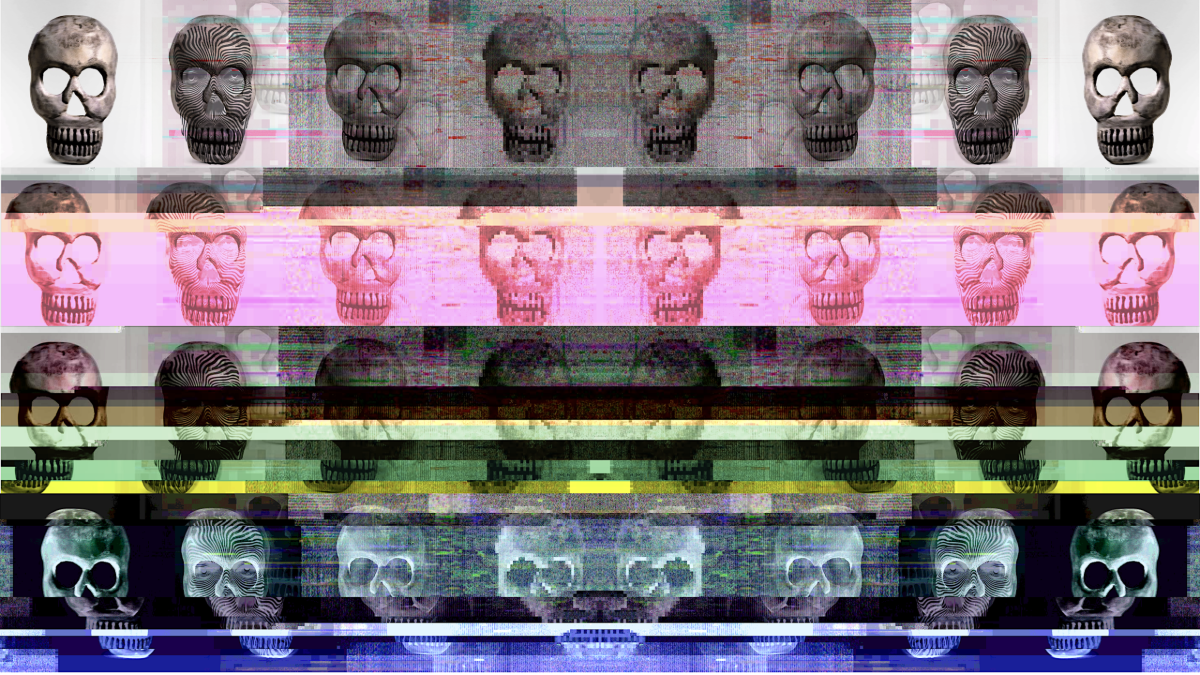
Corruption 1 by Kathryn Conrad / Better Images of AI / CC by 4.0
Over the past decade, congressional hearings, newspaper op‑eds, and pronouncements by tech entrepreneurs have increasingly framed 'AI safety' as primarily addressing 'extinction risk' from AI—the prospect that a future super‑intelligent artificial system could threaten humanity's survival. Invoking human extinction gives the safety of AI systems urgent gravity, but allowing a single extreme scenario to dominate discussion constrains our understanding of what safety for AI technologies can and should address.
In particular, a narrow focus sidelines the work of safety and system engineers who are already fixing today’s brittle systems. It can also persuade the public that safeguards matter only for extreme situations, and make practical lawmakers wary of what might sound like mere science fiction. A credible agenda for the safety of AI systems must keep low‑probability catastrophes on the radar while foregrounding the concrete safety risks we can already measure and reduce.
To see how closely the empirical work on AI safety aligns with the narrative that equates AI safety with mitigating extinction risk from powerful AI, my collaborator, Balint Gyevnar, and I systematically reviewed 383 peer‑reviewed papers published up to November 1, 2023 (see our full paper published in Nature Machine Intelligence here). Our dataset spans flagship computer‑science conferences, interdisciplinary journals, and established safety‑engineering outlets. We observed three clear patterns from our empirical study.
First, published research on AI safety is large and growing: annual publication counts have climbed steadily since 2016, mirroring the spread of machine learning systems into critical domains. Second, safety risk categories are remarkably diverse. Only a very small fraction dealt explicitly with extinction risk, while the others dealt with safety concerns focused on engineering challenges and cybersecurity. Third, most published work on AI safety is pragmatic. Roughly two‑thirds of the papers propose or empirically test concrete mitigation techniques. The remaining studies supply scaffolding familiar to any mature safety discipline. These three patterns echo the historical trajectory of other technological safety fields.
Aviation, nuclear power, pharmaceuticals, and cybersecurity each spawned their own specialized science, yet all share three design principles: build in redundancy and fault tolerance, monitor continuously so deviations are observable and recoverable, and pair technical standards with governance processes that evolve as evidence accumulates. Treating AI as an outlier rather than as the latest entrant in this lineage is both ahistorical and could be strategically unwise. Systematic borrowing from earlier safety regimes—such as the Federal Aviation Administration’s certification model, the Food and Drug Administration’s pharmacovigilance system, or the National Institute of Standards and Technology’s cyber‑framework—can accelerate policy design and avoid relearning expensive lessons.
Anchoring AI safety in broader engineering systems practice offers five concrete advantages for governance. First, legislation can target specific, observable failure modes without requiring lawmakers to adjudicate super‑intelligence AI scenarios. Second, coalition building becomes easier: domain experts in software security, human–computer interaction, ethics, and safety engineering see their expertise recognised, reducing the divide between extinction‑risk advocates and practitioners. Third, oversight can become more agile: tools similar to those proven in medical‑device law fit naturally with AI deployment cycles. Fourth, compliance theatre shrinks: firms align with measurable standards rather than drafting speculative pledges about doomsday prevention. Finally, a pluralistic lens on safety creates room for moral disagreement: societies may never converge on extinction probabilities, but they can still agree that finance models should resist manipulation and autonomous vehicles should obey speed limits.
Two frequent objections deserve a brief response. One argues that present‑day fixes cannot scale to artificial super‑intelligence; yet the history of safety engineering is incremental. Early aircraft inspections evolved into today's stringent airworthiness directives through decades of learning from failures. While AI systems present fundamentally different challenges, the same evolutionary logic could guide the development of deployment-worthiness standards for AI applications. A second objection holds that extinction-from-AI narratives usefully concentrate minds and funding on critical problems. While focus has value, it becomes counterproductive when it crowds out complementary research approaches or erodes public trust in AI governance. Demonstrating tangible short-term safety benefits can legitimize and sustain long-term existential risk investments.
A pluralistic agenda for AI safety implies four lines of action. First, codify baseline safeguards: mandate adversarial‑robustness testing, model‑card disclosures, and secure data governance for any AI system used in safety‑critical or rights‑critical settings. Second, institutionalize incident reporting by creating an AI analogue to aviation’s confidential near‑miss database, complete with shared taxonomies for root‑cause analysis. Third, fund “safety science courts”—interdisciplinary bodies that audit models, replicate claims, and publish advisories, echoing drug‑safety committees. Fourth, invest in open‑source oversight tools for mechanistic interpretability, red‑team simulation, and post‑deployment monitoring, lowering barriers for smaller developers and regulators.
While existential risk from AI remains a legitimate research topic that I've addressed in other work, a particular conception of it — extinction from superintelligent AI — should not be treated as either the only or most urgent cause for AI policy decisions. Recasting AI safety as the newest chapter in systems safety achieves three aims simultaneously: it broadens participation in building safe technology; it grounds debate in the expertise of a diverse body of engineers, social scientists, and ethicists; it roots regulation in observable failures that matter today while scaling to tomorrow’s unknowns; and it preserves intellectual humility about distant threats without letting them crowd out actionable work. AI will reshape critical infrastructure, labor markets, and civic discourse long before it poses species‑level dangers. Governing AI responsibly, therefore, demands a safety agenda as multifaceted as the technology itself—one that is rigorous, inclusive, and humble about both known limitations and unknown unknowns. Narrowing the field to apocalyptic extinction-level scenarios is not just analytically unsound; it results in worse governance. A wider view can save lives now and, by building institutional capacity, better protect the distant future.
Authors
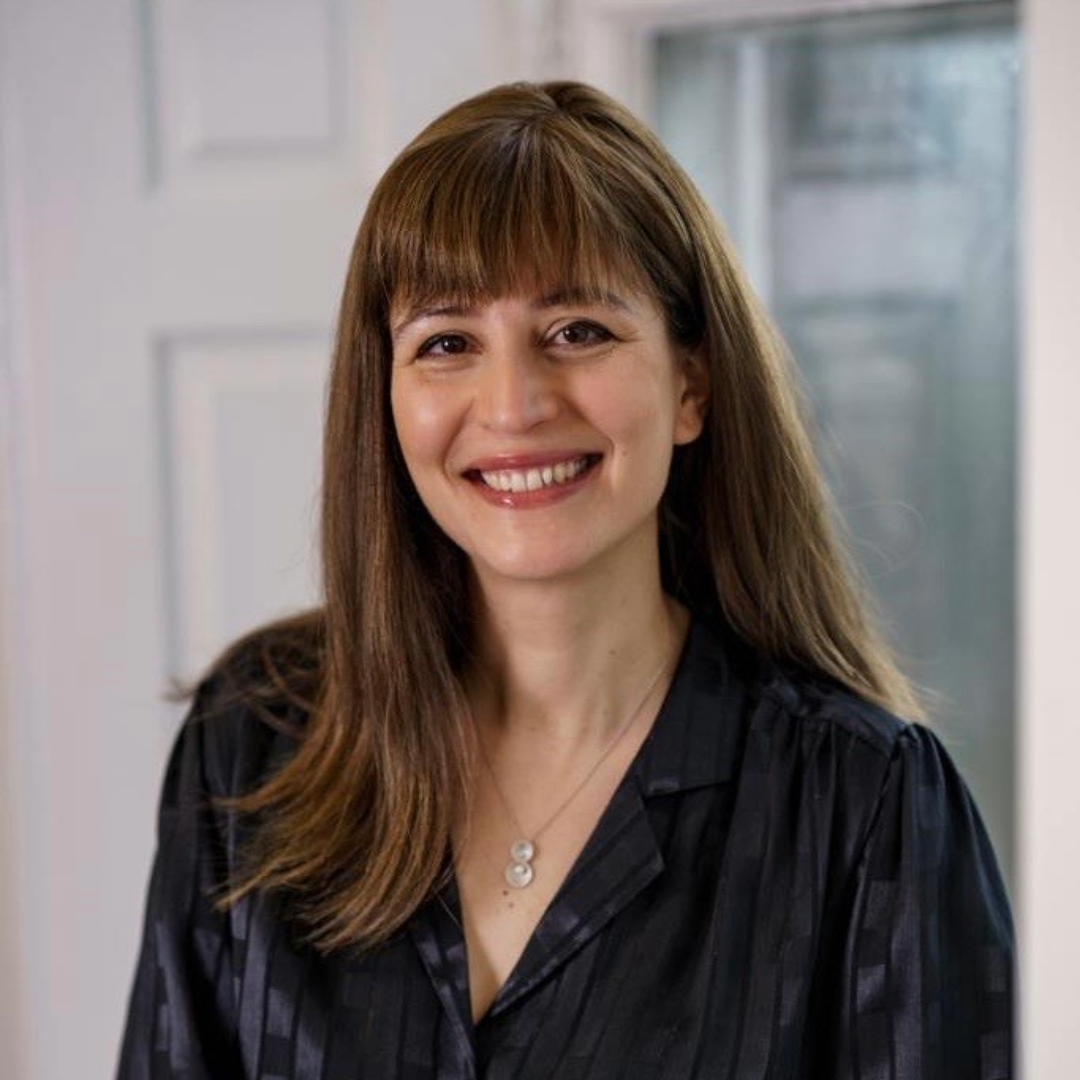