From “Filter Bubbles”, “Echo Chambers”, and “Rabbit Holes” to “Feedback Loops”
Jonathan Stray, Luke Thorburn, Priyanjana Bengani / Apr 17, 2023Luke Thorburn is a doctoral researcher in safe and trusted AI at King’s College London; Jonathan Stray is a Senior Scientist at The Center for Human-Compatible Artificial Intelligence (CHAI), Berkeley; and Priyanjana Bengani is a Senior Research Fellow at the Tow Center for Digital Journalism at Columbia University.
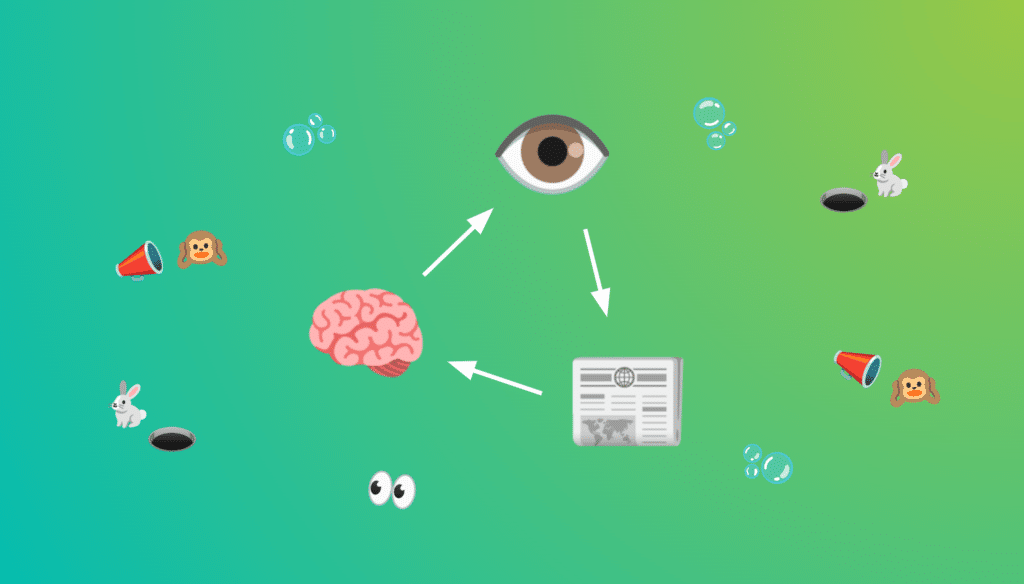
While concepts such as filter bubbles, echo chambers, and rabbit holes are part of the popular wisdom about what is wrong with recommender systems and have received significant attention from academics, evidence for their existence is mixed. It seems like almost every research paper uses different definitions, and reaches different conclusions depending on how the concepts are formalized. When people try to make the question more precise, they usually head in different directions, so the results they come up with are no longer comparable.
In this post, we recap the history of these concepts, describe the limitations of existing research, and argue that the concepts are ultimately too muddied to serve as useful frameworks for empirical work. Instead, we propose that research should focus on feedback loops between three variables: what is engaged with, what is shown, and what is thought by users. This framework can help us understand the strengths and weaknesses of the wide range of previous work which asks whether recommenders — on social media in particular — are causing political effects such as polarization and radicalization, or mental health effects such as eating disorders or depression.
Currently, there are studies which show that bots programmed to watch partisan or unhealthy weight loss videos tend to be shown more of them. There are experiments showing that political ads and cable news can cause small changes in political opinions, and that manipulating the emotional valence of the Facebook feed can influence the emotions expressed by users in their posts. There is also a long history of “selective exposure” research showing that people often engage more with supporting information, and are more likely to share news articles if they agree with them.
In other words, all of the parts of the engagement–recommendations–beliefs–engagement loop have been demonstrated in at least some context. But the effect sizes tend to be small or context-dependent, and because the specific measures used are not consistent, it is not straightforward to assemble these component results into evidence for a complete feedback loop. To do this, we need studies that use consistent measures for the three variables — what is engaged with, what is shown, what is thought — and research designs that can empirically show the causal relationships between them.
How we got here
Distinctions are often drawn between filter bubbles, echo chambers, and rabbit holes, but they can all be thought of as types of information-limiting environments. Concerns about the role of media and technology in creating such environments have been around since at least the 1950s—from Philip K. Dick’s “homeostatic newspaper,” to Joseph Klapper describing mass communication as an “agent of reinforcement,” to Nicholas Negroponte’s vision of a “Daily Me.”
In the context of the internet, the “echo chamber” concept was popularized by Cass Sunstein in 2001, the “filter bubble” by Eli Pariser in 2011, and the “rabbit hole” — a reference to Alice in Wonderland, or in some instances The Matrix — has been used as a metaphor for independent online investigations since at least the mid 1990s. All three phrases started as informal or quasi-academic concepts that have been repeatedly defined and redefined by researchers attempting to quantify the degree to which such phenomena are real.
We want to be able to answer the question: Do recommender systems cause filter bubbles (or echo chambers, or rabbit holes)? But this question is not specific enough. There are many different recommender systems, and many different ways of defining and measuring information-limiting environments. When people rephrase this question to make it precise and falsifiable, they do so in different ways, so their findings are no longer comparable.
Take the concept of “filter bubble” as an illustrative example. The following list gives a sample of the various ways the concept has been defined.
- “a unique universe of information for each of us” (Pariser, 2011)
- “[a technological] information network from which relevant voices have been excluded by omission” (Nguyen, 2018)
- “when a group of participants, independent of the underlying network structures of their connections with others, choose to preferentially communicate with each other, to the exclusion of outsiders” (Bruns, 2019)
- “the exclusion of the uninteresting at the expense of democracy” (Dahlgren, 2021)
- “a decrease in the diversity of a user’s recommendations over time, in any dimension of diversity, resulting from the choices made by different recommendation stakeholders” (Leysen et al, 2022)
The above definitions are qualitative. When trying to measure filter bubbles quantitatively, people use numbers like:
- The weighted average of the squared difference between the expressed opinion of an individual with their social network neighbors, in a theoretical model of opinion dynamics simulated on the follower networks of real social media platforms. (Chitra and Musco, 2019)
- A “time-weighted percentage of news sites visited [during a 4 week period] with political slant scores opposite to a user’s base ideology.” (Kitchens et al, 2020)
- The average ideological slant of videos that appear in Homepage or Up Next recommendations on YouTube, where the slant of the video is estimated by identifying the partisan elites that are followed by Twitter users who linked to the video. (Haroon et al, 2022)
Inconsistent definitions and measurements are not the only limitations of existing research. Portions of the existing literature have been criticized for using non-representative datasets (e.g. only considering the most active social media users), lacking temporal validity (because platforms so frequently change their algorithms), using only observational data (which cannot disentangle the contribution of user behavior from that of the algorithm), making the revealed preference assumption (conflating user behavior with user preferences), falling for the ecological fallacy (for example, by showing a correlation between rates of internet use and political antipathy across regions — there is no guarantee that the individuals who use the internet a lot are the same people who dislike their political opponents) and, conversely, for making an unsubstantiated leap from individual effects (e.g. information consumption) to societal effects (e.g. democratic resilience or harms).
Because of these definitional and methodological issues, we propose shifting attention from filter bubbles, echo chambers, and rabbit holes to a different concept: feedback loops between humans and machines. This concept seems better defined, can be broken into falsifiable questions about each stage of the process, and might help us better coordinate research efforts to produce lasting insight.
The feedback loop model
In theory, the recommender feedback loop works like this:
When faced with a slate of recommendations, a user will engage with some types of content more than others. This is called selective exposure. For example, they may engage with ingroup content more than outgroup content (due to homophily or confirmation bias), with mainstream content more than indie content (due to cultural pressures), or with radical content more than moderate content (due to sensationalism or outrage). The recommender, because it is at least partially optimizing for engagement, starts to show more of the types of content that are engaged with. This changes what the users see, which causes their beliefs and preferences to change, becoming more aligned with what they see. Finally, this preference change causes the user to be even more selective, perhaps by explicitly following more homogeneous sources, or more consistently ignoring other types of content. Then the loop begins again.
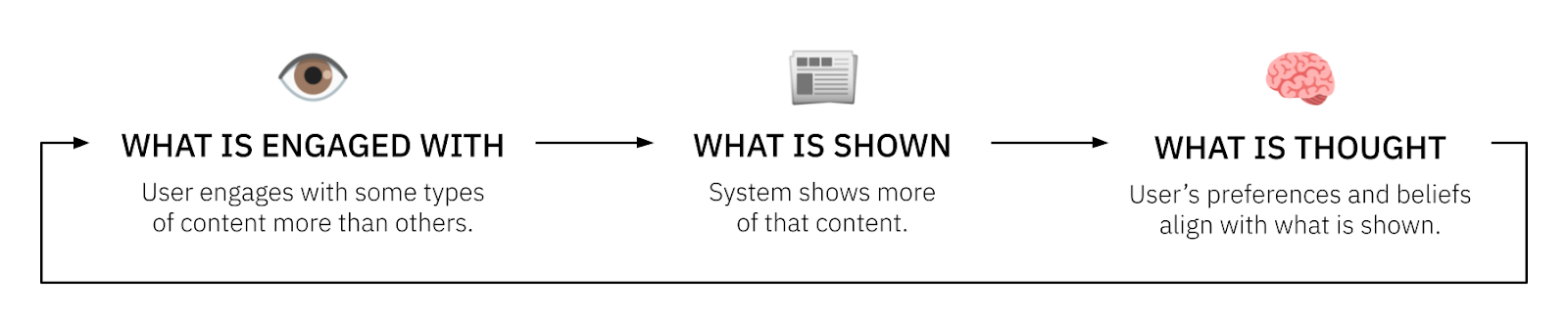
The feedback loop concept isn’t new. Versions of it have been mentioned previously in the literature on filter bubbles, echo chambers, and rabbit holes. In the original book on filter bubbles, Eli Pariser described a recommender “you loop”, which can behave “like reverb from an amplifier.” It is the focus of a recent paper on safe recommender design, and is fundamental to concerns that recommenders might be influencing us (as has been shown to occur in simulations). However, feedback loops are usually not the way that people think about and research these types of problems.
Feedback loops are also not unique to recommenders. They occur in traditional media such as cable news and newspapers, albeit over longer time periods as producers and editors adjust their coverage to chase ratings or respond to the incentives on social media platforms. Also, not all information-limiting environments involve a feedback loop. “Educational” curricula used by extremist organizations for recruitment can be viewed as rabbit holes that are not influenced by the “student,” yet still influence them.
We think there are at least three good reasons to think in terms of feedback loops.
First, the feedback loop framework clarifies the specific research questions which need to be answered to measure the extent to which information-limiting environments are a concern. Concretely, we need to quantify three variables — what is engaged with, what is shown, what is thought — and need to measure three causal relationships between them — (1) how what is engaged with affects what is shown, (2) how what is shown affects what is thought, and (3) how what is thought affects what is engaged with. These variables can be quantified in different ways, so there are different versions of the feedback loop depending on how this is done. But if the three variables are quantified consistently, and there is reliable evidence for the three causal relationships between them, then there is a strong, precise case for a “filter bubble”-like phenomenon.
Second, the model makes explicit that there must be a change in what is thought — the user’s beliefs, opinions, preferences, emotions, etc. — for the phenomenon to be of interest. It is common for existing studies on filter bubbles or echo chambers to only consider partial fragments of the loop. For example, a study may reveal a limitation with regard to what is shown, but not demonstrate that this limitation affects what is thought. But if the user isn’t influenced by what they see, what’s the problem? The concerns about echo chambers, filter bubbles, and rabbit holes assume that people are affected by the content they are exposed to – as opposed to using their own intelligence and agency to decide what to think – and this assumption needs to be tested.
Third, the feedback loop framework can help us talk about practical constraints on the power of recommenders to influence. The feedback loop does not continue forever. At some point, a form of saturation or equilibrium will be reached, perhaps because a diversification stage in the recommender ensures that the recommended content cannot exceed some level of homogeneity, or because people have a finite number of hours with which to engage a recommender, or because people are also influenced by other information sources (e.g. friends and family). The question of how much people can change before equilibrium is reached, and how long this process takes (one loop? more?) is an important one.
Before we move on we note that such feedback loops, if they exist, cannot be attributed solely to a recommender system. Only one of the three segments of the loop– the relationship between what is engaged with and what is shown– is determined by the algorithm and can be (relatively) easily influenced by recommender design changes. The other two relationships — between what is shown and what is thought, and what is thought and what is engaged with — are determined by human psychology and a complex set of other societal factors, and are thus harder to influence. Also, users and creators will adjust whenever the recommender is changed. As an example of the second-order effects involved, consider that content producers will be incentivized to produce content that reinforces feedback loops that lead to them receive more attention. This in turn changes the distribution of content available for recommendation.
Is there evidence for feedback loops?
Feedback loops seem plausible in theory, but are they supported by evidence? Below, we review existing evidence for each of the three causal arrows in the feedback loop model.
Does what is engaged with affect what is shown?
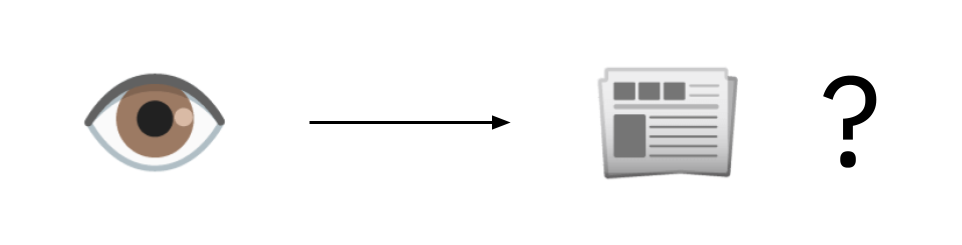
The question of whether a recommender shows more of the types of content that a user engages with might be the least interesting of the three, because this is (part of) what recommenders are designed to do. Responding to our interests makes these information filtering systems much more useful. Nonetheless, the question can be studied empirically, and it is important to do so because the strength of the effect can vary, and most real platforms have built-in safeguards that disrupt the effect for certain types of content (e.g. terrorist content).
Much of the existing literature on filter bubble-like phenomena focuses on the link between engagement and recommendation. For example, recent large-scale studies of YouTube and TikTok show that when a bot is programmed to “watch” certain types of content, they tend to get recommended more of those types of content. Explicitly “following” or interacting with a specific account is also a type of engagement, so studies showing that the accounts users interact with affect what they get shown — such as on Facebook and Twitter — also speak to this question.
Future work on this question would benefit from more consistency in how what is shown is measured. Specifically, the methods used to quantify subjective, qualitative properties of content — such as its political slant — vary greatly, and are difficult to compare. It is also important to distinguish between the slant and diversity of content when assessing changes to what is shown.
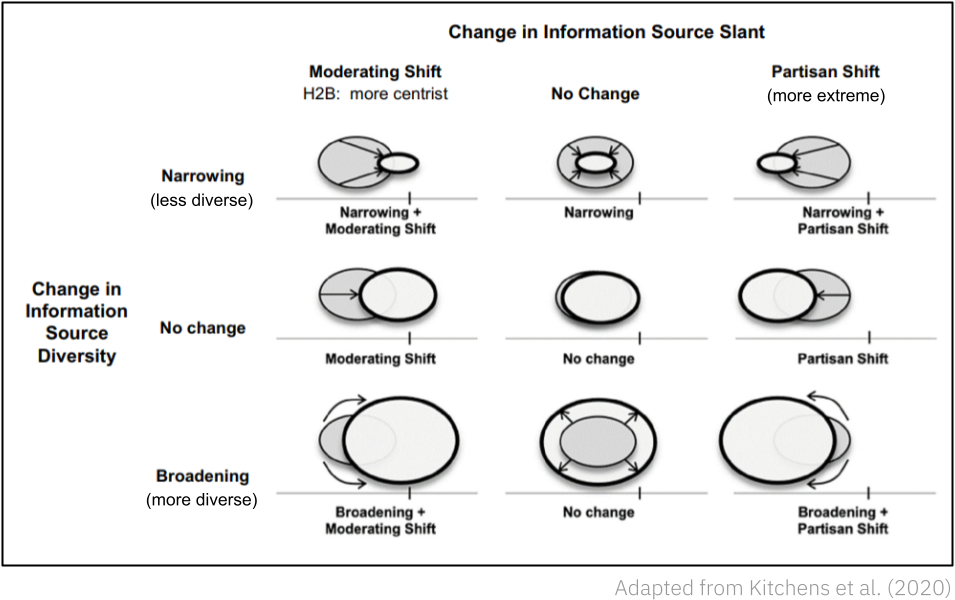
Finally, studies that use bots to assess this question can only tell us so much. Bots may be more fixed or dogmatic in their habits of information consumption than real people, and may not respond to the content they are exposed to in the same way, particularly over the long term. In particular, people do not engage with content deterministically or uniformly at random, and modeling them with bots that behave in this way can significantly overstate the degree to which extreme content is recommended. For this reason, it is important to either study this question in the context of real users, or to put considerable effort into simulating users faithfully.
Does what is shown affect what is thought?
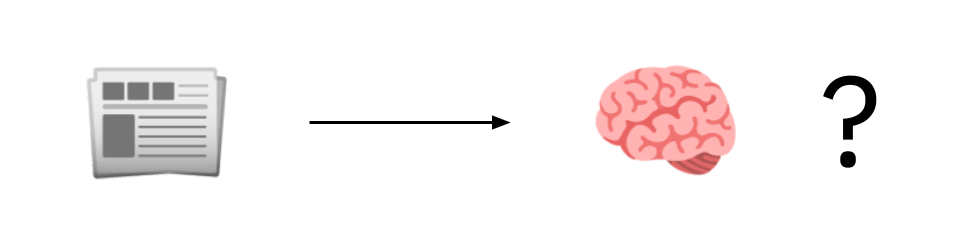
The question of whether the media people are exposed to affects their thoughts, beliefs, or attitudes is the focus of a sizable literature on media effects. To estimate a media effect, it is necessary to focus on particular kinds of thoughts or attitudes or emotions (e.g. political ideology, mental health) and to have some way of measuring them, which usually amounts to either (1) asking people what they think or (2) inferring what they think from what they do.
Most of the existing literature on media effects focuses on advertising or traditional media rather than recommenders. For example, the impact of non-personalized political advertising on voting preferences seems to be small regardless of context, while spending more time watching Fox News has been shown to have a modest effect on voting behavior. In the context of recommenders, the largest examples of media effects experiments we know of are cases where Facebook used a political mobilization message (pinned to the top of the feed) to increase voter turnout, and when that platform changed the emotions expressed in users’ posts by manipulating what they saw in their feeds.
On the one hand, current evidence appears to suggest that the direct effects of individual media items are small, on average (though they can be increased with the use of personalized persuasive messaging). Further, a sizable fraction of people are simply not interested in overtly political media.
Commentators often neglect how little political news most people consume — much of the public is not attentive to politics and thus unlikely to be in an echo chamber of any sort.
— Andrew Guess et al., Avoiding the echo chamber about echo chambers (2018)
If true, media effects overall may be minimal. On the other hand, everything we think is in some sense a response to the information we are exposed to, and changes to that information environment, if large enough, should have significant effects.
There are at least two ways to resolve this paradox. The first possibility is that the media effects of recommenders may be small for most people most of the time, but occasionally much larger for a few individuals (subgroup effects). Current research on recommenders tends to look at average or aggregated effects, and may not be able to detect these sorts of relatively rare large effects. The second possibility is that changes in our thinking do not usually arise from one-off media exposure but from exposure to recurring narratives and themes over the long term (cumulative effects). Both these possibilities can be difficult to observe and need further research attention.
Does what is thought affect what is engaged with?
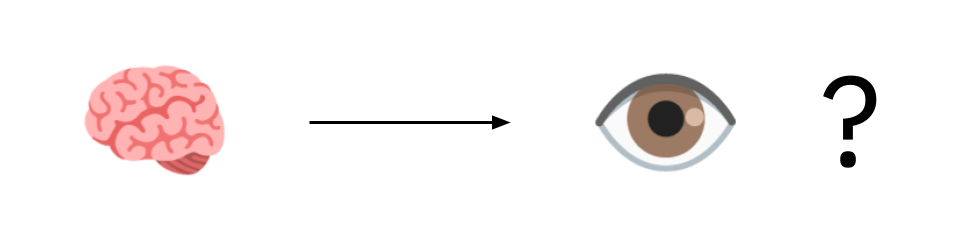
The research area that studies whether what is thought affects what is engaged with is called selective exposure. Generally, it is hypothesized that people tend to engage more with information if it is consistent with their existing thoughts. To assess this claim, we need a way to measure thoughts that is distinct from the way we measure behavior — we can’t assume that people agree with the content they engage with. The easiest option is to simply ask people what they think. Indeed, studies on selective exposure in the context of recommenders usually just ask participants to self-report their political affiliation or beliefs.
It is straightforward to observe a correlation between what is thought and what is engaged with, but that does not reveal whether the relationship is causal. The most straightforward way to assess causality would be to conduct an experiment in which you manipulate what people think and observe the impact on engagement. However, manipulating what people think can be both difficult and unethical. For this reason, many of the experiments on selective exposure manipulate other variables (such as whether like counts are shown) and observe the extent to which this changes the degree of selective exposure observed.
On average, it seems that selective exposure usually has only weak effects.
[These] tendencies are asymmetric; people tend to prefer pro-attitudinal information to a greater extent than they avoid counter-attitudinal information. Selective exposure can also be overridden by other factors such as social cues. In addition, behavioral data shows that tendencies toward selective exposure do not translate into real-world outcomes as often as public discussion would suggest.
— Andrew Guess et al., Avoiding the echo chamber about echo chambers (2018)
The story is complicated by the fact that those with highly partisan political views seem to be more likely to engage with content from the opposite side than moderates.
One study found that people who visited sites with white supremacist content were “twice as likely as visitors to Yahoo! News to visit nytimes.com in the same month”. As Bruns suggests, “they must monitor what they hate”. Meta-analytic findings similarly suggest that the more confident an individual is in their belief, attitude or behavior, the more exposure they have to challenging information.
— Peter Dahlgren, A Critical Review of Filter Bubbles and a Comparison with Selective Exposure (2021)
These findings appear to undermine the hypothesis that what is thought has a strong influence over what is engaged with. However, high-level analysis of this sort might obscure what is really happening. For example, there is some evidence to suggest that when, say, a committed conservative reads the New York Times, they are selectively reading those articles that are relatively consistent with their worldview. Alternatively, there might be framing effects: when a conservative engages with a New York Times article, they may arrive at that URL via a dismissive post from a fellow partisan, which changes how they interpret it. Finally, while selective exposure may be weak on average, it may be stronger for certain subgroups of people. It would be helpful for future research to account for these subtleties.
Assembling a feedback loop
Based on the above, we think that each of the three causal arrows that make up a feedback loop likely exists in some form, albeit with effect sizes that may be small, inconsistent, and context-dependent. Can we conclude, then, that a feedback loop exists? The way previous work has measured what is shown, what is thought, and what is engaged with varies, so it is difficult to assemble these causal segments into a closed loop.
The closest work we know of is a family of studies that use a framework called “reinforcing spirals” to study the interaction between selective exposure and media effects. However, most of this work focuses on traditional media (e.g. TV, radio, newspapers, or specific online news websites) rather than recommender systems, so it does not consider the link between what is engaged with and what is shown. It also tends to use only high-level, aggregate measures of what is engaged with (e.g. the number of days in a week that a particular news source was accessed). The best studies on recommender systems we know of, such as this one on YouTube, only consider one or two sides of the loop. One research group led by Damian Trilling at the University of Amsterdam appears to be thinking clearly about feedback loops, though they haven’t run any experiments yet. And this study by DeepMind researchers models all sides of the loop, but only in simulation.
Where next?
If you wanted to collect evidence for a complete feedback loop, you could design a research project something like this:
Say you suspect that a recommender on a video sharing platform is pushing users toward violent extremism. First, decide on how to measure and quantify three variables: what is engaged with, what is shown, and what is thought. To do this, build a classifier that quantifies the “extremity” of a given video. What is engaged with might then be the observed probability that a user watches at least 2 minutes of a video conditioned on the fact that it was offered to them, its position in the feed, and its extremity. What is shown could be the average extremity of videos recommended, and what is thought could be measured by a survey, such as the Sympathy for Violent Radicalization Scale.
Next, conduct three separate experiments, with real platform users, to assess whether these variables are causally related. To assess whether what is engaged with affects what is shown, artificially increase the engagement with extreme content above users’ true interaction patterns, and observe how this changes their recommendations. This could be done, for example, using a browser extension that engages with content in the background. To assess whether what is shown affects what is thought, artificially add videos to their feed that are more or less extreme than those they would have otherwise seen, and observe how this changes their sympathy for violent radicalization between pre- and post-surveys. (This raises some complex ethical issues; perhaps the videos shown in the experiment should only be less extreme than the status quo recommender output, never more.) Finally, to assess whether what is thought affects what is engaged with, monitor engagement for those same users, who will have been experimentally “de-extremized.” All these experiments should be run over a substantial period of time (perhaps 6 months) and with enough power to detect subgroup and cumulative effects.
This method, while not intended to be a gold standard, gives one concrete example of the kind of research that is needed. To demonstrate a complete feedback loop, we need to measure three variables consistently, and experimentally test the three relationships between them. A single study that takes this approach might only demonstrate a feedback loop of a particular sort in a particular context. But through a series of such studies, we might be able to develop a sense of the overall prevalence and effects of human-machine feedback loops in recommender systems.
Authors
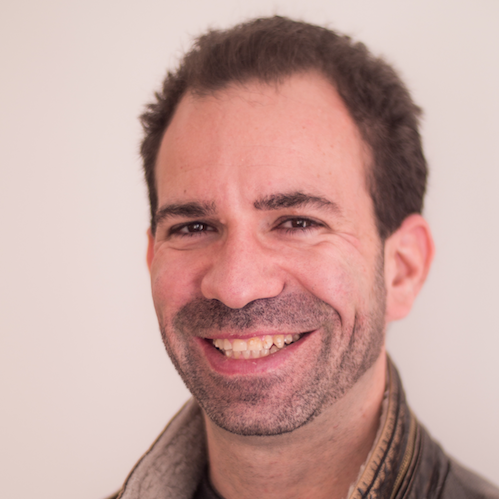
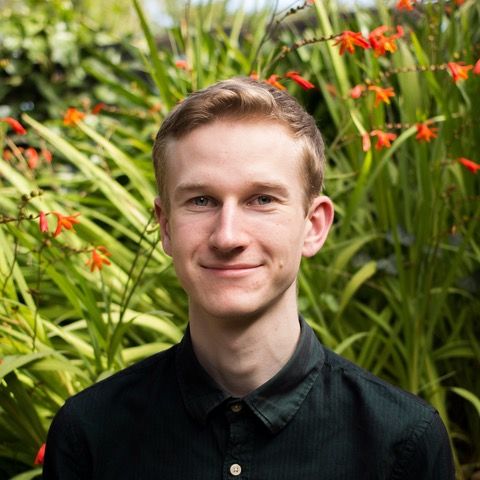
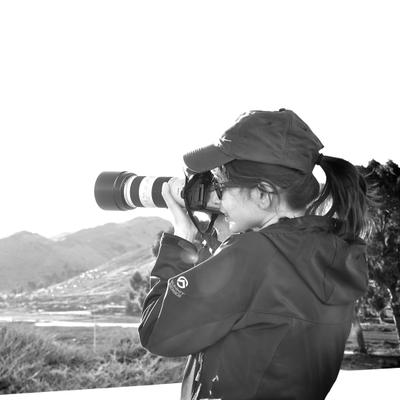