Future AI Progress Might Not Be Linear. Policymakers Should Be Prepared.
Anton Leicht / Aug 2, 2024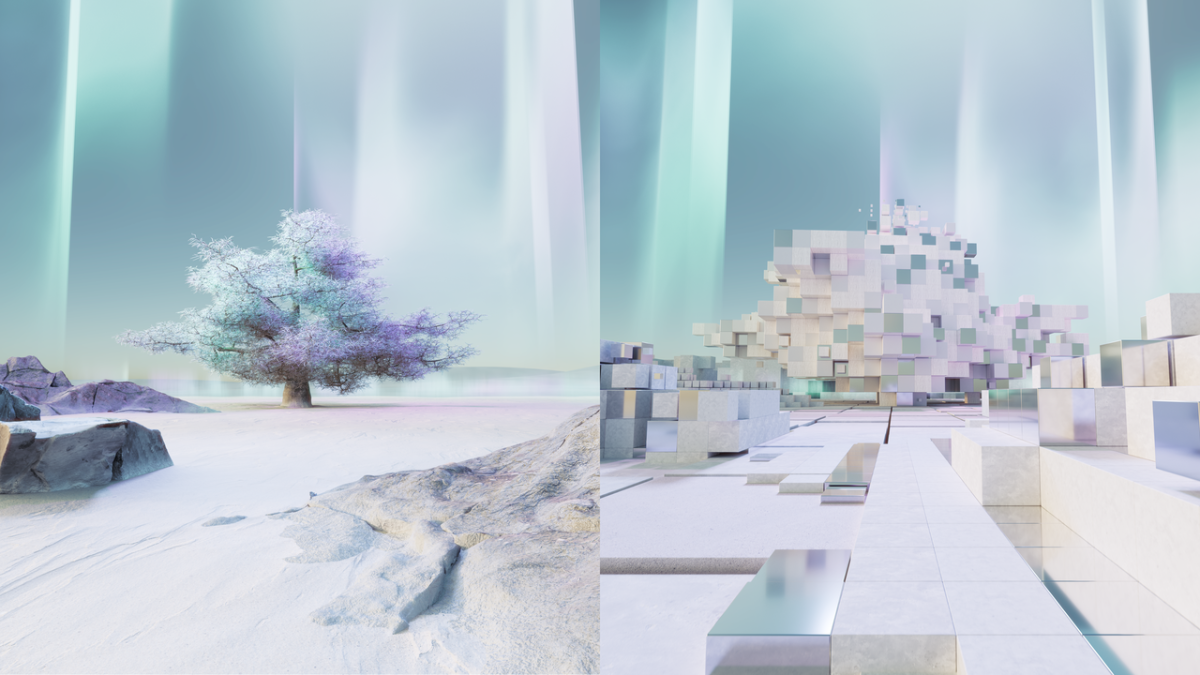
Linus Zoll & Google DeepMind / Better Images of AI / Generative Image models / CC-BY 4.0
Progress at the frontier of AI development over the last years has been remarkable, and seems sure to continue. Much has been said about the overall arc of this progress: increases in computing power and advances in algorithms will likely make AI models more and more capable.
This progress might not unfold as a smooth, linear increase of capabilities. Plateaus and even regressions – sometimes dramatically termed AI winters – have occurred before and might happen again. They could result from economic reasons: many emerging technologies undergo periods of initial excitement and accompanying investment, but if that fails to materialize in profits, funding might soon be scaled back. Frontier AI has not turned a substantial profit so far, but its demands on capital expenditure keep increasing: to keep up linear returns in capability, more and more expensive computing power must be provided. If significantly profitable applications do not arise soon, big funders, both venture capitalists and technology corporations, might reduce their investment.
Already today, market observers and funders alike are starting to ask questions about the profitability of current AI systems. Already today, funds are being redirected from capability advancement to efficiency and commodification. This motivates further uncertainty around the future of private sector AI R&D spending. Plateaus might also result from nonlinear technical progress: some researchers believe that the deep learning paradigm – or at least its concrete application to large language models (LLMs) –underpinning today’s frontier AI is not suitable for important future tasks, e.g. those that require higher agency. If the priorities in the AI research industry were to switch to a different technical approach instead, there would surely be a transitory shock to output in impactful breakthroughs and noticeable products. But whether a plateau is caused by technical or economic obstacles, it will likely be temporary. As long as some progress still happens, increases in compute and algorithmic efficiency would at some point likely revitalize interest and restore sufficient profitability to motivate further growth.
Progress Plateaus Motivate Complacency
Intermediary plateaus on the overall path of technological progress can be perilous for measured policy-making. On the one hand, intermittent lack of progress can lead to a sense of relief or even complacency among policymakers. With few salient instances of progress, novel harm pathways or substantial technological changes happening, political appetite for regulation may decrease, while pressure from industry to cut guardrails to boost innovation to end the plateau might increase. Furthermore, especially given the strong claims of outsized harm potential frequently made by advocates for restrictive AI regulation, there is a potential ‘boy-who-cried-wolf’ effect. Plateaus might be (mis)interpreted as falsifying past advocacy claims of rapidly increasing risks. This can lead policymakers to mistrust both the concrete policy suggestions that had been motivated by this advocacy – such as precautionary regulation – and to mistrust the advocates that had made these prognoses, even when they make new proposals. Plateaus are a perilous time for safety-focused regulation.
On Plateaus, Overhangs Mount
On the other hand, during plateaus, future risks can compound. One type of risk comes from an overhang of structural resources. Following stable progress trends, such as Moore’s Law, computing power is becoming cheaper and cheaper, and similarly, efforts to steadily increase energy supply and talent accumulation are underway. These trends may continue even on a plateau of technical progress, since they span across many industries and might be unaffected by AI-specific cycles. As a result, once a new paradigm is established, or once meaningful investment flows again, progress right after the plateau can be explosive, because it can suddenly leverage much greater structural resources. Imagine, for instance, that current LLMs had been developed at a time where compute was 100x more cost efficient. Capability progress and potentially adoption due to scaling would have been much faster, leaving much less time to assess and legislate on associated risks and harms. Similar stories could unfold with other novel paradigms.
Inversely, risks can compound from an overhang of adoption. Adoption of frontier AI, both via dedicated applications like chatbots and powering established services like Copilot, Apple Intelligence, customer service, and voice assistants, is only now picking up pace, almost two years after the release of OpenAI’s ChatGPT. As a result, early weaknesses in LLMs had little practical effect, mostly confined to the odd adversarial interaction with users of experimental chatbots. Likewise, outages wouldn’t be particularly critical because nobody depended on these systems, and data leakage wouldn’t be particularly concerning because nobody provided much relevant data to them. But assuming adoption and AI integration continues, a post-plateau generation of novel AI could be adopted much more rapidly – both technical structure and social acceptance would already be well-established. Growing pains and early vulnerabilities could have a much greater effect, then, with much less room for iteration in an early experimental stage.
Throughout Slumps, the Risk Landscape Evolves
Both of these issues are exacerbated by the fact that novel AI paradigms might come with different risk vectors: For instance, deep learning architectures raised substantial issues with transparency and scrutability of AI decision making that would not have been present in simple algorithmic decision-making; but on the other hand, deep learning systems do not seem to follow the kind of naive utility function maximization that underpinned some early concerns around existential risk. Sociotechnical approaches to mitigate harms and risks are often specific to these vectors – whether through work on interpretability, reinforcement learning on human feedback, or something else, they might not be easily transferable to different paradigms. On a linear pace of capability growth, policymakers and the public might have enough time to get used to these shifts. But if the low-attention environment of a plateau is followed by faster progress due to overhangs in adoption or resources, adaptation becomes a dangerous live-fire exercise.
In consequence, progress plateaus are a dangerous combination. They create a political environment uniquely unfavorable to caution, safety, and focus on harms; but they can predicate periods that would have uniquely warranted thorough preparation.
Policymaking Can Consider Plateaus
Policymakers and their advisors can act today to address that risk. Firstly, though it might be politically tempting, they should be mindful of overstating the likely progress and impact of current AI paradigms and systems. Linear extrapolations and quickfire predictions make for effective short-term political communication, but they carry substantial risk: If the next generation of language models is, in fact, not all that useful for bioterrorism; if they are not readily adopted to make discriminatory institutional decisions; or if LLM agents do not arrive in a few years, but we reach slowing progress or a momentary plateau instead, policymakers and the public will take note – and be skeptical of warnings in the future. If nonlinear progress is a realistic option, then policy advocacy on AI should proactively consider it: hedge on future predictions, conscientiously name the possibility of plateaus, and adjust policy proposals accordingly.
Secondly, the prospect of plateaus makes reactive and narrow policy-making much more difficult. Their risk is instead best addressed by focusing on building up capacity: equip regulators and enforcement with the expertise, access and tools they need to monitor the state of the field. If government offices are equipped with the ability to understand the progress happening beyond the hype cycle, to anticipate tomorrow’s AI paradigms, growth rates and harm vectors, they can navigate the perils of progress plateaus – much better than any static framework we could build today. Ideally, this is supported by a vibrant ecosystem of research organizations, advocacy nonprofits and evaluation businesses. Building capacity in that sense is robust policy – it hinges much less on one specific view of the future, but equips governments with the ability to address both prosaic progress within the current paradigms and the nonlinear paths with plateaus and setbacks this piece has laid out.
Conclusion
The path of progress towards more and more advanced AI could be convoluted and include plateaus and lulls. During these plateaus, public and political support for reducing AI harms might wane, but right after, risks might be particularly high. Policymakers and advocates should be mindful of that right now – by relying less on progress predictions and focusing more on building capacities to navigate future uncertainty.
Authors
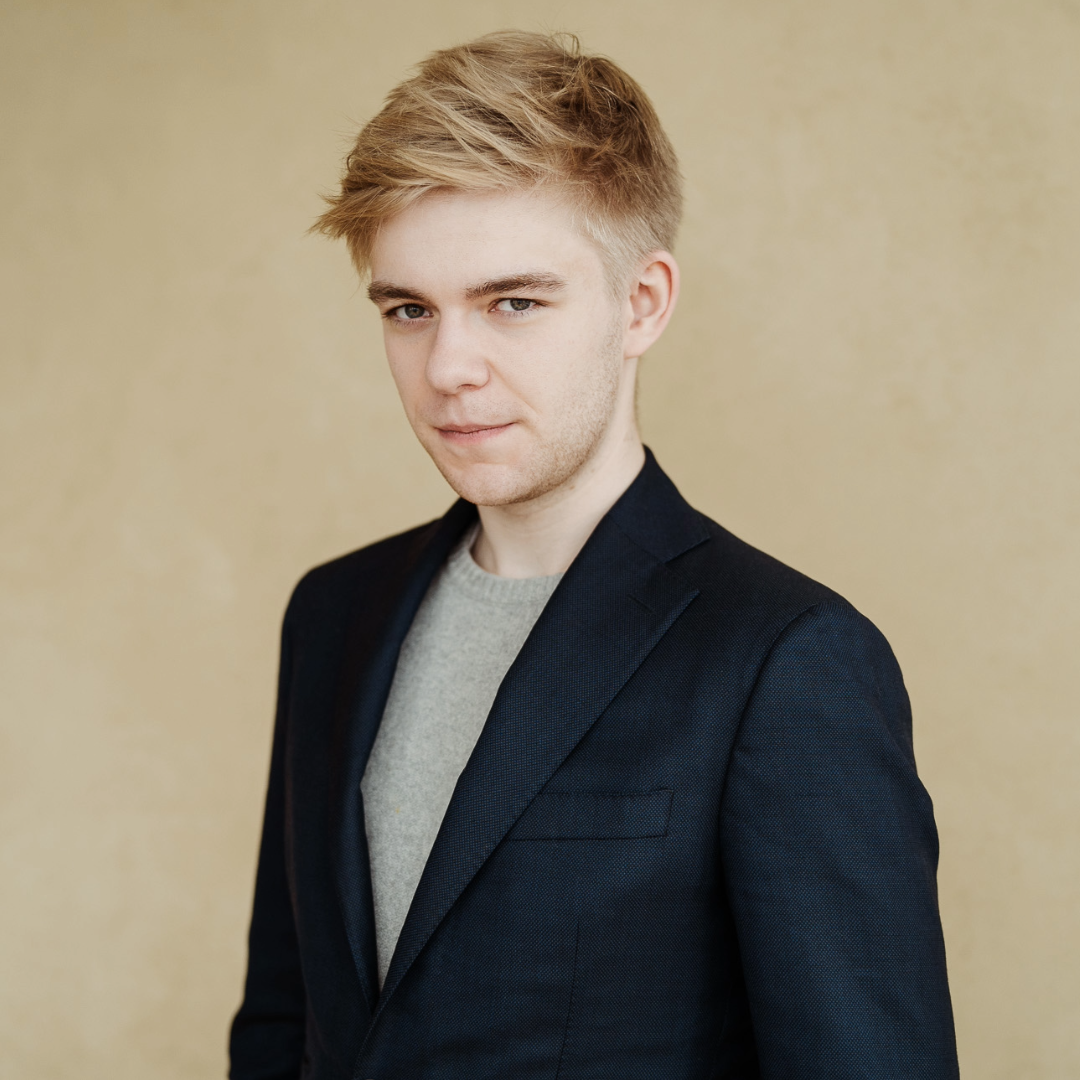