Gary Marcus Wants to Tame Silicon Valley
Justin Hendrix / Sep 22, 2024Audio of this conversation is available via your favorite podcast service.
In this new book, the cognitive scientist, entrepreneur, and author Gary Marcus writes that the companies developing artificial intelligence systems want the citizens of democracies “to absorb all the negative externalities” that might arise from their products, “such as the damage to democracy from Generative AI–produced misinformation, or cybercrime and kidnapping schemes using deepfaked voice clones—without them paying a nickel.” And, he says, we need to fight back. The book is called Taming Silicon Valley: How We Can Ensure That AI Works for Us, published by MIT Technology Press on September 17, 2024. I caught up with him just before the publication date.
A transcript of the conversation is forthcoming.
Justin Hendrix:
Gary, you are one of the most quoted people, I feel like, on conversations related to artificial intelligence. I think it's fair to characterize the valence of the kinds of quotes and the type of energy that's coming from you and all of the various communications channels that you operate as deeply concerned. This book is almost a way of drawing a line under many of those concerns and pointing perhaps to a way forward.
But I want to just tease apart a couple of things just at the outset. It seems to me with you, it's interesting because there's the ideas, and then there's also the people. You know a lot of the people at the top of the game of artificial intelligence, whether in the companies, in research communities, et cetera, which is one of the reasons why I found it interesting that you have a chapter in your book on the "moral descent of Silicon Valley."
I get the sense that you're not just telling a story in the abstract, as I might, about these questions, but you're also thinking about people you have met, have interacted with over time. What are you thinking about there? What is it that you feel like needs to be addressed when it comes to the moral character of this place?
Gary Marcus:
Yeah. I think that's an astute observation, first of all. Most of my work for a long time was just about the science, and I've become more interested in the politics and the people behind it. I remember meeting Larry Page not for the first time in 2014, talking about AI with him. Some of it's off the record, but ... Or maybe all of it's off the record, but I could hint at it.
Larry Page was one of the founders of Google, and their motto was Don't Be Evil. And I think he believed that. I think that he and I had some arguments, some of which we aired in the forum that we were in, but, fundamentally, I think he was interested in seeing a positive world. Although he was making tons of money, I think that he really did care. I think Don't Be Evil was a serious aspiration of his and of Sergey Brin's, who I've also talked to, but not quite as much.
Yes, I know a lot of the people who are running some of the big companies now and I've watched a lot of these things. I don't know all of them, but I know some of them. Of course, I appeared next to Sam Altman in the Senate. I've been watching what I see.
I think I grew up actually loving tech. I was a gadget head. I wanted to ... I mean I had a Commodore 64. It was the first thing I ever spent money on, I think, or significant money. I had PalmPilots. I wasn't the first adopter of the iPhone because I thought something else was better. But I was always interested in the latest gadget, noise-canceling headphones, before anybody else, and generally had a pretty positive view about tech.
There was always some deceitful people and so forth, but I generally had a pretty positive view about tech, and that really changed most sharply ... Although there were some precipitating incidents, but it most sharply changed when ChatGPT came out in November 2022 and was unexpectedly popular.
The technology itself wasn't really new. Those of us in the field knew you could build things of that sort and had some guesses what it would be like. They did a good job adding guardrails. They weren't perfect, but at least there was something as opposed to Galactica that had just been released. Suddenly it went wildly popular and suddenly dollar signs started flashing between people's eyes.
I would give you a contrast, which is in 2016, Microsoft released something called Tay, which was an early chatbot, and of course the first chat that was 1965. Tay quickly, because it was trained on data from humans and humans were messing with it, started spouting Nazi slogans and stuff like that. Within 12 hours, Microsoft took it down and said, "This is not really a great idea. We believe in responsible AI." Their president Brad Smith then wrote a whole book about responsible AI. They seemed to get it.
Then they have a stake in OpenAI. When OpenAI's chatbot took off, Microsoft changed how they were behaving, and radically changed how they were behaving. One example of that is when Sydney, which was powered by ChatGPT or GPT-4 or whatever, had this crazy conversation with Kevin Roose. Roose wrote about it in The New York Times, where it said that it was in love with him and asked him to get a divorce and all this stuff.
Instead of canceling that product like they did Tay, they put a few band-aids on it and rushed forward. Actually Brad Smith was on an episode of 60 Minutes that I was also on, and he was just like, "Oh, we've just solved those problems," and I knew that that was not fully true. Meanwhile, Satya Nadella started saying he wanted to make Google dance, to rush things along.
And so, there was a real shift as people started seeing how much money at least they imagined they could make, and we should talk about whether they really will make it. But people perceived there to be a lot of money to be made and suddenly they really dropped their commitment to responsible AI.
The AI that we have now is actually causing problems. It's like the days when big manufacturing plants would just dump their chemicals in the water and say society can take care of that. We have a lot of negative externalities, is the technical term, from generative AI, and the companies aren't paying for any of it. They're not really paying people whose intellectual property they're borrowing. They're starting to do a little bit of licensing.
OpenAI went so far as to tell the House of Lords, "We can't build our amazing software unless we get all this stuff for free," they more or less said that, which is not true because they could license it. But they're trying to do the biggest land grab in history of intellectual property.
This software discriminates against people. There was a study that just came out showing covert racism, where if you talk to these things in English as opposed to African American English, you get different results, for example. They're obviously being used for disinformation campaigns. Related tools are being used for deepfakes, et cetera.
Companies are not taking that much responsibility. They're basically expecting that societies will absorb that cost. That means, for example, teenage girls are now getting hassled by people that use nudify software to make fake nudes of them and distribute it, and it's not even clear there's any legal protection of that at all, or people get defamed. There was just a lawsuit about how some of Microsoft's Copilot did that.
There are all kinds of negative consequences and the companies are not bearing the cost. They're not doing that much about it. They're doing lip service, like they'll put in a million-dollar donation around disinformation. A $1 trillion company, $3 trillion company makes a million-dollar donation. That's a fig leaf, not a solution.
Justin Hendrix:
Gary, I assume that some of these individuals, where they on this podcast alongside you, they might say things like, "We take these things seriously as well. We go to Capitol Hill."
Gary Marcus:
I'm certain that they would.
Justin Hendrix:
"We're investing in these things. We've built consortia around problems of mis- and disinformation and doing our best to support legislative solutions around non-constitutional intimate imagery," et cetera. Why do you think we've got to a place where the industry's interests are so divorced from the scale of the harms or from the willingness, at least in this country, to address them? Is it just what you say, that it's just the scale of the opportunity and the amount of money they believe is at stake?
Gary Marcus:
That's half of it. The other half is they have no idea what to do about it. One popular thing to do is to talk about Terminator-like scenarios where AI takes over the world. I can't say those are impossible, but they're not our pressing concerns. It's good that some people in society investigate those things and so forth, but the way that's played is they make it sound like their things could destroy the world.
I think the worst culprit here might actually be Dario Amodei, who has literally gone on the record saying that AGI might kill us all in the next three years, and yet he has done nothing to slow down the race that might bring us towards that. When somebody does that, I don't know what his real beliefs are, but the impression that I get is that people talk about the doomsday scenarios as a way to distract from the fact that they're not really doing that much for the immediate pressing concerns. They don't really know how.
So I'll give you a couple of different examples. One is the copyright problem. So we know that these things are trained on lots of copyrighted materials, like novels and songs and so forth. One thing you could request but that they resist is transparency. What did you actually train on? If we had a manifest, then maybe we could figure out a way to compensate the artists and writers, but we don't have that.
Then there's a technical problem, which is what you would like, and you could imagine with some other software, but not with the technical stuff that they're actually building, would be attribution. So you'd say, "I came up with this in this way."
So when I as a scientist write an article, I can attribute sources. I can say this idea comes from so-and-so. But this software, generative AI software, can't actually do that.
I have this interesting paper with Reid Southern, which I mentioned in the book, in IEEE Spectrum earlier in the year, where we showed that you can get generative AI image production programs to plagiarize left-center, and they will do it even if you don't ask. So you say something like, "Draw me a picture of an Italian plumber," and out comes Mario, the Nintendo character. Real artists would never do that. They would think, "That's not a very good idea. I will lose my job if I don't look very original." These AI systems don't think like that and they just spit out Mario.
But what they can do is to say that they're spitting out Mario. They can't say how it is that they got there, because the way that they proceed is they explode all the information they get ... And it's a massive amount of information, it's the whole internet; it's effectively the whole internet ... and they break it up into little pieces. They reconstruct statistically probable assemblies of those pieces, but they don't know where the pieces are from anymore.
So they literally cannot at this moment attribute where things come from. They can't tell you that you got this, which also, by the way, puts the user in trouble because the user ... If it's Mario, the user's going to know Mario. But if it's some lesser-known photographer that the user is ripping off, they may not even know that. If the photographer gets wind of it, they can sue the user. The user hasn't asked to have that happen, but that's what the software tends to do. If you know the metaphor of attractors, they tend to be attracted to popular materials. So they can't solve that problem.
Another example is discrimination. People are using this software now to judge job applications. It's almost certain just from what we know about the nature of these systems and similar systems that they're discriminatory. They're probably discriminatory against minority groups and women and so forth. There's lots of evidence that might be true, but there are no laws in place to allow us to audit what they're actually doing, and the companies aren't actually doing anything about that. It's not like they're writing checks to people who lost their chance at a job because they didn't meet the computer's skewed notion of reality.
The concrete example there is if you write down that you're a ballet dancer, they're probably not going to recommend that you be a computer programmer because, historically, ballet dancers weren't programmers. Now they often are, but you have ... What these systems do is they perpetuate past history. They don't understand human values and so forth.
So they don't know how to solve those kinds of problems. They don't know how to solve them. They don't know how to solve the long-term problems either, but they're not here, so it's not as an obvious thing. So they deflect to these long-term problems about what if AI kills us all, which can't be measured, don't have a tangible consequence right now. Again, they're worth considering, but these other problems are here now, and they can't do anything about it. They distract away from it.
So to go back to your question, some of it is, yeah, they make more money if they don't deal with this. Some of it is the technology that we have right now is what we call black box. We don't know how to look inside it to know how it's doing what it's doing, and that itself is really hard to address these problems, and so they don't. Then they just leave it for the rest of us to clean up the mess.
Justin Hendrix:
Another thing that I feel like is bound up in a lot of your assessment of problems with today's Silicon Valley and with today's AI, which is I guess the direction that we've gone in with regard to this particular strain of AI systems and the enormous amount of capital that's now been deployed to commercialize and deploy generative AI in particular as opposed to the alternative technical directions that you appear to believe will take us further when it comes to delivering on the promise of our artificial intelligence as you see it.
So I don't know. I want to just ask you to just unpack that a little bit for the listener. That seems core to your diagnosis about moral descent essentially, that this is a get-rich-quick scheme in many ways that's based on a kind of scam technology.
Gary Marcus:
It's not quite a scam, but people are scamming with it. The technology really does a great job of statistically analyzing the world and approximating things, but approximating things is not really understanding them. What Silicon Valley has done is to hype this stuff no end to make it sound like it's magic, and it's not.
For most of 2023, they had the entire world going on that premise, that this is artificial general intelligence, or it's not very far away. We still have people trying to convince the world of that. Elon Musk said that we would have artificial general intelligence by the end of 2025. I think if you look at what we actually have right now, that's crazy idea. And so, I offered him a million-dollar bet and he didn't respond. A friend upped it to $10 million, he still didn't respond. Even for him, that's real money.
The Valley has learned that if you promise great things, nobody ever holds you account. If you say you're going to make a fleet of a million driverless cars by the end of 2020, as Elon Musk does, and then it's the end of 2024 and you still haven't, people still don't really hold it against you.
Issuing promises has become free currency that drives stock valuations. It's worked for OpenAI, it's worked for ... I think it's worked for Anthropic, it's worked for Tesla, and so forth. And so, they keep doing it. If nobody's going to hold them account, why not?
Just yesterday, like Masa said, everything's going to be a hundred ... Or the day before, Masa Son said everything's going to be a hundred times smarter than humans soon or whatever. People just have learned that you can play the game this way. So that's one piece of it.
Another piece of it is, yeah, these tools are actually technically inadequate. Maybe the simplest metaphor for people without a strong technical background is to think about what Daniel Kahneman talked about a system one and system two cognition. So system one is things you do by reflex, statistically automatic, and system two is more deliberative reasoning, analysis, and so forth.
The right way to think about what we have now is it's good at system one. It does things automatically and quickly. But if you change from what you've been trained on, you really need to reason over it, these systems fall apart. Like my favorite example right now are these things called river crossing problems. They're things like a man and a woman and a goat and some cabbage need to get to the other side of a river. They have a boat. You can't let the goat stay with the cabbage. You've probably seen word puzzles like this when you were a kid. There are a bunch of them on the internet, which means they're in the training set.
If you give exactly the problem that's on the training set, it will get it right and you'll be like, "Oh my God, this chatbot is so smart. It figured out that I can't leave the cabbage and blah, blah, blah." But if you change the problem, it can't really reason about it. S.
O my favorite example of this which Doug Hofstadter sent me is a man and a woman are on one side of a river with a boat, and they need to get to the other side. What should you do? The system comes up with a cockamamie scheme like the man goes across the river with the boat, leaves the boat, swims back, somehow gets the boat back, and then takes the woman across.
If you actually understood what this is about, you would never say something that insane. In fact, I told my 10-year-old and she's, "Yeah, you just put the man and the woman in the boat and you go across the river. What's the problem?"
So they don't have the level of reasoning that my 10-year-old has, not even close. To even compare them is very insulting to my 10-year-old because she can actually reason about this.
So these systems just don't reason very well. They don't plan very well. And what's being sold is hope, which is a variation on the word hype, which is we'll just add more data and we'll solve these problems. But the reality is we had a big advance between 2020 and 2022, and then GPT-4 was trained in August of 2022. They're released later. We're now over two years later and nothing has significantly solved these problems, a reasoning or the other common problem is hallucination. One of these systems said, "I have a pet chicken named Henrietta." It just made it up. The systems can't actually fact check. They can't go out to Wikipedia to see does Gary have a pet chicken named Henrietta, or do a web search or whatever.
So you have these systems that can't actually reason, and people are pretending some kind of magic will change that. So you're asking a question about the intersection between my politics and political concerns, policy concerns, and my academic interest. It turns out my dissertation was with Steven Pinker, and it was on children learning past tense of English. Why do they sometimes make mistakes? They'll say things like goed instead of went as the past tense of English. That was really what my dissertation was about. But it was also about neural networks, which is a predecessor of today's chatbots, and how they learn things and why it was different from people.
So for 30 years, I've been thinking about when machines can generalize, machines of certain sorts, and when they can't and what the consequences are. And so, I predicted the hallucination errors in 2001 in my book, The Algebraic Mind. I've been thinking about the technical side for a long time and the limitations of just using a big statistical database as a proxy for everything else.
That's really what these systems do. They use statistics as a proxy. Some of the time it works and some of the time you get these crazy answers, and nobody actually has a solution to it. It's just the industry keeps saying, "Oh yeah, we'll solve that in the next few months," and then they never do. They just keep issuing this currency.
And so, the intersection is like this thing that's being wildly oversold from a technical perspective is driving all of the money and power to a few people who know how to build it, even though they're not actually delivering it.
So why is Sam Altman one of the most powerful people on the planet? Is his company actually making money? No, they've never turned a profit. He is on all these boards with the White House and he's in TIME Magazine every other day, et cetera. But his product doesn't actually work. It's fun to play with. It works in certain limited domains like brainstorming, but, in general, you need a human in the loop because it's not reliable.
He himself, eventually, after I had been seeing it for a year and a half, and his people attacked me, he eventually said, GPT-4 sucks. The stuff doesn't work, but it's all on promise that we're giving them immense power. Then what is he doing with that power? One example is he just invested in a webcam company. He also wants to have access to all your personal files.
So my guess is that OpenAI will not make the money from businesses that they thought, and instead they just hired Paul Nakasone, who used to be on the NSA, on their board, and that they're gearing up to be a massive surveillance company.
I should remind the listener, OpenAI is still legally a nonprofit for the public benefit. They're supposed to help humanity, and instead they're making money with a for-profit inside that I would guess is going to become a surveillance company. It almost boggles the mind. It's Orwellian. The name of the company is Orwellian, OpenAI, but they're not transparent about what data they use, they're not transparent what models they use, and they're about to take your data and sell it to maybe governments around the world or whatever they choose to do.
Justin Hendrix:
We've talked about the nonprofit governance issues around OpenAI on this podcast. In the past, I was able to have Robert Weissman from Public Citizen to talk about the letter that they sent, I believe, to the California attorney general on that matter. I believe at the time that you were also in favor of there being some consideration of that and the extent to which OpenAI should be considered and breached of its duties as a nonprofit.
Gary Marcus:
I mean obviously not ... Maybe obviously too strong. It seems very ... What's the right word? There is a lot of reason to think that they're no longer operating ... As Weissman and Public Citizen pointed out, no longer operating according to their charter. If you actually read the charter, which I've quoted a number of times in my Substack, it promises to make AI in the public benefit, for the interest of all humanity. That's just not really what the company is doing right now.
Another thing is I sometimes see people up in arms because some small nonprofit pays their people like a million dollars a year. People can't believe a nonprofit would do that. Almost everybody working for OpenAI, assuming all stock deals close and whatever, will be making tens or twenties, millions of dollars.
So they don't behave like we expect a nonprofit do. They're behaving like a for-profit company. Now their board, I think, is retrenching around Altman and not really living up to what their responsibility should be in terms of the public interest.
Justin Hendrix:
I want to just push you, though, a little bit on that part of my question earlier that was about which direction you think technically this field should be moving in. What do you think could solve these issues?
Gary Marcus:
I hinted at that, but I'll spell out a little bit more. So go back to Kahneman's system one and system two. The system two is about reasoning, really about explicit knowledge, facts that you know or you can read in an encyclopedia or learn about in the world. You can think about the process of fact-checking as an example of reasoning, where you reason from the known facts and figure out if the unknown facts might be consistent with them and so forth. A lot of that is explicit symbolic knowledge, things you actually read. I'll give you one example.
In my TED Talk, I give an example where Galactica, which was a chatbot that came out just before ChatGPT, from Meta, said on March 18th, 2018 Elon Musk was involved in a fatal car collision. It wasn't true. The system just made it up. It's what we now call hallucinations.
You could say, why does it do that and what would a good system do? A good system should look at each assertion that a thing makes and say, is that a reasonable assertion? Is there any evidence, first of all, that he was in a fatal car accident? If you read the rest of Galactica's story, it says he died there.
Let's consider that fact. Could Elon Musk have died in March 2018? What is the evidence that he didn't? Well, there's evidence literally every single day because he posts on Twitter, X every single day, and he's in the news every single day. The evidence that he's still alive is overwhelming.
You want an AI that can evaluate that evidence, that can look on Wikipedia, can look in the news, that can reason about what it's talking about. That's what we need to move ahead. More specifically, I guess, we need an integration really between two traditions in AI that are very old, but have really been very distant from one another.
So one tradition is the neural network tradition that is powering chatbots like ChatGPT and the other tradition is classic symbolic AI, which looks a lot like computer programs and does more of the stuff that I'm describing. Somehow we need to bring them together because they're almost complementary in their abilities, but sociologically, diametrically opposed, and sociology has been devastating here.
On the one hand, you have people like Geoff Hinton who hate the symbolic tradition, even though, ironically, a lot of it was invented by his great-grandfather, maybe not coincidentally. So he's always pushing against his great-grandfather's tradition, saying we can do it with these systems that learn very well. And they can, but they don't reason as well as the symbolic systems. The symbolic systems reason pretty well, though they could be improved, but they don't learn very well from large amounts of data.
It's just screamingly obvious that we need to bring these worlds together, but venture capital doesn't want to do that and the big companies don't necessarily want to do that. You have to think about how venture capital works, too. What they really want is a plausible proposition, doesn't really have to work, that making something really big will make a lot of money, so that they can sell it to their LPs.
If you get 2% of a billion-dollar investment, you're doing really well whether the investment comes true or not. And so, the venture capitalists are all backing essentially the same experiment right now, and that experiment is make these neural networks that we're using right now bigger. Ilya Sutskever's company's got a billion dollars with at least no public results. Many of these things are funded for billions dollars.
If you look at what happened over the last two years, probably about $50 billion went into this idea of scaling, that you can make AI work or make artificial general intelligence simply by having more data and more GPUs, which is why the price of Nvidia, at least for a while, was going up. I think as we're recording this, it's actually going back down because people are saying, "How does this actually make money since it's not reliable?"
But put that aside, the venture capitalists have gone all in on the scaling hypothesis, and so have the big companies. Since almost all other research doesn't get the funding that it deserves, all the talent goes in this one place. When there are massive bets, it becomes extremely hard for anybody to compete with an alternative idea.
And so, there are times historically where science has just been screwed up for whatever reason, and this, I think, is one of them. I'll give you another example.
In the early 1920s, everybody thought ... Or throughout the '20s, everybody thought that genes were made up of some kind of protein. And so, they all ran around trying to figure out which protein was the molecular basis of heredity. Turns out they were wrong. It's actually a sticky acid, the double-helix, DNA. People just spent tons of money and energy pursuing this one hypothesis. It was hard to get people to look at anything else.
Right now it's hard to get anybody to look at anything besides scaling. In fact, I've been watching AI for a long time, since I was eight years old, and I've never seen the intellectual monoculture that we see right now, and it's driven mostly by money. Money has this reinforcement feedback cycle, so of course a billion-dollar model is going to outcompete a $1 million model. And so, people put more money in the billion-dollar models.
But I think it's the wrong bet, and I'm willing to bet that in five years, people will say, "What were they thinking?" Of course that wasn't all you needed. You needed something else. We've wasted most of the 2020s going down this one path.
Justin Hendrix:
I want to just make sure that the way I understand a lot of your policy ideas is consistent with the way you think about it, which is you're talking about very straightforward things that I think every listener to Tech Policy Press's podcast would agree are important things, like ensuring data privacy, addressing liability issues, making sure there's independent oversight. You talk a bit about how we need layers of governance, that kind of thing.
It's not just as I understand it, because we need to address the harms of the technology. We need to steer these companies away from certain practices that are clearly bad for people or bad for society or bad for democracy. But it's also your view that these types of constraints would direct the science in a better direction and give us better AI.
Gary Marcus:
That's right. I tried to have the shortest list I could of things we ought to be doing policy-wise, and I think I came up with 11 or something like that. I wish it could be one. It would be much easier to talk about it in podcasts if I said, "The one thing that we should do is demand transparency in AI," which we certainly should do, or, "The one thing that we should do is to demand that people test the risks and benefits of their systems before they release them widely," which, in fact, we should do. But in fact there are many things.
But some of the ones that I talked about are definitely geared towards making a better form of AI. The form of AI we have right now just lends itself to abuse. In fact, because it's so bad at truthiness, or factuality I should say, it's so bad at factuality, it gets misused for misinformation and for phishing, cybercrime, et cetera, et cetera. So there's a particular form of AI that we have. I think we will look back in a few years and say, "Man, that was not really a hot idea," either on the technical side, but also in terms of applications.
Just today I saw ... It looks like someone was committing fraud in Spotify and streaming services and so forth by making basically fake music, and then trying to get royalties for having bots listen to those music. The music was probably made by generative AI. So there's all this stuff that's going on that's negative.
Other forms of AI might be able to constrain themselves in different ways, but how do we get those? So at least a few of my suggestions are geared to that. One of them, the most direct one, is just I think the government should get back involved in research in AI.
So, historically, the US government has sponsored research in medicine. It's sponsored research in aviation and aeronautics and so forth. There are some investments right now, but they are dwarfed by industry, and industry is betting on this one form of AI that's problematic. Why don't we have the government bet on other forms of AI that might be more controllable, might be safer, might not have these downsides, and, by the way, don't lead to the same kind of weaponization of surveillance capitalism?
And so, I think there's a lot of opportunity for the government to do better, and I would very much like to see that. Then there are other indirect things like I talk about tax incentives. If the tax incentives were for building AI that is pro-social rather than AI that is anti-social, that might actually move the companies in what they did.
Justin Hendrix:
Maybe there's not one single thing, as you say, that you'd like to see done, but there is, you say, in your view, "the single best thing that Congress could do", which would be to create an enduring and empowered agency that is nimble enough to keep up, positively about ideas from folks like the Georgetown scholar Mark MacCarthy, who's also written for Tech Policy Press about this idea of ultimately empowering some kind of singular digital agency, which would be good at keeping up with this pace of technological development. Why do you think Congress needs this or why do you think the federal government needs a singular agency?
Gary Marcus:
So, first of all, I don't think that they'll do it or they won't do it anytime soon. There are some other countries that I think will be ahead of us in this, and I think we will suffer for it. Politically, it's a very challenging thing because it steps into turf of lots of different existing agencies and it's also a lot of work. So standing up Homeland Security was difficult. A lot of people didn't enjoy the process, shall we say, but I think we really should do it.
One issue is simply the speed at which the technology is changing. 2024 is definitely different from 2020, and 2026 is probably going to be pretty different from 2024. So one option, and I actually talked about this with Senator Durbin when I gave testimony a year and a half ago ... I can't tell you how often, by the way, I used the transcript that you wrote of that testimony. I used it many times in writing the book.
So Durbin and I talked about this, and basically he said, "It's not the job of the Senate to make individual policies about individual pieces of technology. They're too slow to do it." I'm putting words in his mouth. He didn't say it exactly that way. But they understand in the Senate that they can't be making a law like if GPT-6 is different from GPT-5 or GPT-5 is different from GPT-4 or some other technology. They're just way too slow to do that. And so, you need an agency.
Imagine if we did this for medicine. When Wegovy comes out, should you have senators pouring over the charts to see if Wegovy is safe? That doesn't make sense. That's not the training that senators have. So we have an FDA agency where people do actually understand medicine and hire outsiders and do what they need to do in order to make rational decisions about what is good for the public and what is not good for the public. They can act much more quickly, much more nimbly than the Senate or the House of Representatives. Even the executive office has some limits on it. And so, you want the expertise.
It's obvious that we need this for AI because AI is affecting every facet of life, and you have hucksters in every domain, so people selling educational software that doesn't work, or whatever, and you have possible good applications. Maybe someone will come along with an educational innovation that will actually fundamentally change education. You want to be able to seize that here and not have the US be like last of the party.
And so, both to exploit the positive uses of AI, which we haven't talked about much today, but I genuinely believe exists, or at least potentially exists, and to deal with all of the risks, I think you need an agency.
The alternative that we have right now I would call muddling through. We have a bunch of agencies doing the best they can. So, for example, Lina Khan in the FTC is doing a fabulous job of taking the existing powers of the FTC and trying to fight against fraud in various forms and so forth. I think she's doing an amazing job.
Wanting an agency is not saying that I don't think the FTC, for example, is doing well, but it's saying the existing laws don't really cover a lot of what we need to cover. Another example would be defamation. It's not even clear that we have an existing law that covers defamation by an artificial machine as opposed to a human, because we usually talk about intent, and the machines don't have intent, but they can still screw up people's lives.
There's just lots of gaps in the existing law. We need somebody whose job it is and who has the personnel to support them that looks full time at this rapidly changing state of play and can keep up. It's just not realistic to assume that 10 people in 10 different agencies who pay a little bit of attention to this and are smart people are really going to be able to handle that.
Justin Hendrix:
Just to emphasize again for the listener, it seems like this project, this book, the things that you're up to these days, they're all about basically steering oxygen and capital away from the current approach to artificial intelligence and the current vehicles that are leading that approach and trying to create room for something different.
I want to point out in the epilogue, you actually give various ways that you think even individuals can get involved in this. But one particular one that stood out to me, this idea that we should all boycott generative AI companies and tools that do not provide remuneration and protect copyright of writers and artists and musicians and others who produce intellectual property. Is that an option these days? Is there a company that you would point to and say, "This is the ethical one"?
Gary Marcus:
There are some small companies. None of the big companies are really being ethical here, but I think we could force their hand as citizens. We could say, even though these smaller companies that make generative images don't have the same quality of product, I just don't want to be part of this. I don't want to facilitate the mass theft of intellectual property from artists and writers, so I'm going to use this other stuff, or I'll just wait.
Honestly, chatbots are super fun to play with, but how many people really need them? A lot of the surveys that I've seen are that programmers use them for autocomplete and people use them to brainstorm, but a lot of people in the end find them more work than not.
What if we all sat down and said, "Look, we love what your generative AI is trying to do. We can see that this is going to be worth a lot of money. We're eventually going to give you subscriptions. We're excited. But we want it to be better first. Get your house in order. Make sure your stuff is not discriminating wildly against people. Make sure it is not ripping off artists. When you get your licensing stuff in order and you make sure the artists and writers and so forth are paid for, and when you deal with these discrimination issues, covert discrimination, covert racism, and stuff like that, sure, then we'll come use your software. But in the meantime, we don't have to have it."
Honestly, my life would be harder without my cellphone, and it would be a lot harder without electricity. But I don't use generative AI, and I'm fine. I didn't use it to write the book. I can live my life without it. We could all wait until it was better. We could say, "Make a better product and we'll come back to you."
Imagine if we all went in airplanes that just suck, and there was no regulation and people just died every day. People just wouldn't do that. They would say, "Airplanes are cool, but come back to us when you know how to make them work reliably." That's not an unreasonable ask.
Justin Hendrix:
I think part of it is this issue of how we recognize the harm. I think of generative AI as original sin. This idea that it's hoovered up all of the world's knowledge, copyrighted material, and the rest of it, and a lot of folks appear to be willing to just say the sin is there. There's not much we can do. These products are staying. But they're still so valuable that perhaps some good will come from their application, and so we should just carry on. Are you suggesting that, no, we should stop at original sin?
Gary Marcus:
I think the long-term outcome for society would actually be better if we did that. I'm not king and I don't think I'm going to get enough people on board realistically, but much better AI than we have now is possible, and the incentive isn't really there to make it right now.
Now, mind you, the current AI is not actually making that much money. OpenAI has never made a profit, for example, but we're giving all of this power to them on the ... I won't say fantasy, but the promissory note that someday they might make a lot of money.
We don't have to use this stuff. If we didn't, I think in the end, we would get a better AI [inaudible 00:40:35]. It'd be like, if you knew that seat belts and airbags were possible, you could still drive other cars, but you could say, "I'd actually like to wait for the safer cars."
Now some of this is complicated because the harms can be distributed not just to the user, but to society. So you use these things and you're hurting artists, you're hurting writers. There's an argument that it's just unethical to use this current breed of software because it is being used for disinformation that's going to undermine elections. Maybe that won't hurt you individually, but it will hurt your nation.
It is being used for cybercrime. Maybe you won't get ripped off, but probably some of your neighbors will. It's being used for kidnapping schemes, where people pretend to have kidnapped somebody and get ransoms. It is being used for discrimination and job hiring.
There's a moral cost to it. There is a moral cost to using things that are made by unfairly compensated laborers. There is a moral cost to using generative AI right now. I think it's actually pretty high.
So you have that on the one hand. On the other hand, I think the theoretical and very reasonable possibility that we could build better AI, and we are not putting any pressure on those companies to really do better. We're saying, "Fine, go make these chatbots that hallucinate. We love them. Knock yourself out," instead of saying, "Come back with a chatbot that doesn't hallucinate, that isn't going to defame people, that I can trust."
Justin Hendrix:
Gary, you end this book by saying, "The bottom line is this: if we can push the big tech companies towards safe and responsible AI that respects our privacy and that is transparent, we can avoid the mistakes of social media and make AI a net benefit to society rather than a parasite that slowly sucks away our humanity."
You seem to be, even in this conversation, slightly hedging on whether we're going to be able to achieve that. I don't know. What do you think are the time horizons here? How long do you think it might take to turn the ship in the way that you want to turn it?
Gary Marcus:
If we could get everybody on board ... For example, the book does very well and there's a lot of conversations ... I think we could act pretty quickly. I'm not super optimistic. I didn't write this assuming that I would succeed in this battle, but I think it's an important battle. If everybody just stopped using generative AI for six months, that would have an amazing consequence. Because so much of this is driven by money and estimates and so forth, I think that would actually drive a lot of companies to do better.
If people could get their congresspeople and so forth to realize the tech policy matters to them as much as immigration policy and economic policy and so forth, then we could get legislation that would protect us from some of the downside risks. Right now the EU actually has some legislation, and American citizens are sitting ducks. We just have no protection to speak, or very little protection, from any of the downside risks of these things. If enough people got exercised, we could change this. That's why I wrote the book.
Justin Hendrix:
Okay, Marcus. Thank you very much for taking the time to speak to me about this today. Thank you again for taking the time.
Gary Marcus:
Thanks for having me.
Authors
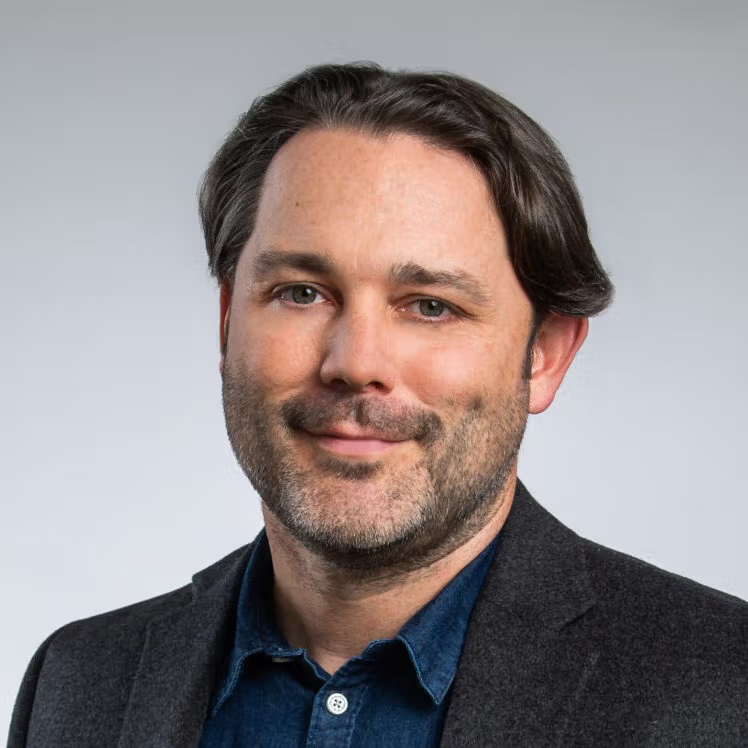