Measuring and Understanding Amplification on Social Media Platforms
Marlena Wisniak, Luca Belli / Aug 19, 2024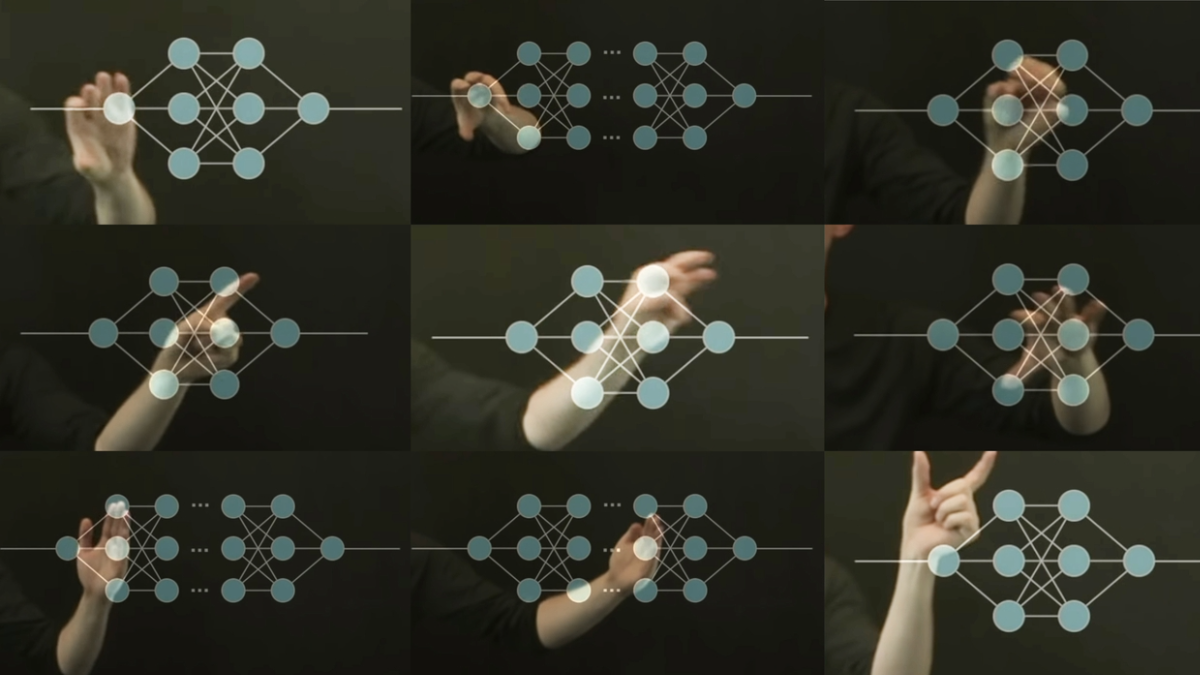
Alexa Steinbrück / Better Images of AI / Explainable AI / CC-BY 4.0
Generative AI is today’s big, flashy technology. Surely, there've been a number of impressive breakthroughs this past year alone, generating lots of press coverage—and let’s be real: hype. From “generative AI can solve all your problems” to doom and gloom scenarios, narratives around the opportunities and risks of generative AI are mostly grounded in speculation.
Unfortunately, this fascination comes at the expense of less attention-grabbing, yet critical, AI systems that are ubiquitous in everyday life and the cause of significant harm in the present. For example, we collectively spend an estimated 12 billion hours per day on social media platforms, which are powered by recommender systems to increase user engagement and time spent on platforms. Even the question of potential harms from generative AI is largely one of recommender systems. For example, manipulated and deceptive content becomes a critical issue for elections, democracy, and news integrity when it’s widely spread.
While recommender systems are an example of a (relatively) old class of AI systems that significantly impact our daily life, they are still poorly understood even by so-called experts. Recommender systems are embedded in a variety of applications, from social media feeds and movie recommendations, to search engines and job boards. While the basic idea is simple—ranking new content based on past behavior—they are trickier to study than other AI systems, such as classification models. Assessing whether a recommender system is working as expected or not is challenging given the presence of undefined concepts, such as the value for users or their preferences. Indeed, a system that might seem to work well for one person might have detrimental effects for someone else.
Emerging regulation, such as the Digital Services Act (DSA) in the European Union, acknowledges the importance of requiring more transparency from online platforms. The DSA focuses on content moderation, where platforms and search engines remove content that they deem illegal or violating their terms of service. As important as content moderation is, it’s not the only process that shapes what content users see. Much of this content is instead enabled by recommender systems, which are the basis for promoting and recommending content in our feeds. It’s also why some content gets boosted, a process commonly known as amplification.
To better understand how social media platforms function, we need to understand, measure, and track algorithmic amplification. While there’s currently no agreed-upon definition of amplification, it’s generally understood as the extra exposure that a recommender system provides to a piece of content. Focusing on amplification can help assess the broad range of societal and human rights implications of algorithmic content moderation. These are broader than bias and discrimination, which have been the focus of Responsible AI practitioners.
We propose introducing standardized measures and metrics to improve tracking and measuring algorithmic amplification. Inspired by regulation in the food industry, we call these metrics ‘nutrition labels.’ Such labels can inform users on what content they see, and why, including what kind of content gets recommended more. Because of the real-time nature of social media platforms, a static number would not be very useful. This is especially true when monitoring critical and fast-evolving events such as elections, wars, natural disasters, or other crises. We envision nutrition labels as dynamic dashboards, where algorithmic amplification measures are updated almost instantaneously.
Aiming to spark an inclusive conversation on how to measure amplification—with participation from civil society, academia, policymakers, international organizations, and the private sector—we offer a couple suggestions for what these measures could look like. First, platforms should report on the creators who receive the most exposure, relative to the size of their following base. Second, they should report on the share of exposure creators receive from direct followers, as opposed to other users who are not explicitly following them. It’s not technically possible to measure amplification for all accounts on a platform, and it would likely come with dangerous privacy implications. That’s why, in our research, we suggest narrowing the scope of groups and accounts of interest, centering on public figures as those are the ones with the highest risks for human rights and democracy.
Understanding algorithmic amplification is necessary to explore the processes and tools that empower users, especially marginalized groups who are most at risk of harm, so that they can proactively shape and assess the content they engage with. Nutrition labels are surely no silver bullet. There are many challenges in implementing and operationalizing them, from insufficient digital literacy to conflicting business and profit incentives. Nonetheless, we believe that labels can be a helpful tool in the broader algorithmic transparency toolkit. Their potential warrants further exploration, together with civil society and affected communities, who should play a central role in designing them.
Authors
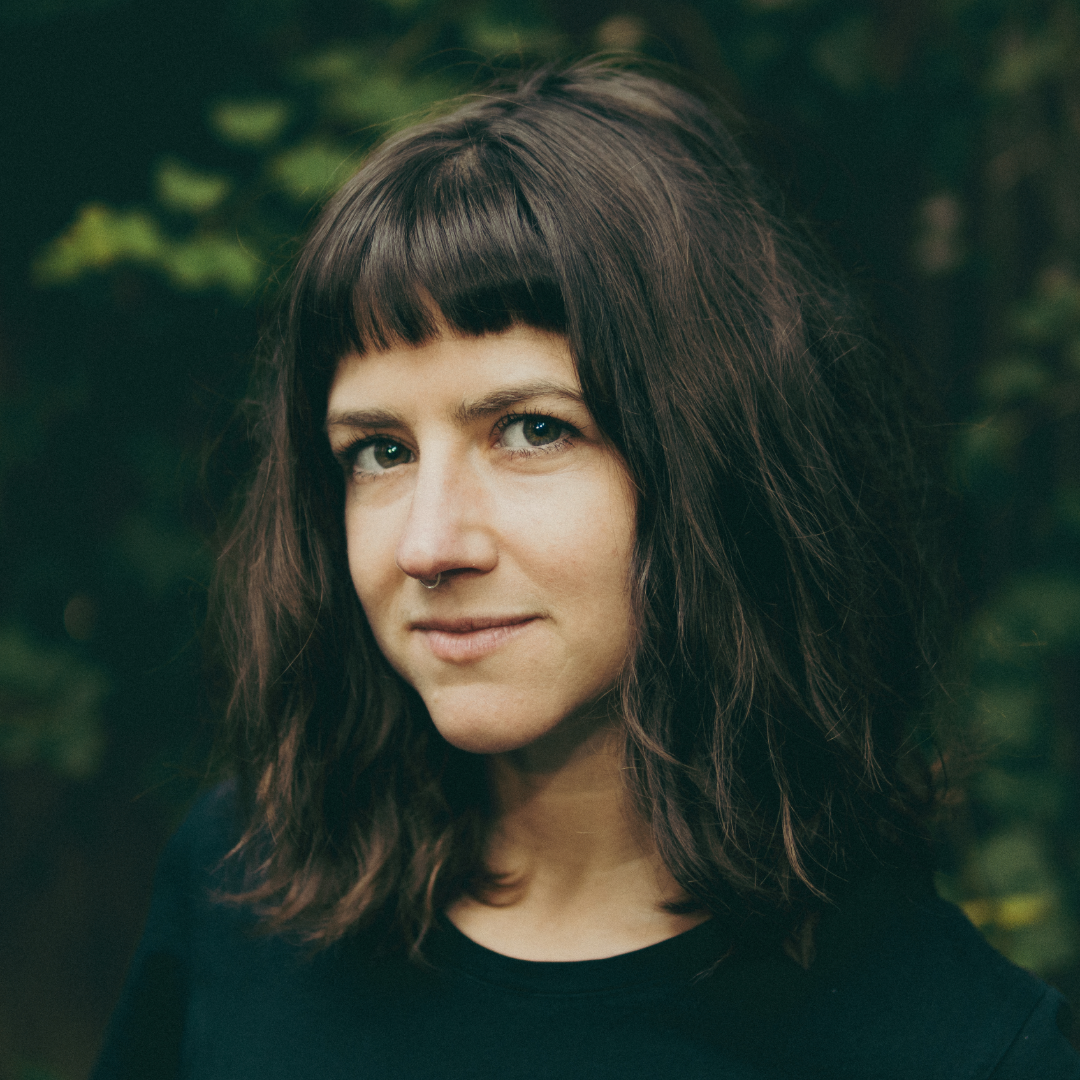
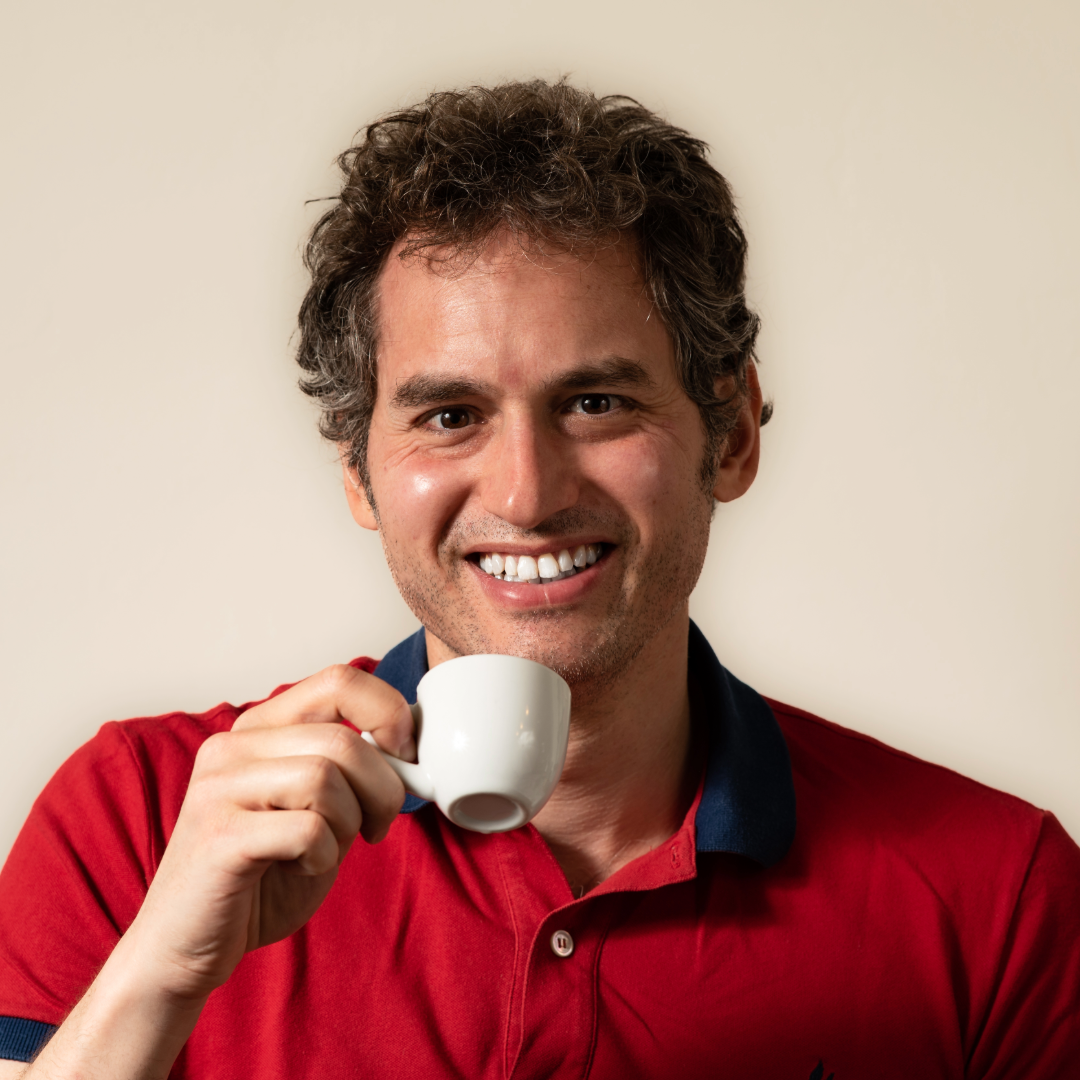