Red Teaming AI: The Devil Is In The Details
Sina Fazelpour, Dylan Hadfield-Menell, Luca Belli / Feb 9, 2024On October 30, 2023, President Joe Biden released an executive order on “Safe, Secure, and Trustworthy Development and Use of Artificial Intelligence.” The order discusses a combination of mitigation strategies to manage the risks AI systems can pose. A distinctive feature of the order is an emphasis on “red-teaming” AI systems to identify their vulnerabilities. The order directs developers of high-risk AI models to disclose the results of red-teaming efforts and directs the National Institute of Standards and Technology (NIST) to develop rigorous guidelines for red-teaming efforts.
The recognition of the importance of red-teaming is a promising development. But to realize the benefits, the operationalization of red-teaming must be sensitive to questions about values and power. Otherwise, red-teaming risks devolving into a mere rubber stamp for industry’s products. To be truly effective, red-teaming practices must be concrete, transparent, and independent. Where possible, they should be subject to democratic oversight.
With red-teaming, the details matter
Common AI evaluations focus on systems’ technical characteristics, measuring them against pre-specified desired expectations. Red-teaming, in contrast, focuses on finding flaws. Here, a specialized group—the red team—uses adversarial (and potentially unconventional) tactics to push systems towards undesirable behaviors, including those previously unexpected. For instance, testing an AI chatbot might involve getting it to produce harmful content like discriminatory remarks or cyberattack instructions. By offering a way of exploring how a system can behave in dynamic interactions with users, red-teaming can enhance our foresight about potential deployment risks—however, the details of red-teaming implementation matter.
The first issue concerns what the red-teaming targets are and who sets them. AI systems pose diverse risks—from privacy breaches to bias and misinformation. What targets are set for red-teaming and how they are communicated can shape the scope and nature of identified vulnerabilities. For instance, a system might mistakenly appear non-discriminatory if surfacing various discriminatory behaviors wasn't explicitly part of the red team's agenda. Alternatively, being overly prescriptive can stifle the red team from thinking outside the box of already anticipated risks, diminishing one of red-teaming’s key benefits. This raises questions about the authority and expertise required to set comprehensive yet flexible targets aligned with the practice's objectives.
Second, we must ask who the red team is and why they were chosen. Understanding the red team's selection criteria, composition, incentives, expertise, and resources is crucial for gauging the implications of their findings. Consider red-teaming an AI chatbot for healthcare: a team comprising AI engineers from a competitor may spot different vulnerabilities than one from an academic lab. Both teams will likely explore different concerns than a team selected from advocacy groups working on behalf of underserved patient populations. Lack of transparency about the composition of the red team can lead to an overemphasis on risks that need specialized knowledge or resources. Alternatively, it can result in missing vulnerabilities that the team did not consider or know how to evaluate.
Third, we need to consider what constitutes “success” and “failure” in red-teaming attacks and according to whom. While everyone might agree that getting an AI to reveal someone's social security number is an obvious privacy breach, evaluating other red-teaming outcomes can be more complex and contested. Confirming the effectiveness of an AI-generated bioweapon recipe, rating the toxicity of AI output, or setting reasonable timeframes for a failed attack are tasks that demand significant verification capabilities, invite disagreements, or require pre-established standards. The absence of clear or well-justified criteria can cause serious disconnects between red-teaming intentions and implementation.
Towards transparency and democratic governance
There is currently no consensus on how to deal with these and other challenges. This is not unusual. Making progress in emerging technologies also requires creating novel tools and standards for evaluating and governing them. That said, we do have a general idea about the path ahead. First, our standards must emphasize transparency about how and on what grounds choices about the red-team targets, composition, and standards of success are made. Second, at least in some cases, we need democratic input into making those choices and navigating the relevant trade-offs. Third, an independent oversight mechanism ensures sustained transparency and democratic governance. These standards should learn from existing practices that complement cybersecurity vulnerability identification with responsible disclosure.
The recent power struggle to control OpenAI’s board highlights the limits of AI self-regulation. Voluntary commitments become even more insidious when tied to loosely defined practices such as red-teaming. Such ill-defined evaluations provide companies with a superficial way to self-certify their products. Worse, their ambiguity also enables those companies to become the gatekeepers to the means of their accountability. Policies emphasizing concreteness, transparency, and independence better foster a responsible AI landscape than speculation and misplaced trust about the governability of the individual personalities that lead tech companies in that landscape.
Authors
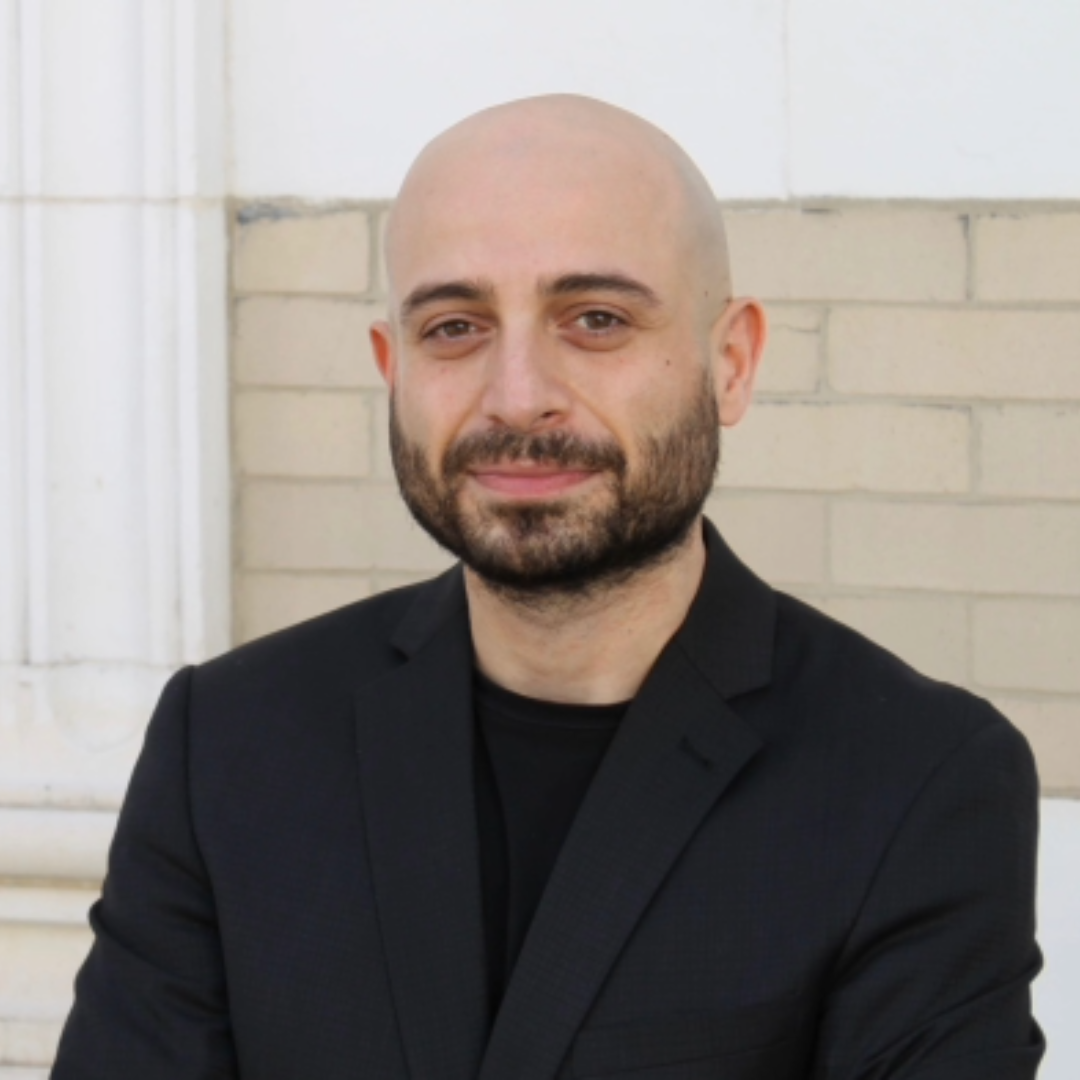
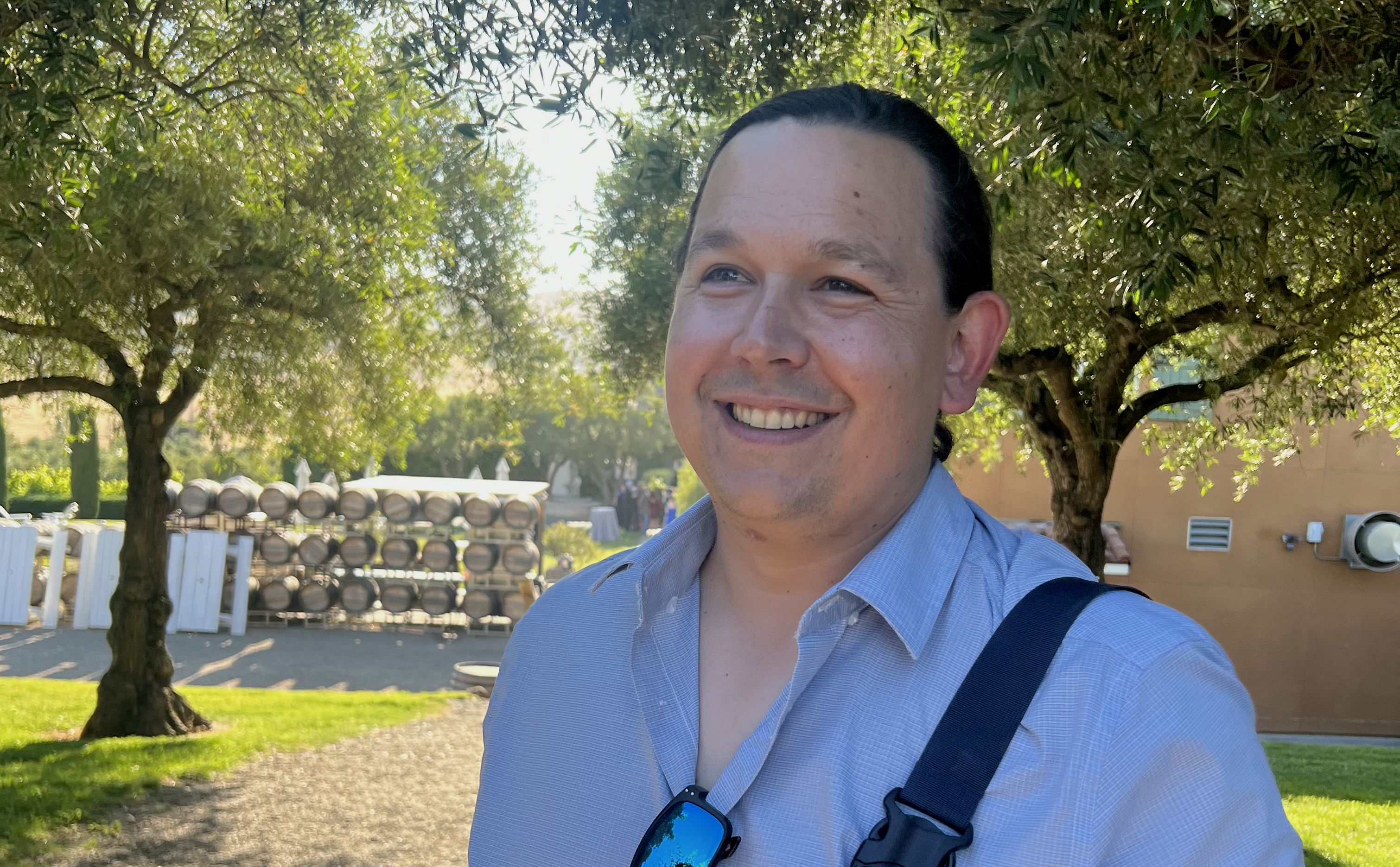
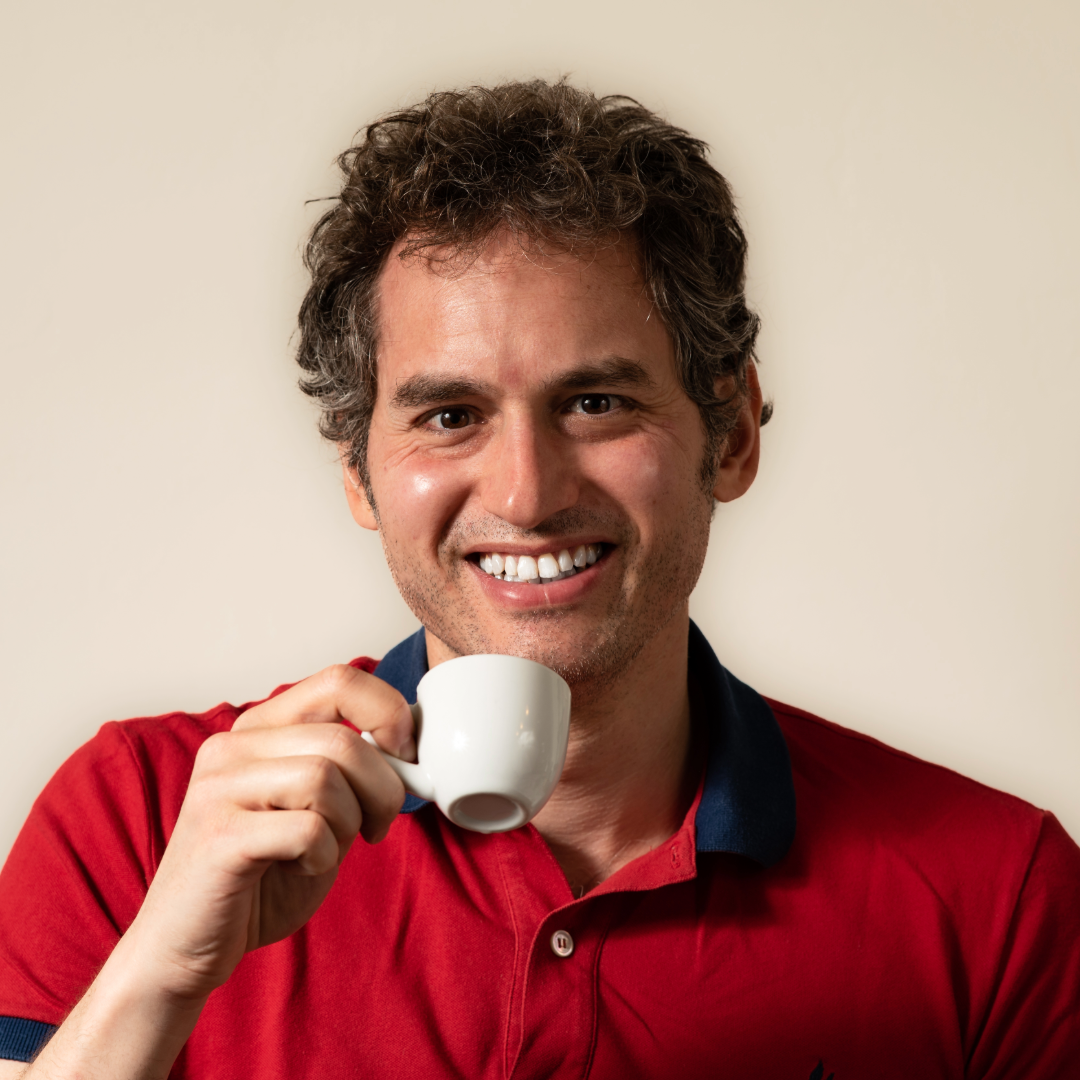