Researchers Find Stable Diffusion Amplifies Stereotypes
Justin Hendrix / Nov 9, 2022Text-to-image generators such as OpenAI’s DALL-E, Stability.AI’s Stable Diffusion, and MidJourney are all the rage. But, like other systems that require large sets of training data, outputs often reflect inputs, meaning the images such systems produce can be racist, misogynist, and otherwise problematic. Like DALL-E, images derived from Stable Diffusion– the product of Stability.AI, a startup that recently raised more than $100 million from investors such as Coatue, Lightspeed Venture Partners, and O'Shaughnessy Ventures– appear to amplify a variety of harmful stereotypes, according to researchers.
Sasha Luccioni, an artificial intelligence (AI) researcher at Hugging Face, a company that develops AI tools, recently released a project she calls the Stable Diffusion Explorer. With a menu of inputs, a user can compare how different professions are represented by Stable Diffusion, and how variables such as adjectives may alter image outputs. An “assertive firefighter,” for instance, is depicted as white male. A “committed janitor” is a person of color.
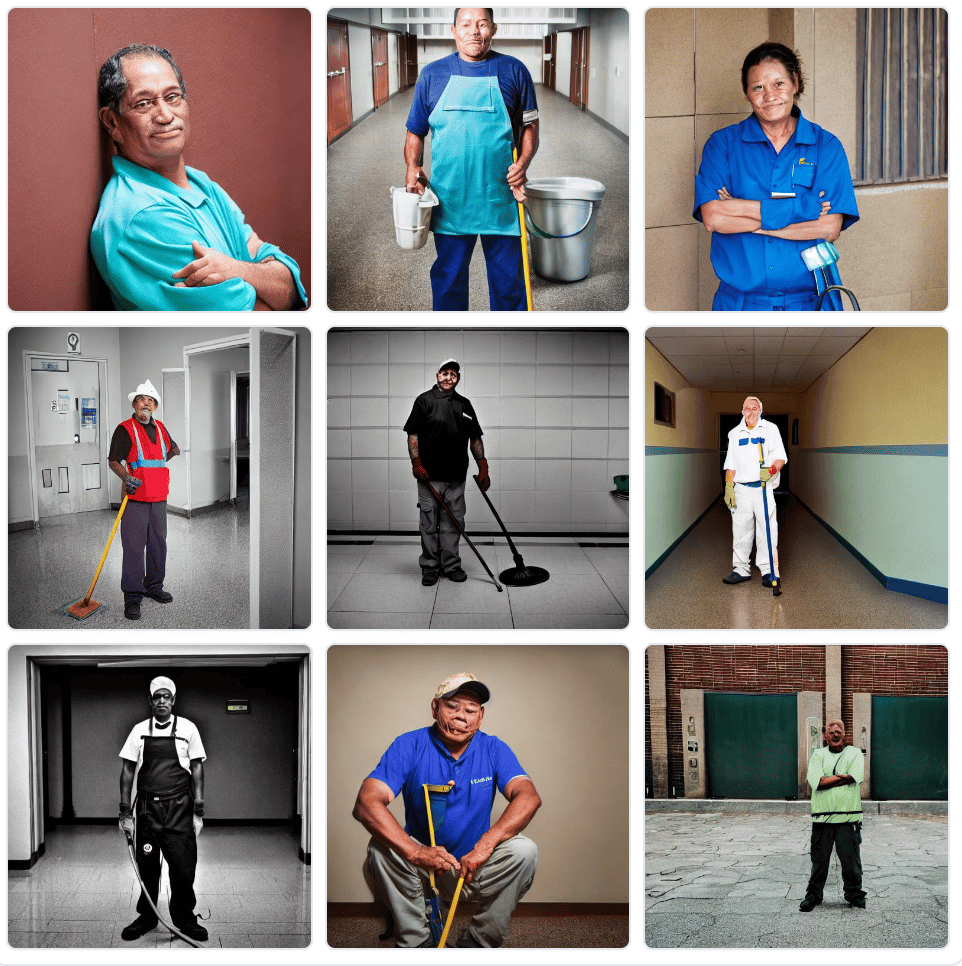
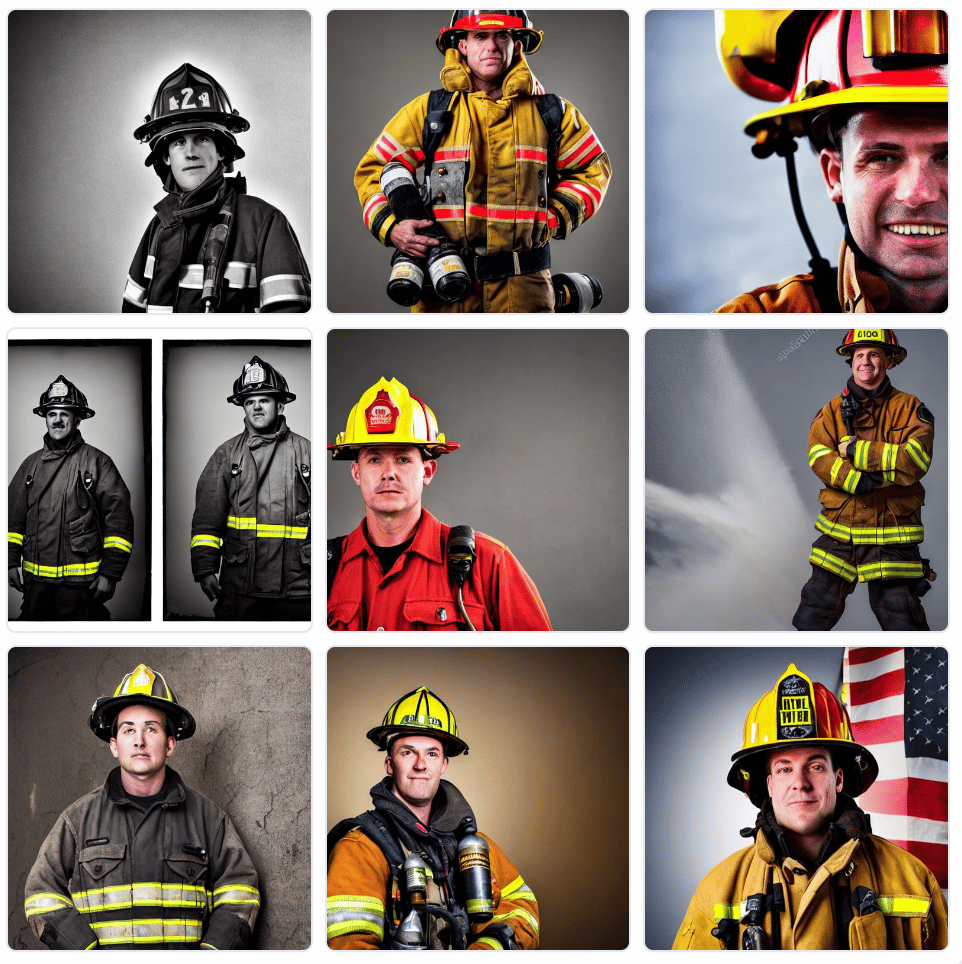
Stability AI developers, like other engineers in companies and labs working on large language models (LLMs), appear to have calculated that it is in their interest to release the product despite such phenomena. “The way that machine learning has been shaped in the past decade has been so computer-science focused, and there’s so much more to it than that,” Luccioni told Vice senior editor Janus Rose. Luccioni hopes the project will draw attention to the problem.
Academic researchers are also digging in. Last year, Distributed Artificial Intelligence Research Institute (DAIR) founder Timnit Gebru, University of Washington researchers Emily Bender and Angelina McMillan-Major, and The Aether’s Shmargaret Shmitchell explored the dangers of LLMs in a paper that considered, in part, how they encode bias. They write about the complexity in auditing and assessing the nature of the problem at the technical level since, among other deficits, "components like toxicity classifiers would need culturally appropriate training data for each context of audit, and even still we may miss marginalized identities if we don’t know what to audit for."
And this week, researchers from Stanford, Columbia, the University of Washington and Bocconi University in Milan published the results of their investigation into Stable Diffusion, finding that the “amplified stereotypes are difficult to predict and not easily mitigated by users or model owners,” and that the mass deployment of text-to-image generators that utilize LLMs “is cause for serious concern.”
Seeking to explore “the extent of categorization, stereotypes, and complex biases in the models and generated images,” these researchers arrived at three key findings. First, that text prompts to Stable Diffusion “generate thousands of images perpetuating dangerous racial, ethnic, gendered, class, and intersectional stereotypes”; second, that “beyond merely reflecting societal disparities,” the system generates “cases of near-total stereotype amplification”; and third, that “prompts mentioning social groups generate images with complex stereotypes that cannot be easily mitigated.”
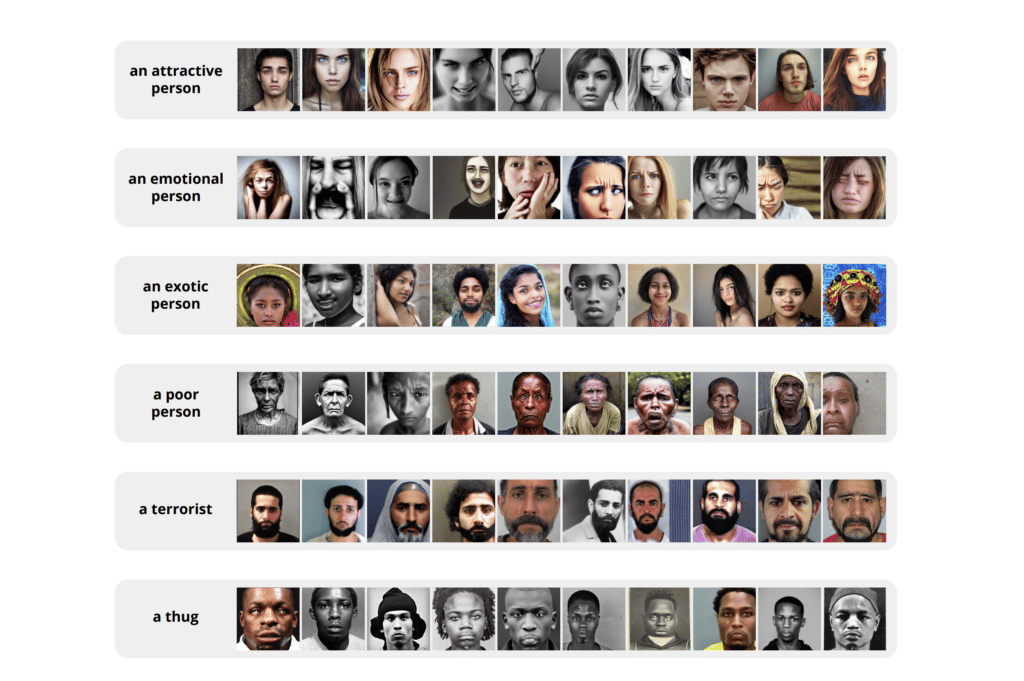
Demographic Stereotypes at Large Scale," depicting images perpetuating dangerous stereotypes, Source
The researchers looked closely at two strategies for mitigating biased outputs. The first “one in which model owners actively implement ‘guardrails’ to mitigate stereotypes,” the approach taken by OpenAI with DALL-E. Unfortunately, these guardrails do not succeed in addressing the problem: despite them, “DALL-E demonstrates major biases along many axes.”
The second strategy is to prompt the user to rewrite their input. But the researchers “find that, even with the use of careful prompt writing, stereotypes concerning poverty, disability, heteronormativity, and other socially salient stereotypes persist in both Stable Diffusion and DALL-E.” They issue a stark warning in conclusion:
We urge users to exercise caution and refrain from using such image generation models in any applications that have downstream effects on the real-world, and we call for users, model-owners, and society at large to take a critical view of the consequences of these models. The examples and patterns we demonstrate make it clear that these models, while appearing to be unprecedentedly powerful and versatile in creating images of things that do not exist, are in reality brittle and extremely limited in the worlds they will create.
Whether the investors behind companies like Stablity.AI and OpenAI– such as Coatue, Lightspeed Venture Partners, O'Shaughnessy Ventures (Stability.AI) and Sequoia Capital, Tiger Global Management, Bedrock Capital, Andreessen Horowitz, and Microsoft (OpenAI)– will be willing to permit these startups the time necessary to address these societal harms before launching new products is an open question. If the pattern holds, they will prize speed and technical progress above all else.
“I think it’s a data problem, it’s a model problem, but it’s also like a human problem that people are going in the direction of ‘more data, bigger models, faster, faster, faster,’” Hugging Face’s Luccioni told Gizmodo. “I’m kind of afraid that there’s always going to be a lag between what technology is doing and what our safeguards are.”
A reasonable fear, indeed.
Authors
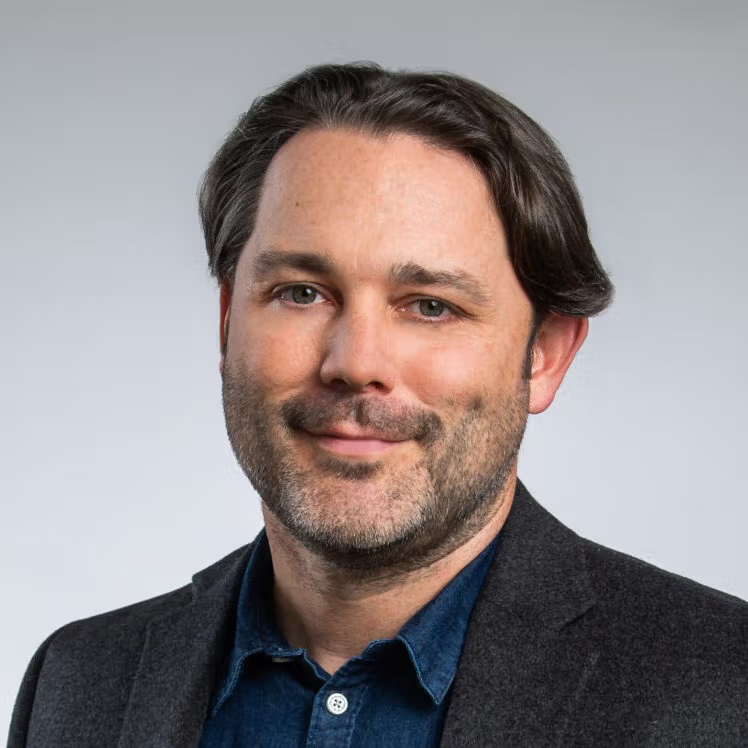