Transcript: Dave Willner on Moderating with AI at the Institute for Rebooting Social Media
Prithvi Iyer / Apr 3, 2024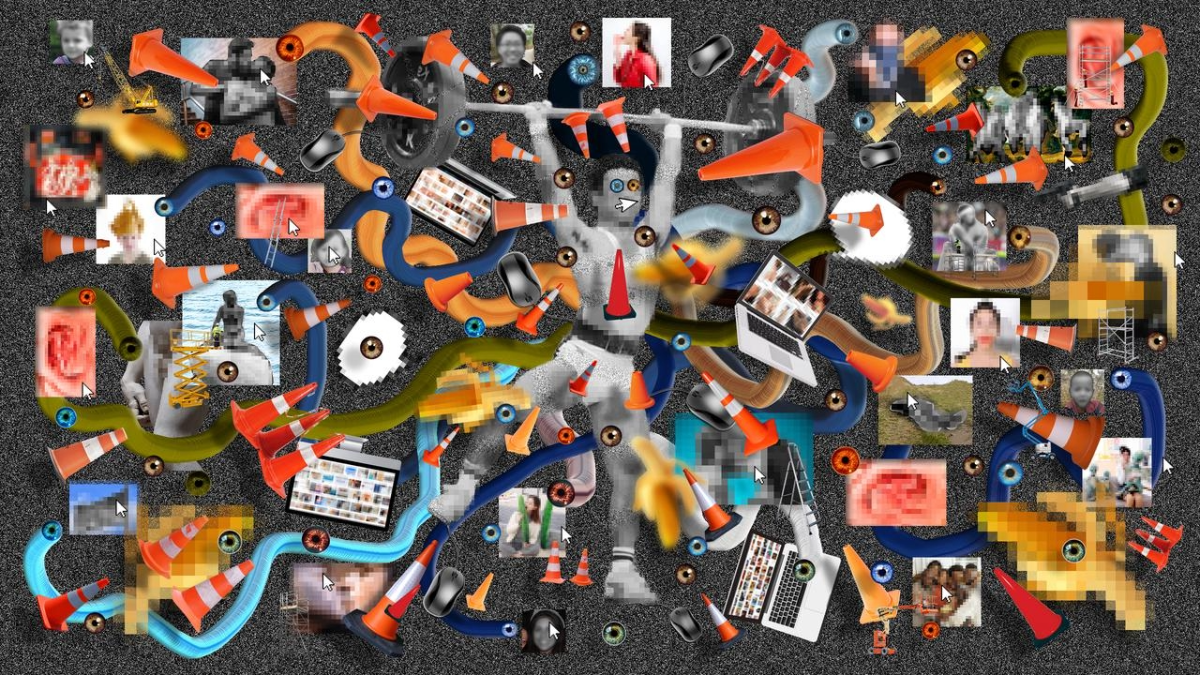
Collage depicting human-AI collaboration in content moderation, by Anne Fehres and Luke Conroy & AI4Media / Better Images of AI / CC-BY 4.0
David Willner, a non-resident fellow at the Stanford Cyber Policy Center and former head of trust and safety at OpenAI, recently delivered a talk on the potential for large language models (LLMs) to improve content moderation practices as part of the Rebooting Social Media Speaker Series organized by the Berkman Klein Center at Harvard University.
Content moderation plays a crucial role in ensuring the safety, integrity, and inclusivity of online platforms. Willner makes the case that human beings are “bad at content moderation.” He says the process of moderation involves two key components: establishing values and classification. While values may be easy to agree upon, classifying online content in accordance with those values is much harder. For example, Willner discussed a controversy on Facebook about images of breastfeeding, wherein even if there is value alignment (i.e., such images should not be censored), it is hard to ensure that individual moderators can scalably implement the policy without error. Thus, Willner emphasized the need to shift the focus from values to the functionality of classification, acknowledging the complexities involved in moderating diverse and dynamic online content.
Willner pointed out that manual moderation processes fall short of addressing the sheer volume and complexity of user-generated content. He highlighted the mental health toll of moderation while sharing personal anecdotes about the challenges of human moderation, which included dealing with boredom, limited memory of human moderators, and an inability to arrive at consensus. During his time at Facebook, Willner noticed that employees who had significant technical expertise only agreed some percentage of the time on what constitutes child sexual abuse material (CSAM). Thus, the challenges of classifying problematic content are especially prominent for moderators, often employed as contract workers with little technical know-how.
So, how can LLMs potentially change content moderation? According to Willner, LLMs offer several distinct advantages over human content moderation, including better short-term memory, consistent decision-making, and enhanced reasoning capabilities. (Willner and his colleague Samidh Chakrabarti recently shared insights on this subject for Tech Policy Press.) In essence, LLMs can engage in the core activity of content moderation, such as reading a policy document and following its rules. LLMs can also potentially transform how policy documents are written and implemented. By instantly adapting to word choice variations and allowing for real-time testing of different policy formulations, LLMs offer a dynamic and iterative approach to content moderation.
In addition, the ability to provide transparent explanations for moderation decisions may facilitate greater accountability. Oftentimes, human moderators are unable to provide concrete explanations for their classification decisions, and LLMs are able to provide detailed reasoning by extracting the specific clause in the policy document that informed its decision. Willner spoke about an instance wherein an LLM's reasoning made him change his own view about a particular moderation decision. Willner also spoke about the value of fine-tuning smaller LLMs specifically for content moderation tasks rather than only relying on off-the-shelf general models like GPT-4.
Willner urged attendees to exercise caution against viewing LLMs as a panacea for content moderation challenges. He acknowledged the inevitability of errors and biases in AI-driven moderation systems, and emphasized the importance of ongoing refinement and transparency in decision-making processes. On the question of LLM hallucinations, Willner emphasized the importance of including the policy document as a prompt for each decision. This helps the model stay grounded in the text, and the only task for the model is to read the rules in the policy document to classify content.
What follows is a lightly edited transcript of the presentation, drawn from the YouTube recording of it.
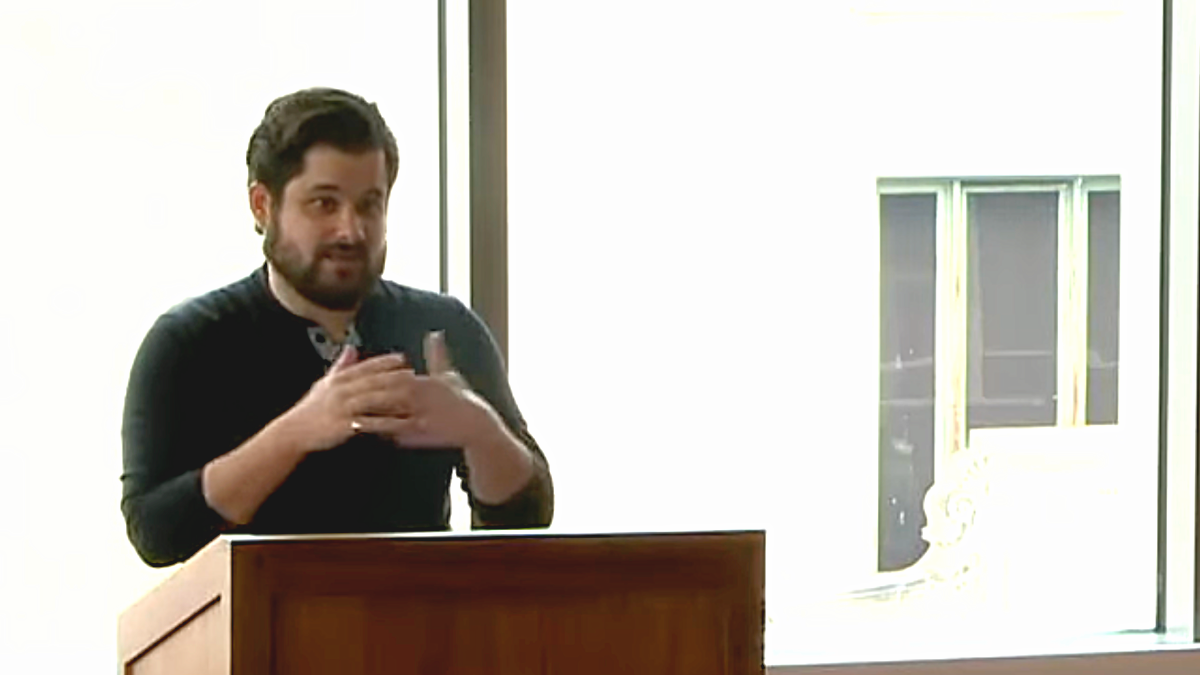
Dave Willner at Harvard University, March 21, 2024. Source
Moderator:
Thank you everyone in the room and on Zoom for joining us for today's RSM Speaker Series event. We really cannot be more excited to welcome Dave Willner for a talk entitled Moderating AI and Moderating With AI. I'm sure everyone is excited to hear Dave, so I'll offer just the briefest note of introduction.
Dave was a member of Facebook's original team of moderators, playing a key role writing its earliest content policies, and building the teams and enforce them. After leaving Facebook, he took on roles building the community policy team at AirBnB and as head of trust and safety at OpenAI. He's now a non-resident fellow in the program on Governance of Emerging Technologies at the Stanford Cyber Policy Center. Dave's experience and content moderation and trust and safety expands almost the entirety of their histories as fields, so we're extremely lucky to welcome him today to hear his thoughts on where the space may be heading. So with that, Dave Willner.
Dave Willner:
Hi, folks. Yeah, it's great to be here. Just wanted to start by apologizing. As it was noted, I've been doing this the entire time and it is all my fault, so sorry about that. I wanted to make a case to all of you around the use of AI in content moderation and how I expect it to change things. I have come to think that powerful foundation models are going to fundamentally transform how we do moderation. There's been a lot of focus on the novel risks that those models present. That's fine. Those things are true. I'm not going to dwell on that today. I think it's been fairly well covered, but the models are also, because of their unique capabilities and ways of working, going to be very useful in solving problems that have previously been intractable. That's those sort of solutions also, I think, going to prove deeply relevant to alignment questions in AI itself because at least today, our current ways of controlling and steering models are themselves downstream of techniques that actually have a lot of shared DNA with how we do content moderation for the present.
So just to sort of briefly recover who I am, why you should care, how I think about any of this, I have been working in this field for about 16 years at the forefront of controlling social technology. First in social media, then in the sharing economy, then in AI. I spent a lot of that time trying to not just grapple with the of emerging technology, but grapple with using emerging technology to solve the problems it creates. So in addition to working on policy itself, I've spent a lot of time at the intersection of operations policy and tooling and figuring out how we actually do the rules that these platforms claim to have, and I'm going to dwell a lot on that question of actual performance in the talk today.
Currently, I'm a fellow at the Stanford Cyber Policy Center. I'm spending a bunch of time on this subject learning how to use LLMs to do content moderation with a guy named Samidh Chakrabarti who's another fellow at the center. He ran civic integrity at Meta from 2015 to 2021. We ended up doing the same fellowship sort of coincidentally, having very similar thoughts. Beyond using off the shelf models, we're also doing some work trying to train smaller large language models to be good at this task, specifically because we think that more efficient models to be able to do this would be a really helpful contribution to the space.
I bring all of that up just to make the point that my perspective here is very much a practitioner's perspective, not an academic perspective. I come to this as someone just desperately trying to solve these problems for the last nearly 20 years and very focused on what practically works and how we use these tools in practice, not merely in theory.
So, first, to just sort of set the table about why I have this strong belief about the importance of AI in the future of content moderation, I want to do some grounding about how I see content moderation today, why it works and, frankly, why it doesn't work very well. I think there's broad spread agreement it doesn't work very well. It doesn't seem like it would be controversial to say here. Bad things keep happening to clearly innocent people. Watching the child safety hearings in the Senate earlier this year is enough to sort of demonstrate that to anyone. There are very serious social externalities that are going on, and this has naturally led to a lot of public theorizing as to why. There's a lot of discourse in the atmosphere about why social media moderation doesn't work well. I've come to think we're basically having a 'problem of evil' conversation about tech giants.
The 'problem of evil' is this idea in theology that's concerned with reconciling the existence of a benevolent and omnipotent God with suffering in the world, and we're having a 'problem of evil' conversation about Mark Zuckerberg. Right? If Mark Zuckerberg is good and in full control of Facebook, why do bad things happen to people on his platform? A lot of the popular explanations focus on this idea of benevolence. There is either the notion that they aren't trying, that the tech platforms don't care, they're indifferent. There's the notion that they're greedy, that they sort of do care, but they don't want to spend the money; or there's the notion they're actively malicious, that they have bad values that are antisocial and that we don't want.
Those things may or may not be true. I don't think they're the root of the problem. The root of the problem is that they're very far from omnipotent. Put in another way, we're bad at content moderation because we're bad at content moderation. We're not good at doing the core activity. To understand why we're bad at it, it's important to take apart moderation into a couple of components, values and the actual classification task.
The values piece of moderation receives a lot of attention. There's a lot of discussion about what the rules should be. Community policies are primarily understood, I think, as expressions of companies' values. That's not untrue, but it is not the most significant thing that those policies express. I've come to believe that the focus on values is a form of 'bikeshedding.' Bikeshedding is this idea, sort of relies on a story sort of gaslighting, that if we were all on the board of a nuclear power reactor, all else equal, we would spend more time discussing the color of the new bike shed of the reactor than we would discussing nuclear safety because more of us can have opinions about colors and bike sheds than are qualified to have opinions about nuclear safety. Values function in that way in this discussion. Everybody has values, so it's easy to have opinions about values.
The reality is that the sorting task underneath the values, the classification task is the thing that we are very bad at and that dominates any possible set of values. To get into why classification matters, I'll give you some examples of how that is the case.
There are lots of situations where the value proposition that you want to achieve is not particularly in dispute, but where the ability to do it is very, very hard. To give you some social media examples, reclaimed slurs are a great example of this. It's very intuitive to say we want to allow people who are members of the community to use certain language, but actually doing that requires you to know at scale who is a member of that community, who they're speaking to, what the actual context of the conversation is. So doing the thing is hard, even if agreeing on whether or not saying it is good or bad is hard.
Similarly, the controversies here are often made worse by public pressure. Facebook's breastfeeding photos controversy back in 2009, 2010 was very much one of these problems of classification. The question of 'should breastfeeding mothers be able to upload photos of their children on Facebook' is not really that interesting of a policy question. Getting a moderation system to very consistently distinguish between nudity where there's babies involved and it counts as breastfeeding and nudity where there's not is hard and flawed, and so the ability to execute on the policy is challenging.
The Napalm Girl incident that happened in 2015, Napalm Girl is a reference here to a photo that won Pulitzer Prize. It was a photo of a girl who'd been attacked in Vietnam. It's very, very intuitive to say all of the Pulitzer Prize-winning photos should be able to be on Facebook, but you have to know what all the Pulitzer Prize-winning photos are and get everybody doing moderation to know that, too, or you can't actually achieve that policy goal.
So why are we bad at large-scale classification? Well, we're bad at large-scale classification because fundamentally we're trying to solve an industrial scale problem with pre-industrial solutions. Social media is this mass distribution machine that requires no intervention for data. It allows billions of people to talk to billions of other people nearly instantaneously without any direct human intervention in the communication itself. It's pure mass production of speech. But we don't really have mass production capability for the ability to moderate speech. We're still stuck in essentially a piece work system. Piece work was a way of manufacturing textiles and articles of clothing in the early industrial revolution when we invented yarn machines but hadn't been invented machines that could do sock knitting, and so work would be parceled out to people in their homes to be done in an artisanal way to a particular spec.
Moderation today essentially works exactly like that. Right? We have specific people, sometimes distributed, sometimes in one place, working against a document that tells them how to grade content on an artisanal level except they're doing it en masse. Systems like that have trouble achieving very high degrees of consistency. We don't have machinery to do those core lines of the process. I'm going to sort of get into a little bit why humans struggle so much to do those processes well. Even where we do have machinery for different parts of this classification process, the machinery itself mostly replicates the problems that humans introduce into the system as it exists today. And then, I think finally the nature of language itself probably caps how well we can do this. We are not making machine parts here or knitting fabric. We are ultimately dealing with classification of language and culture, which is a fuzzy activity inherently, and so the upper boundary of excellence is probably fairly low.
That said, until we make progress on at least some of those human or machine constraints, we're not going to see better moderation online. I think AI is going to help with that because it surpasses both human capability and the capability of our current machines in a number of very specific ways that I'm going to get into after this, after sort of digging into specifically how humans fail, because the ways in which we are inadequate to these tasks are important to understanding the ways in which LLMs to be helpful.
Okay, so why are human beings bad at classification? We're bad at classification for a lot of physical reasons. Our working memories are really, really small. The length of the content policies you can feasibly write as a policy writer are maybe a few pages, maybe five, maybe six. That's not because we couldn't write a much longer of what hate speech might be or how to tell whether a photo contains unity. It's because most people can't actually use a hundred-page document about what hate speech might be to make a thousand decisions a day, particularly not if you want them to stay up to date on what that document says and you're changing it all the time.
Our long-term memories are also very unreliable. I sort of alluded to this in the Napalm Girl context. But, sort of notions of art, which is again a thing I think we all think is probably good and want to have to be on Facebook or notions of what a real name is, are basically look-up questions. There's no art pixels. Art is just all this stuff we've decided is art as a society. And so in order to treat that stuff differently, you have to know what it is, which means you have to remember what it is, and people are not terribly good at remembering huge amounts of very specific facts about individual pieces of content. It can be done, but that's what getting a PhD is for. It's not something that you do as an hourly job.
We're quickly exhausted. This work gets coded as introductory-level work. It's entry-level work. But focusing intently on content for thousands of repetitions for eight or 12 hours a day is intensely, intensely draining. People get tired, they make mistakes, they get bored, which is another sort of understated part of this. There's the trauma and emotional part of the labor has been much discussed and that is very much real. But honestly, a lot of the time the work is dull. Many of the things you're looking at are not interesting to classify, they're not violating, they're just kind of random noise. It's a little bit like staring at white noise on a television screen and waiting to see if something meaningful shows up, and it's very hard for people to maintain focus under those kinds of conditions.
We rely on our own internal models. People don't really use the rules to make these decisions. They read the rules once, use them a couple of times, internalize some sort of approximate model of whatever the rules say well enough to not get in trouble with their boss, and then just keep doing that until they get in trouble again. And so as these rules change, people lag in that change.
We also typically can't recall our reasoning. If you ask a given moderator why they made a particular decision when they made it yesterday, if they made a thousand decisions, they're almost certainly not going to be able to tell you. And so while this is this human process which sort of seems like it has meaning, the meaning is often not retrievable.
And then finally, and this one is often hardest for folks to grapple with, we really do not have any shared common sense, and there's a couple of specific examples that were really important in shaping my thinking here. Warning upfront: all of this is going to get a little unpleasant from a content point of view. We were trying to figure out how to classify CSAM, child sexual abuse material at Facebook for the purposes of creating the photo DNA databases that today underlie a lot of the attempts to control that material online. We had 12 folks who've been doing this for a year, had been reporting information to NCMEC that entire time. These were full-time employees. Like me, these were kids who went to Stanford and Harvard. When we asked them to classify material to simply report to NCMEC and not report to NCMEC without talking to each other, they could only agree about 40% of the time, and they've been doing this for a year on what you would intuitively think is the worst thing, the easiest thing to get consensus on. No consensus.
Most other areas are actually worse than that. When we first tried to outsource nudity moderation to India, we ran into a similar problem where we had rules that had said, also take down anything that is sexually explicit beyond a bunch of particular things we listed, and immediately the moderators started taking down photos of people kissing and holding hands because what we meant and what they understood those words to mean were not the same, because you just can't assume shared reference or shared values.
All of that is made worse by the economic way we currently organize this labor. So the work is very notably poorly compensated. I think some of that is probably inevitable, given both the scale of which it is done and a bunch of the other things we're going to get into here. Being sort of forced into consistency is pretty demoralizing. It's an alienating form of labor, particularly because this is labor where people have strong moral feelings about what they're being asked to do. And so it's unnatural to be asked to put on another morality, but putting on that other morality to get everybody on the same page is the heart of the activity, so you kind of can't avoid that, and that is itself draining and not a ton of fun. So people who have better options leave. These are high-turnover jobs as a rule.
In the context of this is well known in the context of our customer support, but even for Airbnb's outsourced trust and safety teams, the average retention is about nine months. It's a very, very short period of time because when people have the ability to do better work, they go. That undermines the accrual of expertise. It also means that you have to invest a ton into sort of training and updating the system because you're constantly teaching new people to do this stuff and having to constantly reorient them as you make changes. So the entire system is extremely cumbersome and doesn't lead to sort of updating results.
Cool. Okay. Hopefully, I have convinced you at this point people are not good at sorting things into piles.
Why is our automation bad at this? Right? Our existing automation is bad at this because all it's really doing is statistically copying what all those people who are not good at this did. Our most advanced automation techniques, our black box machine learning is just predicting what a human moderator would do if you asked them to sort a piece of content according to a policy. It's just a mathematical simulation of the results you would get if you had bothered to ask a person, which is very useful to be clear because a lot of the time it does a pretty good job and it means you can avoid asking people, which is great. Avoids trauma much faster, has some real upsides. But it also means that it inherits the fuzziness and the unreliability and the non specificity that we bring to that process.
There are other forms of automation, but they're actually even simpler, right? They're either asserted if then rules, exact word or pattern matching; they're all even less nuanced and less capable. We have no automation that does the activity that humans are actually doing as part of this classification process. We only have automation that simulates the outcome of the activity that they've been doing.
Actually, the automation we have today adds a bunch of other problems. Their decisions are meaningless. Very literally meaningless, right? They're not making an argument about why a particular piece of content fits or doesn't fit a given policy; they're simply saying, "95%, this is shaped the same way as other things you told me violated this policy." There's no meaning to the decision, which both makes it hard to debug individual decisions and is very disturbing for, frankly, people who are subject to those decisions because we want these things to have meaning, to be able to dispute them and to be able to argue with them.
Updating the models is also extremely cumbersome. Training large machine models under current circumstances needs thousands, tens of thousands of examples, which means every time you change your policy, not only are you having to update the humans and wait for that to all phase in, you then have to wait for all those humans to learn the tens of thousands of things to then be able to train your machine learning model. So our automation is also often very out of date to the point where it's typically not fully synchronized with whatever given platform's policy allegedly is at any particular time, which is confusing and results in outcomes that are not ideal. So also, machine is not very good at classification, at least in our current circumstances.
And then on top of that, I think the best we can ever hope for here is significantly less precise than what we can ever hope for in material manufacturing. Despite all of the manufacturing analogies I've been making, we are not, in fact, making steel cylinders with a metal frame lathe; we are playing around with words, and words are inherently vague. The language itself is just not terribly precise, and that's particularly true in mass scale; social media, where people often write frankly fairly badly or unclearly, or approximately from a jargony point of view. Everyday language is not meant to convey precisely specific meanings. It's meant to be efficient in communicating between people with a shared context, and social media moderation doesn't share that context. It's a very radically postmodern exercise. All of the authors might as well be dead. There's only the text. You're sort of just staring at these word boards after the fact, and that renders them very, very difficult to understand.
A version of this is the conversation about cultural context that comes up a lot. Having more specific cultural context is helpful here, but that's only a version of it, and in a lot of ways, the easiest version to solve. Local interpersonal context is as big a part of this problem as broader cultural context. If I call you an elephant, am I calling you old, wrinkly, gray, fat, wise, or Republican? Right? There's no way to know the answer to that question outside of our specific and interpersonal relationship, and there never will be, and so we're sort of tapped at the maximum here, so that's the doom part of this.
As an aside, all of the problems I outlined are also problems for AI alignment under current circumstances. So our primary techniques for AI alignment reinforcement learning rely directly on curated data sets of desirable behavior that we are trying to get the machines to copy. All we're doing in RL is curating a set of prompts and responses to the model and allegedly from the model that we want the model to behave more like, and then doing a mathematical process to get the model to ape that behavior, which means that ultimately what we are aligning the model to do is dependent on the same kind of content classification. It has the same kind of content classification problems that I've just been talking about in the social media context, and you can see this in the two kinds of reinforcement learning that get talked about most frequently. Reinforcement learning with human feedback, which is where we're having humans do the classification, and reinforcement learning with AI feedback, which is where we're using AI to do the classification. Even in the activity itself, this is baked in there. And then all of our other techniques for controlling the output of generative AI today are just wrapping content moderation techniques around either the input prompts that people send in the model or the outputs from the model in response to inputs. It's all the same stuff.
And so thinking about how we can do this core task better is relevant both to social media but actually also deeply relevant to conversations around AI safety. You can see this in the Google Gemini blow up, which was, at least from my point of view, almost certainly either some poorly thought through alignment instructions or some poorly thought through moderation and modification of the incoming problems to try to correct for problems in the model itself. It's simply failures of classification technique and a lack of nuance in doing that task.
As an example, ChatGPT when we first launched it wouldn't tell you facts about sharks because we had taught the model that violence was not good. We didn't want it to help you plan violence, and we also didn't want it to graphically describe violence. It wildly over-rotated and was like, got it, bears are canceled, no more bears, we can't talk about bears, which is this perfect example of this sort of classification over-response of these kinds of systems.
So setting all of that context, generative AI actually I think going to be very helpful here. Used properly, it is possible for it to exceed both humans and machines under existing real circumstances, and by used properly, I mean a very specific thing. So the naming, generative AI, is in a lot of ways, I think, distracting for this purpose. It's more important to understand it as language parsing AI, reading AI. We have machines now that can do something that functionally is equivalent to a human reading a document and responding to what it said, which means we have a machine that can directly address the core activity that a human moderator is doing instead of merely producing the result.
I am not speaking theoretically here. This already works shockingly well. One of the first things we did internally with GPT-4 when it became available to us in August of a couple of years ago was try to figure out how to use it to do content moderation. Within a week or two, me and a couple of other engineers were able to get to 90% plus consistency with my decisions with the model reading a document that any of you could read and following the instructions that provided in order to classify content. Things have only gotten better from there. Open AI published a blog post about this middle of last year about using GPT-4 with content moderation. There are multiple startups pursuing this path, and it's something that I've continued to work on at Stanford with Samidh, who is particularly interested in fine-tuning smaller models to be able to do this because the smaller you can make the model, the more broadly adoptable it will be.
Doing content moderation with GPT-4 is a little bit like going to a grocery store in a Ferrari. You get there. It's very expensive and most people don't have one, and so building a smaller, more compact, more usable, more broadly accessible system seems to us to be pretty important. When I say you can in fact use these models to read policy text, follow it, and classify content, that is not theory, that is already happening.
Used in this way, the LLS directly address a number of the problems with human moderators. Their short-term memory is already better than ours. The largest models have context links of hundreds of pages of text, and so you can load tons of information into a model for making a specific decision. Their long-term memory is or will be more reliable using things like databases plugged into a model to be able to give very exact recall of large amounts of information. They don't get bored, they don't get tired, they don't lose focus, they don't seek better jobs, they don't experience trauma, which is a pretty important part of this. There's, I think, a real moral case to do that here as well. We can reasonably expect them to record what they did and why they did it according to the text as they understood it at the time every single time they make a decision and store all of that information, which helps with things like the requirement for explicability that is embedded in a lot of the recent law.
They're also way, way, way faster. Even the largest models are much faster than people even doing this very cumbersome policy text-driven process. And then on the flip side, the LLMs are better than existing machinery because again, they're directly doing the task, and so they produce responses that are scrutable or at least feel meaningful. There's a broader philosophical question here about whether or not they're really reasoning. Honestly, for these purposes, I don't think it matters because they are producing reason-shaped answers in language, and those reason shaped answers can be used to debug the decisions the model made by changing the instructions you gave it. So when you have a model make one of these decisions, if you don't agree with this decision, you can simply ask it why. It will make a bunch of words at you. Whatever you sort of think metaphysically those words mean, they are useful for understanding how it was functioning, and you can incorporate that feedback back into the policy text to produce change behavior, and this works really, really very well.
And so it's functionally equivalent to an explanation in the sense that it is a word shaped response that helps you understand what happened and why and do something about it. And so, at least to me, a lot of this sort of hand wringing around whether or not this is true reason is irrelevant, at least functionally for this task in short term. That's not me dismissing those concerns in a sort of longer term, more AGI-focused way, but for this purpose with something like GPT-4, it is neither here nor there to a very great degree.
I'd also point out that when you're dealing with really any people, but certainly people in a mass bureaucratic system, you don't understand why anybody does what they do either, and it's very, very difficult to get that recall or get reasons that mean anything out of the systems that we have today. So it's also not super clear to me that the alternative is really well-thought-out, clearly described reasons.
I don't want to stand here and sort of make the case that this is magically going to solve all of our problems, so please do not take me as saying that. So first, the systems themselves will have flaws. They're going to make mistakes. Some of those mistakes are downstream of some of the sort of language limits that I talked about earlier, but some of those mistakes are simply going to be errors or problems with the performance of the model. They're going to have biases. There's been a fair amount of reporting about this already. In the use of these systems for things like hiring decisions or other kinds of adjudication decisions, that's very real. I'm not minimizing that we need to work on those problems, but I would say that at least those are static engineering-shaped problems instead of the situation we have now where all of the individual moderators also make mistakes and also have biases, but who they are changes every nine months. And so understanding, correcting for, and controlling those biases is, essentially, I think impossible at present because it is this sort of royally massive chaos. Simply pinning down the biases to a single set of them such that we can start to try to study, understand, and engineer account for them feels better and more tractable than where we've been, where there's this essentially ever-churning cauldron of biases that is never static and therefore cannot be stabilized.
I also do think that this is going to, circling back around to my earlier point, that classification is more important than values, I weirdly think if I'm right about this, we're going to have more fighting about values because we're going to be better at doing the thing, and so what the values are is going to start to matter more. I think we've already seeing shadows of this in some of the sort of woke AI cultural war stuff that is starting to creep into AI alignment conversations and some of the reaction to Gemini. So, oddly, as we get better at doing the activity, we're just going to fight more about values.
That said, and I sort of lampshaded this earlier, I think it's morally urgent that we figure out how to do this. Even given all of those flaws, having people continue to do this work is bad. There's been a lot of focus on ways in which the work conditions can be made better, ways in which pay can be more equitable, breaks can be given, preventative techniques for controlling wellness. All of those things are good given no alternative, but in a lot of ways, they seem to me to be questions of engineering a better radiation suit when maybe we could just have a robot do it instead and not worry so much about radiation protection. The problem with Radium Girls making watch faces wasn't just that they were licking the radio, it's that they were painting watch faces with radioactive material, which is not a safe or good idea.
I think getting to the point where we can relieve the sort of direct coalface labor here from humans is an actively good thing, even though it is fraught. I think that's actually doubly true for marginalized groups personally, right? Part of the sort of perverse shadow of the request for more cultural context being ejected into moderation is it's essentially a call for the enlistment of people who are victimized by speech in the controlling of that speech to begin with, which is perverse when you think about it that way. This will mean, I think, job losses, particularly at BPOs, but again, it's not clear to me that job losses are per se bad if the jobs themselves are dangerous, toxic, and not conducive to human thriving. I also think it will mean more jobs overseeing these systems on the flip side.
So even with all of those caveats, this is a really big deal if you accept the case I have made to you because it's not just going to mean we are going to lift and drop AI in place of human frontline moderators. Right? It will change the kind of systems that are viable to have. It opens up new sort of possibilities of moderation, things like super deeply personalized moderation become more feasible, ambient moderation becomes more feasible. I think in the future, LLM-powered systems are going to allow things like Siri to prevent your grandmother from being pig-butchered over the phone, which is an inconceivable thing to try to do right now, but seems very possible in this sort of future in the same way that deeply personalized moderation filters seem possible.
It is utterly transformative of the policy drafting process. Right now, a lot of content policy is basically astrology about how moderators will work, will react to the words that you wrote. You're sort of guessing because the update time is so long and retraining is so cumbersome that you can't really do empirical testing of the outcomes of your decisions feasibly. These systems respond instantly to your word changes, which means you can actually test different versions and approaches to things and see how that produces different outcomes, which is revolutionary in terms of the policy process directly. I think it also is going to open up new policy vistas, not just new processes. Right now, we have generally global moderation standards on the social web because, frankly, it's too cumbersome to do nation-by-nation moderation for anything but the most sort of large-scale blocks. That may no longer be true. We could potentially start to think about really localized or regionalized moderation standards. And then, similarly, different moderation philosophies that no one has ever really, to my knowledge, seriously trying to engage in at scale become possible. Things like deeply intersectional approaches to moderation, which no one has ever tried to do because it's just wildly cumbersome and impractical might become possible with these sort of tools.
A bunch of those ideas are probably bad, to be clear. I'm not saying all of the things I just said should happen. I'm saying they're now not impossible, and there will be other better ideas that are now not impossible, which is going to be very interesting. Similarly, changing the cumbersomeness of our moderation technology will change the kinds of platform designs that are valuable. There's been a lot of discussion of network effects in social media. Yes, I think an under-discussed aspect of the reason we've seen a lot of centralization in social media is how annoying moderation is to do at scale. I have been very skeptical of federated solutions simply because I did not understand how that was going to work at the level of Mastodon except scale to Facebook size. These sorts of systems might actually provide the ability to make that kind of a system work.
Just as an example of this sort of consolidation I think you naturally see due to moderation, think about Reddit and the power mod situation; Reddit is technically the sort of flat-federated system, but the reality is a few thousand people moderate half of Reddit because it is, in fact, a full-time job that has caused a bunch of concentration in the sort of bureaucratic processes. To do that moderation, even in a system that is designed to be [inaudible 00:36:19]. So I think that's really, really interesting.
Push for the extreme. Why are we even talking about post hoc moderation at all if I'm right about this? Why aren't you ending up in dialogue with the text box you're trying to write in about whether or not what you're saying is constructive? Again, maybe creepy, maybe a bad idea, but a possible idea now, and I think there will be more versions of that.
The systems are also going to create new kinds of abuse. Ultimately, this technology is technology for sorting things into piles regardless of what your piles are and why you want to do that. So it is going to be useful for things like censorship and surveillance; it's going to be useful for things like jawboning. The virtuousness of these tools is simply a product of how they are used; it's not a product of the tools themselves. I think we could easily see the law start to try to specify exactly what your content moderation prompts and standards will be. That is probably a bad idea, but I suspect we will see some folks attempt it at some point as it becomes more and more possible.
All of that said, though, I think this is coming, no matter what, I'm really quite sure that some version of this is going to come to fruition, and so I think we all have an obligation to embrace it and try to figure out how to use it well now so that we're not left on the back foot when it becomes increasingly prevalent. So with all that said, A, hope that was convincing, and B, just want to leave you with an even more provocative question, which is: what would the internet look like if we weren't terrible at content moderation?
The internet has been assumed to be a sort of semi-anarchic space. There's been a lot of discourse about how that has become less true over time, and some, I think about of wistful warnings for a freer version of the internet among at least certain quarters. But, really, we're still pretty bad at content moderation. If I'm right about this, I think it might start to really seriously change the dynamics of how the web even could work in ways that I think are really hard to get our heads around. Maybe bad, maybe good, but worth sort of noodling on in so far as you accept the case. So with that, I'm done.
RELATED READING: Using LLMs for Policy-Driven Content Classification
Authors
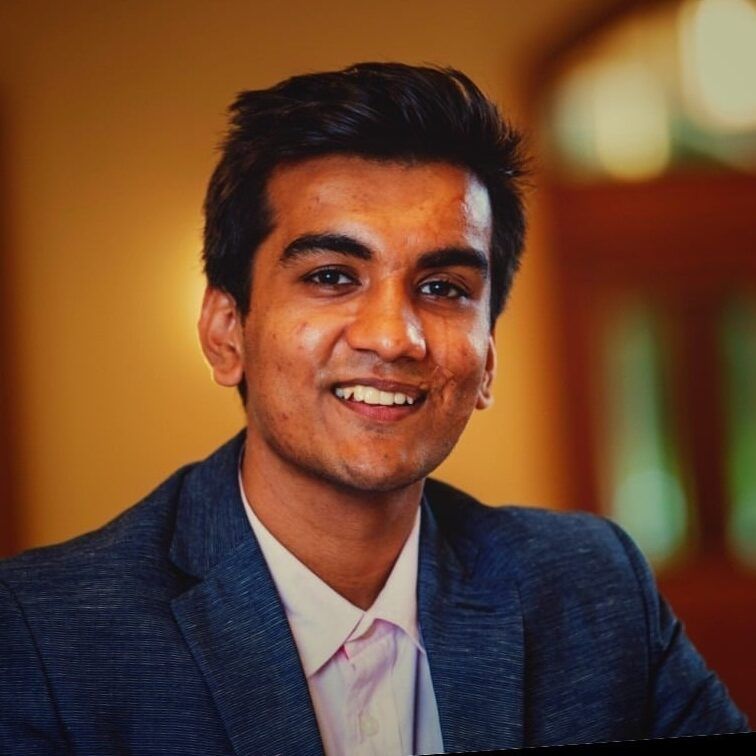