Transparency Won't Be Enough for AI Accountability
Elizabeth (Bit) Meehan / May 17, 2023Elizabeth (Bit) Meehan is a political science PhD candidate at George Washington University.
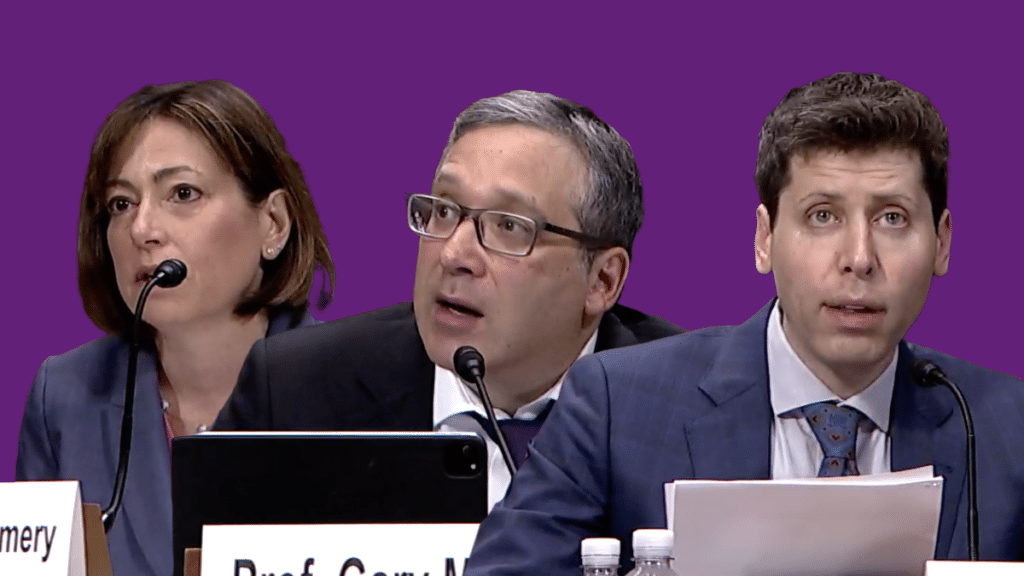
On the surface, the Senate Judiciary Subcommittee Hearing on Oversight of AI went much differently for OpenAI CEO Sam Altman than it did for Meta CEO Mark Zuckerberg in his first Congressional hearing in 2018. Altman received a more conciliatory welcome and meaningful discussion over the harms, biases, and future of AI compared to the tense hearing over the same issues on social media platforms. But like Zuckerberg, Altman used the opportunity to call for more regulation of his industry.
Although the Senators and witnesses suggested a range of regulatory solutions, such as licensing and testing requirements, one regulatory concept appeared to appeal to everyone: transparency. The need for transparency for AI companies and systems was invoked several times throughout the oversight hearing, including from the committee’s Chairman, Sen. Richard Blumenthal (D-CT):
“We can start with transparency. AI companies ought to be required to test their systems, disclose known risks, and allow independent researcher access. We can establish scorecards and nutrition labels to encourage competition based on safety and trustworthiness, limitations on use.”
NYU Professor Emeritus Gary Marcus echoed Sen. Blumenthal:
“Transparency is absolutely critical here to understand the political ramifications, the bias ramifications, and so forth. We need transparency about the data. We need to know more about how the models work. We need to have scientists have access to them.”
Likewise, IBM Chief Privacy & Trust Officer Christina Montgomery defined AI transparency as:
“...disclosure of the data that’s used to train AI, disclosure of the model and how it performs and making sure that there’s continuous governance over these models.”
Many AI researchers and tech advocates, including experts at Mozilla, have also called for greater transparency around AI. But transparency on its own - collecting and disseminating accurate, broad, and complete information about a system and its behaviors - will not be able to curb the harms of AI. It requires an ecosystem of policymakers, firms, civil society groups and the public to work together effectively to hold bad actors accountable and contain harms even from AI developers with the best intentions.
Transparency is often regarded as the solution to a myriad of problems, proposed by policymakers after some kind of crisis like the sea-change unleashed by ChatGPT. And there is plenty of precedent in other sectors, from securities markets and hazardous chemicals to automobile safety and nutritional information (see Sen. Blumenthal’s 'nutrition labels for AI' recommendation), many other issues have inspired transparency mandates. These disclosure laws create a win-win situation for governments. Through light-touch regulation, they can rely on the public engaging in participatory democracy and market forces to sanction bad actors for them.
But by itself, transparency has many flaws. Individuals often don’t know what information they want to have about a problem, and when they are given information, they often don’t have the background knowledge or tools necessary to make sense of it. Disclosers themselves spend enormous amounts of time, money, and resources carefully crafting disclosures that often go unread. Or they provide way too much information in the fine print, making eyes glaze over while obscuring critical details that might change people’s decision making. The link between transparency and accountability is tenuous.
Then there’s breaking down different varieties of transparency, as hinted at by Marcus and Montgomery. Researchers have developed general categories of transparency that also apply to AI including procedures (e.g. the algorithms themselves and the policies to govern them), content (e.g. data inputs and outputs), and outcomes (e.g. AI performance, impact assessments, actual impacts on humans, and policy implementation). The witnesses and Senators at yesterday's hearing called for all three varieties of transparency, all of which require different approaches and resources to achieve. It’s not clear which one is the most important or would create the biggest impact in mitigating the harmful consequences of AI.
Another lingering question is who benefits, and how, from greater transparency. Large firms are often the winners when new regulations are adopted, pushing out smaller competitors that lack the resources to keep up with disclosure requirements and to hire lobbyists to help shape the content of the rules in the first place. It’s not difficult to see why Altman would want to shape the rules now in OpenAI’s favor. Rather than letting the sunshine in, transparency can help to keep potential competitors out.
Despite all of these issues, policymakers don’t have many solutions as likely to find bipartisan support than transparency in their toolkit. It’s a familiar tool that appeals to a wide range of actors on the left and the right. Yet compelling disclosure of information from firms for examination by the public often fails to achieve impact without meaningful implementation and enforcement of transparency laws. Any AI transparency law must focus on rigorous policy implementation and enforcement through a funded government agency conducting third party audits by independent researchers. This agency must also have the power to impose substantive financial sanctions on offending companies and on the individuals who lead these companies.
Any transparency laws must also consider what the public wants to know about AI. Just as humans and algorithms should be studied together, humans and transparency must be studied together. For instance, all of the explainers on how the TikTok algorithm works and qualitative research on what people think influences what they see should guide the development of AI transparency laws. Without asking people what information they want to know and understand, any AI transparency law is unlikely to meet its goal of holding firms accountable.
Authors
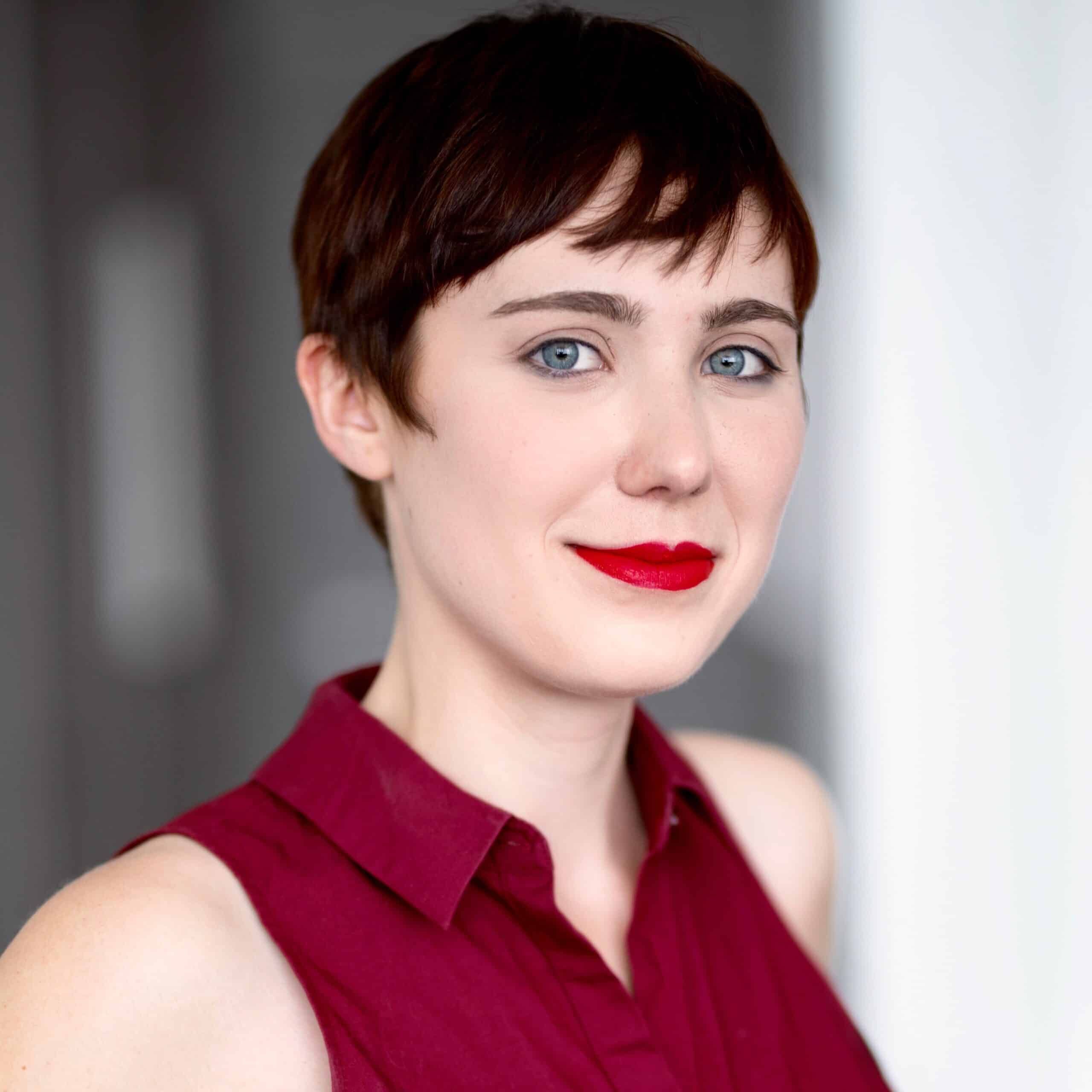