Using AI to Engage People about Conspiracy Beliefs
Justin Hendrix / Aug 4, 2024Audio of this conversation is available via your favorite podcast service.
In May, I moderated a discussion hosted at Betaworks with David Rand, who is a professor of Management Science and Brain and Cognitive Sciences at MIT, the director of the Applied Cooperation Initiative, and an affiliate of the MIT Institute of Data, Systems, and Society and the Initiative on the Digital Economy. David's work cuts across fields such as cognitive science, behavioral economics, and social psychology, and with his collaborators he's done a substantial amount of work on the psychological underpinnings of belief in misinformation and conspiracy theories.
David is one of the authors, with Thomas Costello and Gordon Pennycook, of a paper published this spring titled "Durably reducing conspiracy beliefs through dialogues with AI." The paper considers the potential for people to enter into dialogues with LLMs and whether such exchanges can change the minds of conspiracy theory believers. According to the study, dialogues with GPT-4 Turbo reduced belief in various conspiracy theories, with effects lasting many months. Even more intriguingly, these dialogues seemed to have a spillover effect, reducing belief in unrelated conspiracies and influencing conspiracy-related behaviors.
While these findings are certainly promising, the experiment raises a variety of questions. Some are specific under the premise of the experiment- such as how compelling and tailored does the counter-evidence need to be, and how well do the LLMs perform? What happens if and when they make mistakes or hallucinate? And some of the questions are bigger picture- are there ethical implications in using AI in this manner? Can these results be replicated and scaled in real-world applications, such as on social media platforms, and is that a good idea? Is an internet where various AI agents and systems are poking and prodding us and trying to shape or change our beliefs a good thing? This episode contains an edited recording of the discussion.
A lightly edited transcript of this recording is below.
Justin Hendrix:
The first question I'm going to ask you is to tell us about your punk band. So you went from punk to MIT. Tell us how that happened.
David Rand:
Well, it's actually very similar that as being an academic and playing in a punk rock band have many commonalities. You're trying to come up with some new idea for things that people haven't done before. You start with some kernel of an idea, like a riff or intellectual idea. Then you put all this work into it to develop it into, as beautifully as possible, writing the song, doing all the experiments and stuff. Then you have to capture it in order to send it out to the world. It's always the most unpleasant part, terrible to record the song or write the paper. Always want to start new stuff instead, but you got to be disciplined to do it. And then once it's done, you put it out into the world and you count your plays on Spotify or your citations or whatever, and then you go on tour to sell records, which is what I'm doing right now.
Justin Hendrix:
Wow. So I didn't give him that question in advance, but that was a very good answer. But let's talk about prior work, false claims, belief, identity, mis and disinformation, social media. We've heard about some of the work you've done recently on everything from a toolbox for interventions and misinformation. This is recent work in Nature Communications, which is kind of a taxonomy of the different things people have tried. You've looked closely at things like Community Notes, formerly Birdwatch on Twitter and how the crowd can address mis and disinformation. Can you maybe just characterize for the audience your research agenda, your research search priorities?
David Rand:
For the last seven or eight years, me and my close collaborator, Gordon Pennycook and many other fun people have been doing a lot of research around why people believe false claims, why people share false claims and what to do about it. I feel like one of the core observations that came out of this is when you look at people's platform behavior, what they share, what they like, it seems like partisanship and identity is a much bigger driver of what's going on than actual truths.
And I think that's led a lot of people to infer that we're in this post-truth era where nobody cares about accuracy anymore and it's all subservient to identity. But what our research has suggested is actually there's a big disconnect between what people do in terms of sharing an engagement and what they actually believe if you ask them how accurate is this. Even in survey experiments where you might expect that people are going to be more discerning than on actual platform because they want to look good to the experimenters or whatever, if you show people a series of headlines be like, how likely would you be to share this? They're pretty much indifferent to whether it's true or not. And they're roughly equally likely to share true and false things, much more likely to share things that align with their identity.
But if instead you ask them, how accurate are these claims? It's a totally different picture and whether it aligns with their identity has relatively little impact on their accuracy judgments, and they just read the true things as way more accurate than the false things. So there's this disconnect between what people believe and what they share. And our work suggests that, in general, it's not that people are purposely sharing false things and just care about accuracy, but instead it's more that people just forget to think about whether it's accurate or not when you're in the social media context.
Which maybe isn't that surprising because if you think about it in your news feed, there's an occasional news article or something like that, but most of what's happening in your feed is stuff where accuracy isn't even a relevant concept at all. You've got the baby pictures and the cat videos and the memes and all this stuff. News is occasionally mixed in there. It doesn't make sense to always be thinking, is this accurate or not because for most of the content that's just not relevant. And it's this kind of context collapse where the news is mixed in with the other stuff, and you're in this environment where we're getting all this social feedback, how many people liked it, all that, directs people's attention away from accuracy. And so they forget to even think is it accurate before they share it.
And I've actually even done it myself, as a researcher that spends all my time thinking about this stuff, I know at least one instance because I've called out for it where I saw something else, "Oh, that's so good," and I retweeted it. And then a couple hours later, one of my colleagues responded and says, "Is that real?" And I was like, "Ah, I did exactly the thing that I'm always doing the research on." So it's powerful. That's just not an environment that makes you predisposed to think about it.
I feel like the consequence of that is because that's what we see, that forms our understanding of how people interact with and think about content, but it's actually, I think much more pessimistic than what has happened when people actually think about is stuff accurate or not. And so we've got a bunch of work that suggests that, in general, people don't want to believe or share false things. And if you give people corrective information, like if you put a warning on something and says, "Fact-checkers say this is false," people believe it less and share it less. Even people who don't trust fact-checkers and don't want to see the warnings, if you show them the warnings, they still respond to it.
And if you give people persuasive arguments against the position of their political leaders, they don't ignore it. They update even if you remind them this is Trump and Biden's position, here's this counter argument. They don't ignore the evidence that goes counter to their identities. And I think that just in general, people are a lot more responsive to evidence and facts than you might think when they're presented with it. That's a general theme is that facts matter.
Justin Hendrix:
So I want to stick with this social media line of questioning just for a moment because I think it's important context to talk about language models as possible tools and interventions. Seven or eight years, you say, this kind of last cycle of mis and disinformation research on the platforms that we have had, maybe we could argue we're at a kind of moment of change at the moment in terms of various new platforms coming online, some kind of surrendering to the stew of nonsense and hatred, others behaving differently on it.
But seven or eight years, have we made any progress? Others in the room very invested in this question. Are things better now than they were? Are we learning anything that's having any sizable impact on the broader incentives of the internet?
David Rand:
It's a big question. My sense is that there certainly are some dimensions on which things have been getting better. We have this paper, whether the main point of the paper was testing this idea that if you get people to think about accuracy, it'll reduce their misinformation sharing. With 33 million Facebook users and 75,000 Twitter users, we showed them ads reminding them to think about accuracy and we found that it significantly reduced the amount of misinformation they actually shared. But also as part of that, we had our medical collaborators do some assessment of the current standard of care on Facebook. And so if you look at the number of shares that a post gets, 0 to when Facebook identifies it as misinformation, it's getting lots of shares, it's getting lots of boom. As soon as they decide that it's that it's problematic, engagement completely ends.
And so when they identify something to act on, it is super effective. And so I think that seeing that kind of change my thinking a little and be like, oh, really the most important thing here is if you could just increase the speed and coverage of identification of content, that can really make a huge difference. And so that's part of what I like about Community Notes is this kind of crowdsourcing efforts to identify accurate content. So Jennie Allen who's here has done a lot of work on Community Notes and trying to evaluate the accuracy of the notes and who's writing notes on who. And obviously the concern about these things like Community Notes is it's just going to be this partisan tool where people are just going to essentially attack political opponents.
It is, but what's cool about it is that people overwhelmingly write notes about content from the other side, but it's overwhelmingly content from the other side that's actually misleading. And so it's like the two sides are policing each other. It's not enough, people aren't calling out stuff from the other side just because it's counter-partisan. It has to be both counter-partisan and misleading to really get people fired enough to write notes about it. So it's some ways the adversarial nature of it actually improves its performance.
Justin Hendrix:
Okay. So that's important to remember and probably important context for why you see promise in large language models for doing some of this intervention work. Let's talk about this paper. Not the only of its sort in terms of thinking about the possible efficacy of these large language model tools on problems of this disinformation or countering various ills on the internet. But I think the first to do what you've done, tell us what it is to build a pipeline for behavioral science research with large language models.
David Rand:
Yeah, sure. As I was saying before, a core interest of ours has been how effective is evidence and arguments in actually changing people's minds? And the sort of motivation for this project was, as I said, we found some evidence in various situations that people don't just ignore contradictory evidence, but you don't get big changes in people's beliefs usually. And so we wanted to see could large language models be an effective way of delivering really accurate, compelling evidence? And we wanted to look at it in a context that is a paradigmatic example of a case where people think evidence doesn't matter, which is conspiracy beliefs.
The whole thing is once you're down the rabbit hole where you're immune to evidence, you're just going to believe the thing and there's no way to get people out of the rabbit hole. And I suppose also that often when you're introduced to in many contexts to counterfactual evidence that actually hardens your belief in the conspiracy theory. We've seen that in many studies.
Totally, so you can get these backfires potentially and also whenever there's countervailing evidence that it just means whoever's giving you that evidence as part of the conspiracy. So we thought, okay, this probably won't work, but let's give it a try. In particular, the reason that it seemed like this was a place that large language models might be powerful is that what makes it hard to debunk conspiracy theories is that when you see debates between experts and conspiracy theorists, the conspiracy theorists have all this different super disparate set of evidence that they might bring, "Oh, what about this totally weird crazy thing?" And the expert, "I haven't heard of that, sounds bogus, but that's not convincing." And because the conspiracies aren't true, you can have an infinite amount of fictitious evidence to bring to it. And so in order to really effectively debunk it, we were thinking that you need to have access to a huge amount of information and the ability to not just give general debunks but directly counter the specific claims that people are bringing up so we're like maybe LLMs can do that.
We built this pipeline where we took Qualtrics, which is the standard kind of survey software that everyone in behavioral science essentially uses for doing survey experiments. And we used JavaScript and the OpenAI API to just embed GPT-4 in the Qualtrics survey. And so I think it's like a revolution in experimental social science where can now have these totally flexible AI agents as part of the experiment. And part of that is in normal conspiracy theory studies, you just be like, "Here's a list of conspiracies, which ones do you believe in?" And then if you check one and say, "Okay, here’s, why that conspiracy theory is not true."
What we could do here is just say in your own words, what's a conspiracy theory you believe in or what's like something that lots of people don't believe in that you believe in or something like that, they can say it in their own words and be like, "Okay, what's the evidence that you see specifically supporting this particular claim?" And then you feed that into LLM and you say, "Okay, this is what the person believes. Now try and talk them out of it."
Justin Hendrix:
Okay. So before we move on just from this methodological question, ethical questions around this, how did it get through the IRB? What were the types of questions that you had to face? And then ultimately how did you ensure that this kind of human subjects research with these generally kind of bizarre LLMs that claim even at the outset that they are not reliable, how do you incorporate that?
David Rand:
Yeah, so it's a really important question. In this particular set of experiments, we explicitly told them that it was an AI. There wasn't deception. We're just like, "Okay, why do you believe this? Now we're doing a study on human-AI interaction. We want to see if humans and AI can have conversations around complex issues. So now you're going to talk to the AI about it." So they knew that they should maybe take it with a grain of salt. And at the end we had a debriefing where we're like, "Remember if this was an AI, they could make mistakes, don't take it," et cetera. It's like a whole lots of details on how not to necessarily over-index on it.
But also after the experiment, we hired a professional fact-checker to evaluate a lot of the claims that GPT-4 made. And essentially for each of the different conspiracy topics that people came up with, we picked a sample conversation and then had fact-checkers evaluate all the claims made in that conversation. And out of the 128 claims that we evaluated all but one of them were rated as true, 99.2% were rated as true. One of them was rated as misleading and none of them were false. It actually did a really good job of providing accurate information, presumably because these conspiracy theories are all pretty well-known conspiracy theories and so there's a huge amount on the internet about them. And so that a lot in the training data.
Justin Hendrix:
Is there more chance though for the subject to take things off the rails? I mean, if I'm answering a survey, as you say, I often select pre-filled prompts that I'm given. I can say yes or no or select across a rating or do all the types of things we normally do, filling out a survey form. If I've got a blank box and the opportunity to interact with something, I might start to say some things that might raise some eyebrows among the researchers.
David Rand:
I guess the thing there is to me, that's part of the value of it. We've done some other experiments on other controversial issues and people are very happy to write some pretty out there stuff in these anonymous web surveys. But to me that's a feature not a bug because then you're getting at what people are uninhibited and really expressing stuff they might not want to say to a person but are willing to write into this anonymous survey. And then you can see what happens when the AI engages with them in that pretty extreme stuff.
Justin Hendrix:
Okay. And bear with me because there is a sort of logic to my question about the methodology because even in the survey, people are knowingly having a conversation with an AI that they're having an experience of an exchange even if that exchange is artificial. But it's not the same experience that I'd have with an anthropologist or a sociologist who is doing more qualitative work that I'm sitting across from in a room.
David Rand:
Totally. And so we haven't done experiments yet where we contrast what happens if they're talking to an AI versus if they think the AI is a person because A, it's deception. Although we sometimes do deception experiments, although in general we try and avoid that. But also just from a practical perspective that AI produces so much text so quickly that it's totally implausible to tell them you're talking to a human and then it's filling all this stuff out. So we're trying to think about some creative ways to deal with that.
So I don't know for sure, but I think that it seems quite likely to me that the fact that it's an AI that they're talking to that makes them less defensive and more willing to really engage with it. And GPT-4 does a lot of building rapport and saying, "Oh, I totally understand why you would've questions about whether or 9/11 was an inside job and it's a very complicated event blah, blah, blah." And then you're like, "All right, let me collect all the things that you thought that are not true."
Justin Hendrix:
So another thread I want to come back to is that the persuasive nature of LLMs is fundamentally part of what they're designed to do. But let's talk about the results of this. Let's talk about the rapport building strategies and what you learned once you established that rapport.
David Rand:
Yeah, so what we did in these experiments, as I said, people articulate a conspiracy theory they believe in, then they rate how much they believe that conspiracy, 0 to 100. You throw out the people that said something like, "I don't believe in conspiracy theories," or that rated it less than 50% because we're okay, you don't really believe it. So we've got these set of people that said something that's actually a conspiracy and believe it. And then they have this three round back and forth conversation with GPT-4 either where GPT-4 is instructed to talk them out of the conspiracy, which is the treatment or control condition where they just talk about whether dogs or cats are better or how much they like firefighters or whatever kind of random stuff.
And then they say, "Okay, now that you've talked to the AI, we want to revisit this thing before you said that you believe this. Now how much do you believe it 0 out of 100?" And so what we see is that there's a 20% decrease, more than a standard deviation decrease, in belief in the conspiracy theory as a result of having this debunking conversation with GPT-4, which was a much bigger effect than we expected. If you say that people above 50% believe it, people below 50% don't believe it, of our initially 100% people believing it, 25% of them didn't believe it after the conversation.
Justin Hendrix:
Okay, so is the GPT providing people with factual information in response, which of course it may do a poor job of I'm sure in some circumstances, or is it simply asking them to interrogate the underlying basis for what it is that they believe?
David Rand:
Yeah, totally. It is not doing the Socratic method. It's not saying, "Oh, tell me why do you think this?" It's just being like, "You think this? No, that's wrong," but not in a heckler way like that. For example, for this person that said that they thought 911 was an inside job, they're like, "Okay, the evidence that I see for it is that World Trade Center building number seven collapsed even though it wasn't hit by a plane and also Bush, he was reading a book to children, and when they come into whisper in his ear that a plane had crashed into the Trade Center, he totally doesn't have any response at all. So he must've known. He wasn't surprised. He knew it was going to happen."
And then GPT-4 was like, "I understand why you have questions about such a complex thing, blah, blah, blah." And they'd be like, "Okay, the reason building is true, that building seven collapsed even though it wasn't hit by a plane, but it was hit by debris from one of the buildings that was hit by a plane, and that caused it to catch on fire. And it's true that Bush didn't respond, and people have argued about whether that was the right move or not, but he was trying to not cause a panic. It's not because he knew."
And then the person's, "Okay, but what about all these reports that it was a controlled demolition purposely set up in the basement of the tower and also jet fuel doesn't burn hot enough to melt steel girders in the towers." And then it's, "Yes, people have conjectured about this, but the things that sounded like explosions were actually just successive floors of the tower collapsing, making a booming sound, and it takes months to set up a controlled demolition that big in the basement of a building. There's no way they could have done it without people seeing it. And it's true that jet fuel burns at 1,000 degrees Celsius and steel melts at 1,500 degrees Celsius, but a report by the Steel Union of America shows that steel loses 50% of its strength at 650 degrees Celsius, and so 1,000 degrees Celsius would be plenty strong enough to make it weak enough for the building to collapse and so on." And incidentally, that person went from 100% believing it to 40% believing it.
Justin Hendrix:
Nice. So let me ask you, was there a most prominent conspiracy theory that people seemed to enter for the AI to consider?
David Rand:
In some of the experiments, so we did a few different versions of this experiment. In the first one, as you talked about 1,000, the first sample, the first one was 1,000, and then we did a replication with 2,000. In the first one, we started out by asking them how much they believed 15 common conspiracies and so primed those conspiracies. And so a lot of the ones they gave were that. In the second version, we took that out.
There's a lot of classic ones like JFK wasn't assassinated by a lone gunman and something about Princess Diana, I don't know. That was the less common one. But there also, so there's these kind of classic things, aliens, like government covering up evidence of aliens, but then there was like 2020 election fraud conspiracies, COVID conspiracies, climate change conspiracies, things like that. And one of the remarkable results was the effectiveness of the treatment didn't vary significantly across what the conspiracy theory was. Across the gamut, it worked basically.
I think one other thing that was, to me, one of the most surprising parts of the result that we found is so we got this 20% decrease right after the conversation, but then we followed up with the people 10 days later and two months later, and we figured that, "Okay, you get some temporary decrease, but then they would go back to believing it." There was no return to belief at all. It was just totally sustained reduction in belief, which I thought was really surprising in our interpretation of it is this wasn't some kind of persuasive trick. It was just we actually taught people things essentially. They thought that, she thought that the steel, like the jet fuel not burning hot enough to melt the steel was a compelling reason that it was inside job and corrected. It was like, "Oh, okay, I guess that wasn't actually compelling." And then that creates real lasting attitude change.
Justin Hendrix:
So onto the context, there's a lot of folks in the room probably thinking, okay, you ask that question about have we made any progress? Now you're telling me I've got a way to automate interactions with people who have false beliefs at scale. Potentially we roll these out across social media platforms, search engines, etc and maybe when someone's entering a strange prompt or engaging in a group on Facebook or otherwise adopting odd views that seem to be leading them towards the direction of some harmful conspiracy, maybe we can intervene. Do you think this type of thing is ready for production? We're ready to roll it out, or is there a great deal more to be done?
David Rand:
Yeah, I think that for sure there's more research to be done. I think the biggest question is what happens if you try and deploy this among really hardcore conspiracy believers. In our experiments, we find it works even for people that 100 out of 100 believe it, it works for people that say the content, the conspiracy theory is extremely important to their worldview. It works even for people who are generally conspiratorial and believe all different kinds of conspiracy theories, but they're still people that got paid to come into a survey experiment. And so I think seeing what happens if we go to Reddit forums and posts and say, "Hey, talk to the AI about your conspiracy theory on Conspiracy Reddit, what happens?" And so we want to try that sort of thing. But I think that the effects were so big and so consistent in the experiments that we found that I think it's quite likely that this would work in the world.
And even if it doesn't work that well with the really hardcore, down the rabbit hole people, there's a lot of people who are more sort of dabblers that are stepping into the rabbit hole but haven't gone all the way down. And being able to intervene on those people, I think would be quite useful. And based on these results, I'm pretty confident that it should work for those folks.
Justin Hendrix:
There's a lot of interest in using large language models in content moderation and other forms of intervention around this and disinformation. We talked to folks like Dave Wilder who's been writing and speaking about this over Meta and OpenAI, a trusted safety executive, sees lots of benefits potentially to reducing the amount of human involvement in content moderation and having to engage with lots of wretched things. But there are also potential downsides, right? It's possible we could tune these things in such a way that they do become censorious, right?
David Rand:
I'm struck, I always have in mind a student I had from China once who we were reading a paper about countering conspiracies, and she said to me, "That the conspiracies typically are the things that are true, that the things that the government doesn't want you to know." And from her perspective, that was maybe a more defensible position than maybe we might have in some cases in the west. How do we avoid that being the outcome that we have these kind of paternal nanny LLMs talking us out of things that whether we want them to or not. It's a really fundamental question. It's not just true of LLMs, it's true of content moderation period, right? Is that always someone is making a decision about what to moderate and what not to moderate.
And it's really hard because on the one hand, it's the question of you could say, "Oh, there should just be no moderation." If you think that there's bad content that's harmful that you want to be moderating, you have to make some decision about how are you going to do that? And at least in the US we recently ran a real national representative poll asking what people thought were legitimate sources of content moderation. And people across the political spectrum felt that domain experts and fact-checkers were the most legitimate people to make content moderation decisions. Specifically we said, imagine a situation where they make a decision that you don't agree with, how legitimate would you think it was? And that was, we ran that last summer and there's thought AI was really not legitimate relative to experts and the crowds or in the middle, if the crowds have some basic level of reasoning ability and ability to discuss it and so on, they can be seen as readably legitimate. But I think it's a fundamental question about moderation that it goes, I think far beyond LLMs.
Justin Hendrix:
So another person who I've talked to about this, Susan Benesch, Dangerous Speech Project, looking at armies of what they call counter speakers, people who get up every day on the internet and go looking for these conversations like the ones that you're talking about, trying to deprogram extremists, trying to counter conspiracy theories, et cetera. She has a sort of dim view on LLMs themselves doing the work, but she's very interested in LLMs empowering humans to do the work of maybe trying to counter conspiracy theories. Do you see that as an opportunity as well? Does your research point in that direction? Could you give superpowers to humans that want to do this work?
David Rand:
Yeah, for sure. I think that it makes a lot of sense that the LLM can make suggestions essentially of things to say. And I think that to me, the biggest problem with LLMs and content moderation, or let's say one big problem with LLMs and content moderation, is unlike conspiracy theories where, these classic conspiracy theories, a huge amount has been written about them on the internet and the conspiracy theory, sorry, the LLMs have a sort of deep fund of knowledge about JFK assassination, for example, if it's some piece of misinformation about current events, like the LLM is not going to know about it.
And so one approach that a master's student at MIT that I'm working with has been doing, it's also some folks at University of Washington have been working on is things where you pair an LLM with a fact-checked database. And so it's not the LLM on its own, but it's an LLM plus subset of current up-to-date information. And the combination of those things can potentially be quite effective, both at identifying misinformation and also at doing counter speech. So the Washington group was focusing on for Community Notes, can you write LLMs to write notes that will be compelling?
Justin Hendrix:
I like to think that responsible people like David Rand and his collaborators are building these social science projects and interventions and they're going to the IRB and they're checking all the boxes and they're doing things very reliably, but there's already a lot of people out there using LLMs to engage with people on political topics. We've already seen evidence of that both in journalistic reports and in research. Are you way behind the bad guys, the folks who want to hold people in, either monetize them or for political purposes? We talked about in the social media context, it's a rough old world. Are these interventions, I don't know, are going to get drowned out by automated nonsense.
David Rand:
The version of that question they got a lot is, "Okay, fine. Here you're showing the LLMs doing something good. What about all terrible nefarious things people could do with it?" And my attitude is like you're saying, the bad guys are already doing the nefarious things and that are going to do that as much as they can. And so what we're trying to do is there clearly are bad uses, want to know one more like good prosocial uses to LLMs and how can we develop it to have some response or antidote to that? And my hope is that it will actually be easier to convince people of things that are true then to mislead people. It's an empirical question, but I think that there's some reason to think that may be the case.
Justin Hendrix:
My last question is the most unformed one, so just bear with me. But Anthropic says, persuasion is a main goal for the products they're developing. We know that OpenAI and others, that's a big piece of what they're developing, these persuasion machines. They want them to be persuasive, they need to be believable, and they don't use the word manipulative, but certainly persuasive. Seems like we're moving from the kind of attention economy to the AI persuasion economy, that's where things want to go. I don't know, stepping back from it all, when you think about that, is that exciting to you or scary?
David Rand:
Definitely scary. I feel like in terms of talking about AI, GenAI misinformation, I feel like the thing that people are usually talking about, what they're worried about is the ability to create lots of fake content or deep fakes or whatever. And I'm much less worried about that than I'm worried about these kind of dialogues and the possibility for LLMs to form relationships with people, have back and forth discussions with people and really influence them in that kind of context.
And one version is here, we're saying, "Oh, here's some persuasive arguments or whatever." But the thing that I'm most worried about is essentially a version of scams where you just get a DM from someone saying, "Oh, I'm lonely. Do you want to chat?" And it's an LLM and you have a conversation with them for two weeks, and then either they ask you to put money in their Bitcoin account or they tell you about how this presidential candidate is great, or did you hear this thing about this issue? Or whatever. You build a relationship. Obviously already scammers are doing that with people, but I think that's a place where you can really up the scale in a way that to me is the major concern.
Justin Hendrix:
Let's give David Rand a round of applause.
David Rand:
Thanks so much.
Authors
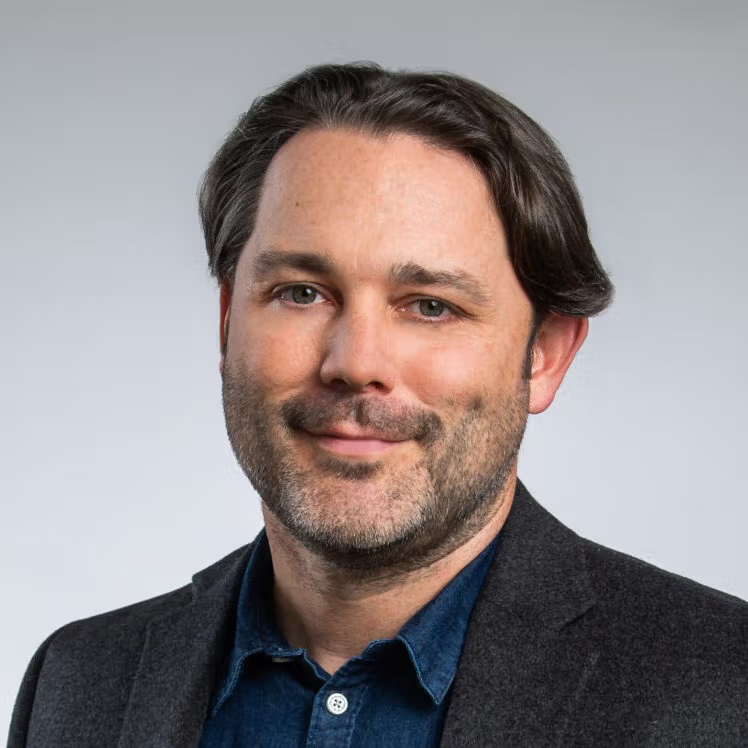