Without Accounting for Bias and Discrimination, AI Safety Standards Will Fall Short
Serena Oduro / Sep 16, 2024Serena Oduro is a Senior Policy Analyst at Data & Society.
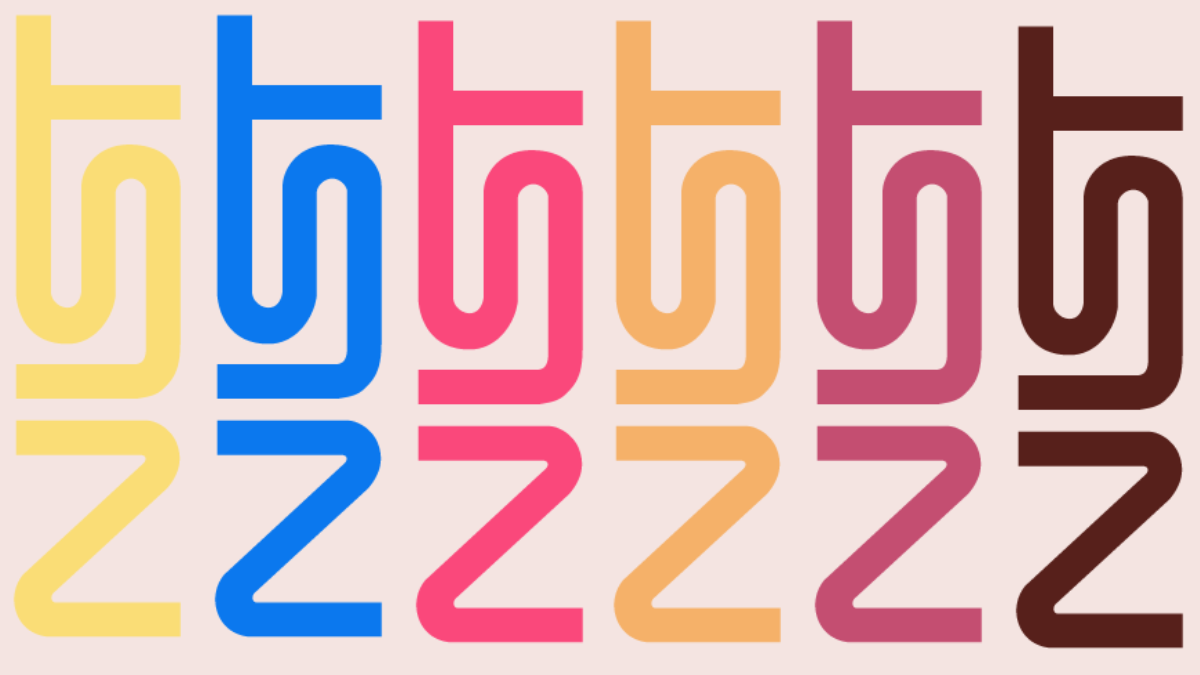
Composite of NIST logos.
It was a brat summer and an Olympic summer — but less known to many, also a summer in which the foundation of much-needed AI safety standards that will guide AI governance began to come into focus. As the public grapples with the many ways AI models are being integrated into our lives, it is increasingly clear that standard development bodies will need to act with purpose to ensure that these standards address bias and discrimination, and other AI-related harms that gravely impact the public today.
In July, the National Industry of Standards and Technology (NIST), the leading AI standards development body in the US, released an initial public draft on Managing Misuse Risk for Dual-Use Foundation Models. While the draft addressed national security issues, NIST explicitly noted that it did not address issues of bias and discrimination. This absence was a missed opportunity to address the misuse risks that impact society and should greatly concern the public. We have seen how models can be used to further violence towards minority groups, and, as Russia’s use of social media algorithms to flame social divisions in the 2016 US elections exemplifies, even be used by state actors to sow division. While the initial draft does address child sexual abuse material (CSAM) and non-consensual intimate imagery (NCII), its inclusion only highlights the importance of addressing bias and discrimination: issues of CSAM and NCII are rooted in gender-based violence, and cannot be effectively addressed or understood without that broader context. Bias and discrimination are a foundational influence on our society that can be wielded by state and independent actors to threaten our safety. Any attempt to regulate and create standards across AI governance efforts must treat bias and discrimination as a cross-cutting central issue and address them as such. Simply put, they do not belong in a separate bucket.
To fully address the grave threats that algorithmic bias and discrimination pose to historically marginalized communities and our governmental and social structures, our AI policies, standards, and standards-making processes must be guided by advocacy and research that highlight types and uses of AI systems that are discriminatory, and that implement and advance the methods, practices, and policies needed to protect the public. AI standards should make it a norm for historically marginalized communities to be consulted, and their requests prioritized, throughout AI development and risk assessment processes. It should also be a norm that a wide swathe of experts – including data scientists, machine learning engineers, humanities experts, social scientists, and user experience researchers and designers – inform and are engaged in AI development and risk management, so that the many pernicious ways that bias and discrimination manifest are addressed and remediation processes are established accordingly.
NIST’s July release of its Artificial Intelligence Risk Management Framework: Generative Artificial Intelligence Profile offered a glimpse of what it looks like for AI standards to grapple with bias and discrimination. The profile is a 60+ page document that takes the four functions from NIST’s AI risk management profile (Govern, Map, Measure, and Manage — think of these as the stages of the AI development and management process), and provides actions across them to establish practices for managing generative AI risks.
The profile identifies “harmful bias and homogenization” as one of the twelve risks generative AI poses and provides actions to address these risks, such as implementing continuous monitoring of generative AI systems for impacts on sub-populations. Yet the specificity of the recommended actions in the draft published for public comment earlier this year to address harmful bias (including those to ensure multidisciplinary expertise in risk assessment, participatory methods, and evaluation methods for discrimination) were reduced in the final version. While it is no surprise that the hefty initial draft needed to be streamlined, there is no excuse for holding back on the actions and methods needed to ensure that the public is safe from AI harm.
If guidelines laying out the standards for AI risk management are not where in-depth guidance on bias and discrimination will be provided, it is critical for other empowered cross-disciplinary sites to do so. NIST’s US AI Safety Institute and Assessing Risks and Impacts of AI (ARIA) program are two potential sites to create in-depth standards to assess and prevent bias and discrimination. Yet the tech industry's dominance, the treatment of bias and discrimination as a distinct issue area, and an over-focus on model testing and technical evaluations could thwart that potential – and not just at NIST or in the US. As AI standards are created across the world (including while the Codes of Practice on General Purpose AI under the EU AI Act are developed), it is imperative to make addressing bias and discrimination a central focus. This means centering voices, objectives, expertise, and methods that are rich with guidance, but often ignored in the AI sphere — including demands from historically marginalized communities, participatory methods, ethnographic methods, and other sociotechnical forms of evaluation.
It will be an uphill battle to ensure that AI standards adequately and robustly address bias and discrimination to protect the public. But these concerns cannot be sidelined. Refusing to prioritize addressing bias and discrimination creates a monstrous impediment to building the standards and practices needed to address AI harms and build AI systems that propel us all forward. As this AI ‘standards summer’ cools into the fall, it is vital that we take stock of what has been built thus far and recommit to ensure that the standards being built actually protect the public.
Authors
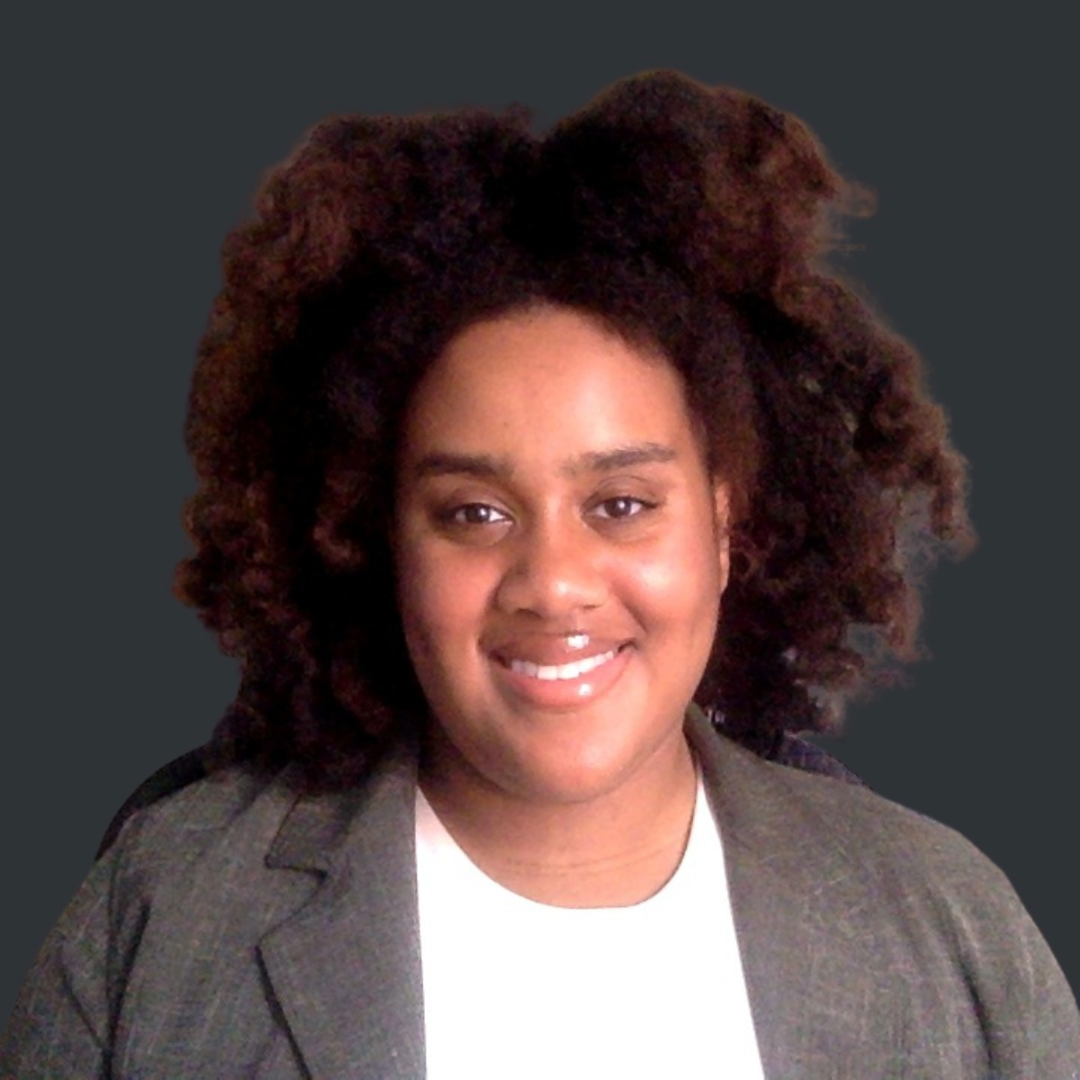