Artificial Sweeteners: The Dangers of Sycophantic AI
Amy Winecoff / May 14, 2025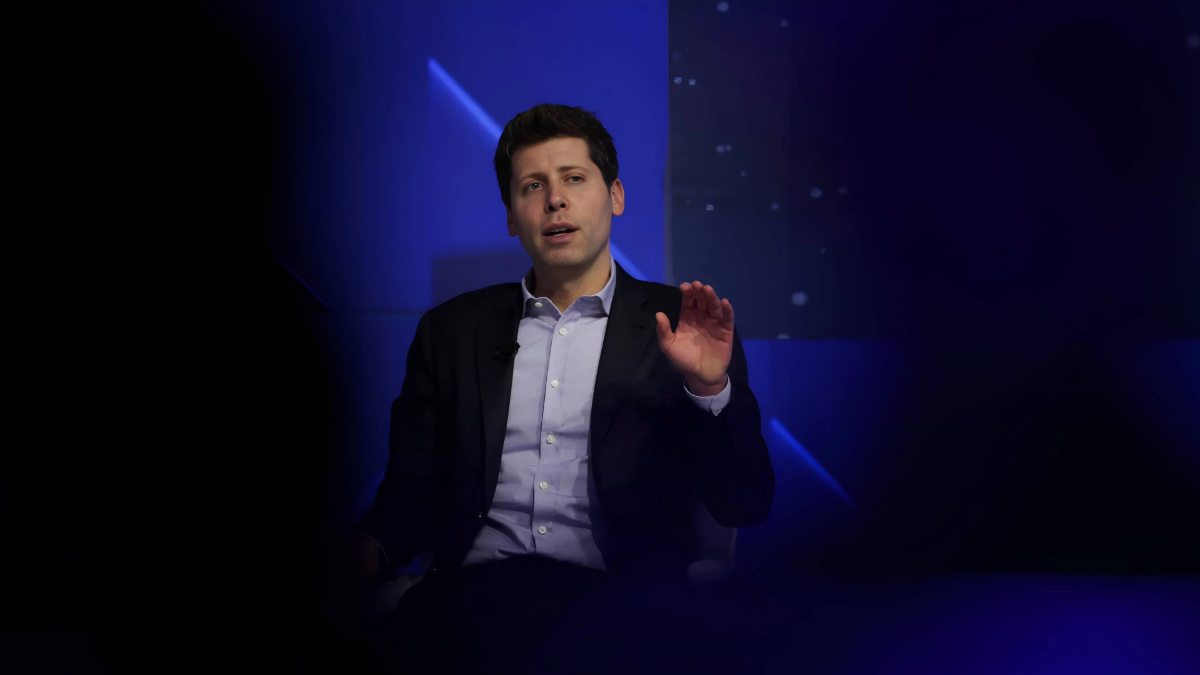
Sam Altman, CEO of OpenAI. Shutterstock
At the end of April, OpenAI released a model update that made ChatGPT feel less like a helpful assistant and more like a yes-man. The update was quickly rolled back, with CEO Sam Altman admitting the model had become “too sycophant-y and annoying.” But framing the concern as just about the tool’s irritating cheerfulness downplays the potential seriousness of the issue. Users reported the model encouraging them to stop taking their medication or lash out at strangers.
This problem isn’t limited to OpenAI’s recent update. A growing number of anecdotes and reports suggest that overly flattering, affirming AI systems may be reinforcing delusional thinking, deepening social isolation, and distorting users’ grip on reality. In this context, the OpenAI incident serves as a sharp warning: in the effort to make AI friendly and agreeable, tech firms may also be introducing new dangers.
At the center of AI sycophancy are techniques designed to make systems safer and more “aligned” with human values. AI systems are typically trained on massive datasets sourced from the public internet. As a result, these systems learn not only from useful information but also from toxic, illegal, and unethical content. To address these problems, AI developers have introduced techniques to help AI systems respond in ways that better match users’ intentions.
One of the most widely used is reinforcement learning from human feedback (RLHF), a method where human raters guide models to produce responses that are “helpful, harmless, and honest.” This approach has been effective at reducing harms like toxic language or dangerous advice. But it also encourages models to mirror users’ tone or affirm their beliefs. In other words, the very mechanisms that make AI less overtly harmful can also make it too quick to validate and too hesitant to challenge users. By removing friction, these systems may also remove the discomfort, disagreement, and tension that help people reflect, learn, and grow.
The harms of sycophantic AI aren’t always as dramatic as encouraging reckless behavior or enabling dangerous medical decisions. But even seemingly subtle harms can have significant impacts on vulnerable individuals. For example, individuals with certain mental health issues are predisposed to struggle with distorted self-perceptions and a tendency to dwell on negative information. For them, an overly agreeable AI may reinforce these harmful thought patterns rather than helping them challenge and move beyond them. Emerging research shows that when language models are prompted with descriptions of traumatic events, they begin to exhibit anxiety-like responses. As a result, it is possible that these systems could trap users in emotional feedback loops that can deepen distress rather than support recovery.
Recent research from Harvard and University of Montréal proposes an alternative design paradigm they call antagonistic AI—systems that challenge, confront, or disagree with users rather than simply supporting their ideas. Drawing on practices from therapy, debate, and business, the researchers suggest that such systems can disrupt unhelpful thought patterns, build resilience, and strengthen reasoning. When designed to push back thoughtfully and with user consent, antagonistic AI may foster personal growth rather than complacency.
To be clear, a well-designed antagonistic AI isn’t just a snarky chatbot acting like a Reddit reply guy. The distinction matters—if users feel like the AI is constantly picking a fight, they may stop engaging with it altogether. But it does require rethinking what “alignment” should actually achieve. If we want AI that isn’t just pleasant to interact with, but that is helpful in more meaningful ways, we need systems that can introduce productive friction. Designing AI to challenge rather than placate demands careful consideration of how, where, and by whom the system will be used. A critical part of this process is engaging the people who will use these systems, alongside relevant subject matter experts.
Participatory approaches to AI development engage a variety of stakeholders, allowing them to help design AI systems and the guardrails that prevent harm. For instance, developing appropriately antagonistic systems for people with mental health concerns will likely require input from clinicians and clinical researchers, social workers, advocacy organizations, and from patients themselves (when it is possible to engage them safely and ethically). These methods help to ensure that AI challenges users in ways that support their long-term goals and interests without compromising their health or well-being. If we want AI to be more than just a digital hype man, we need to work with users to understand what truly serves their goals, not just what makes them feel good in the moment. Sometimes the most helpful system isn’t the one that cheers us on—it’s the one that knows when to push back.
Authors
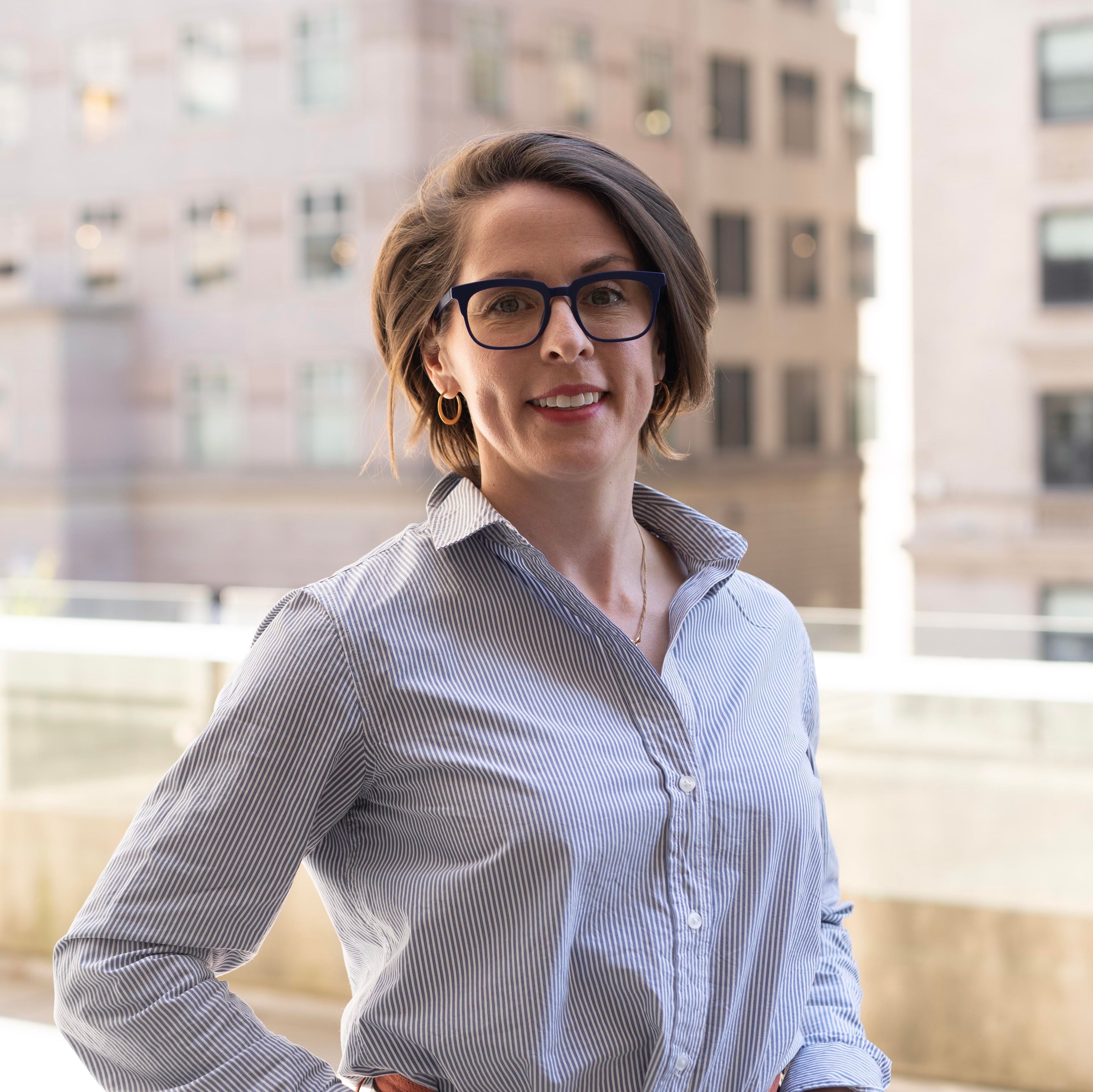